Snapshot of - MESSAGE-GLOBIOM
Archive of MESSAGE-GLOBIOM, version: 1.0
Reference card - MESSAGE-GLOBIOM
The reference card is a clearly defined description of model features. The numerous options have been organized into a limited amount of default and model specific (non default) options. In addition some features are described by a short clarifying text.
Legend:
- not implemented
- implemented
- implemented (not default option)
About
Name and version
MESSAGE-GLOBIOM 1.0
Model link
Institution
International Institute for Applied Systems Analysis (IIASA), Austria, http://data.ene.iiasa.ac.at.
Documentation
MESSAGE-GLOBIOM documentation consists of a referencecard and detailed model documentation
Process state
published
Model scope and methods
Model documentation: Model scope and methods - MESSAGE-GLOBIOM
Model type
- Integrated assessment model
- Energy system model
- CGE
- CBA-integrated assessment model
Geographical scope
- Global
- Regional
Objective
MESSAGE at its core is a technology-detailed energy-engineering optimization model used for energy planning. Through linkage to macro-economic, land-use and climate models it is capable of taking into account important feedbacks and limitations in these areas outside of the energy system.
Solution concept
- Partial equilibrium (price elastic demand)
- Partial equilibrium (fixed demand)
- General equilibrium (closed economy)
Solution horizon
- Recursive dynamic (myopic)
- Intertemporal optimization (foresight)
Solution method
- Simulation
- Optimization
Temporal dimension
Base year:2030, time steps:10, horizon: 2110
Spatial dimension
Number of regions:11
- AFR (Sub-Saharan Africa)
- CPA (Centrally Planned Asia & China)
- EEU (Eastern Europe)
- FSU (Former Soviet Union)
- LAM (Latin America and the Carribean)
- MEA (Middle East and North Africa)
- NAM (North America)
- PAO (Pacific OECD)
- PAS (Other Pacific Asia)
- SAS (South Asia)
- WEU (Western Europe)
Time discounting type
- Discount rate exogenous
- Discount rate endogenous
Policies
- Emission tax
- Emission pricing
- Cap and trade
- Fuel taxes
- Fuel subsidies
- Feed-in-tariff
- Portfolio standard
- Capacity targets
- Emission standards
- Energy efficiency standards
- Agricultural producer subsidies
- Agricultural consumer subsidies
- Land protection
- Pricing carbon stocks
Socio-economic drivers
Model documentation: Socio-economic drivers - MESSAGE-GLOBIOM
Population
- Yes (exogenous)
- Yes (endogenous)
Population age structure
- Yes (exogenous)
- Yes (endogenous)
Education level
- Yes (exogenous)
- Yes (endogenous)
Urbanization rate
- Yes (exogenous)
- Yes (endogenous)
GDP
- Yes (exogenous)
- Yes (endogenous)
Income distribution
- Yes (exogenous)
- Yes (endogenous)
Employment rate
- Yes (exogenous)
- Yes (endogenous)
Labor productivity
- Yes (exogenous)
- Yes (endogenous)
Total factor productivity
- Yes (exogenous)
- Yes (endogenous)
Autonomous energy efficiency improvements
- Yes (exogenous)
- Yes (endogenous)
Other socio economic driver
- Behavioural change
Macro-economy
Model documentation: Macro-economy - MESSAGE-GLOBIOM
Economic sector
Industry
- Yes (physical)
- Yes (economic)
- Yes (physical & economic)
Energy
- Yes (physical)
- Yes (economic)
- Yes (physical & economic)
Transportation
- Yes (physical)
- Yes (economic)
- Yes (physical & economic)
Residential and commercial
- Yes (physical)
- Yes (economic)
- Yes (physical & economic)
Agriculture
- Yes (physical)
- Yes (economic)
- Yes (physical & economic)
Forestry
- Yes (physical)
- Yes (economic)
- Yes (physical & economic)
Other economic sector
- Services (physical)
Macro-economy
Trade
- Coal
- Oil
- Gas
- Uranium
- Electricity
- Bioenergy crops
- Food crops
- Capital
- Emissions permits
- Non-energy goods
Cost measures
- GDP loss
- Welfare loss
- Consumption loss
- Area under MAC
- Energy system cost mark-up
Categorization by group
- Income
- Urban - rural
- Technology adoption
- Age
- Gender
- Education level
- Household size
Institutional and political factors
- Early retirement of capital allowed
- Interest rates differentiated by country/region
- Regional risk factors included
- Technology costs differentiated by country/region
- Technological change differentiated by country/region
- Behavioural change differentiated by country/region
- Constraints on cross country financial transfers
Resource use
Coal
- Yes (fixed)
- Yes (supply curve)
- Yes (process model)
Conventional Oil
- Yes (fixed)
- Yes (supply curve)
- Yes (process model)
Unconventional Oil
- Yes (fixed)
- Yes (supply curve)
- Yes (process model)
Conventional Gas
- Yes (fixed)
- Yes (supply curve)
- Yes (process model)
Unconventional Gas
- Yes (fixed)
- Yes (supply curve)
- Yes (process model)
Uranium
- Yes (fixed)
- Yes (supply curve)
- Yes (process model)
Bioenergy
- Yes (fixed)
- Yes (supply curve)
- Yes (process model)
Water
- Yes (fixed)
- Yes (supply curve)
- Yes (process model)
Raw Materials
- Yes (fixed)
- Yes (supply curve)
- Yes (process model)
Land
- Yes (fixed)
- Yes (supply curve)
- Yes (process model)
Technological change
Energy conversion technologies
- No technological change
- Exogenous technological change
- Endogenous technological change
Energy End-use
- No technological change
- Exogenous technological change
- Endogenous technological change
Material Use
- No technological change
- Exogenous technological change
- Endogenous technological change
Agriculture (tc)
- No technological change
- Exogenous technological change
- Endogenous technological change
Other technological change
- Energy Conversion - Exogenous Technological Change
Energy
Model documentation: Energy - MESSAGE-GLOBIOM
Energy technology substitution
Energy technology choice
- No discrete technology choices
- Logit choice model
- Production function
- Linear choice (lowest cost)
- Lowest cost with adjustment penalties
Energy technology substitutability
- Mostly high substitutability
- Mostly low substitutability
- Mixed high and low substitutability
Energy technology deployment
- Expansion and decline constraints
- System integration constraints
Energy
Electricity technologies
- Coal w/o CCS
- Coal w/ CCS
- Gas w/o CCS
- Gas w/ CCS
- Oil w/o CCS
- Oil w/ CCS
- Bioenergy w/o CCS
- Bioenergy w/ CCS
- Geothermal power
- Nuclear power
- Solar power
- Solar power-central PV
- Solar power-distributed PV
- Solar power-CSP
- Wind power
- Wind power-onshore
- Wind power-offshore
- Hydroelectric power
- Ocean power
Hydrogen production
- Coal to hydrogen w/o CCS
- Coal to hydrogen w/ CCS
- Natural gas to hydrogen w/o CCS
- Natural gas to hydrogen w/ CCS
- Oil to hydrogen w/o CCS
- Oil to hydrogen w/ CCS
- Biomass to hydrogen w/o CCS
- Biomass to hydrogen w/ CCS
- Nuclear thermochemical hydrogen
- Solar thermochemical hydrogen
- Electrolysis
Refined liquids
- Coal to liquids w/o CCS
- Coal to liquids w/ CCS
- Gas to liquids w/o CCS
- Gas to liquids w/ CCS
- Bioliquids w/o CCS
- Bioliquids w/ CCS
- Oil refining
Refined gases
- Coal to gas w/o CCS
- Coal to gas w/ CCS
- Oil to gas w/o CCS
- Oil to gas w/ CCS
- Biomass to gas w/o CCS
- Biomass to gas w/ CCS
Heat generation
- Coal heat
- Natural gas heat
- Oil heat
- Biomass heat
- Geothermal heat
- Solarthermal heat
- CHP (coupled heat and power)
Grid Infra Structure
Electricity
- Yes (aggregate)
- Yes (spatially explicit)
Gas
- Yes (aggregate)
- Yes (spatially explicit)
Heat
- Yes (aggregate)
- Yes (spatially explicit)
CO2
- Yes (aggregate)
- Yes (spatially explicit)
Hydrogen
- Yes (aggregate)
- Yes (spatially explicit)
Energy end-use technologies
Passenger transportation
- Passenger trains
- Buses
- Light Duty Vehicles (LDVs)
- Electric LDVs
- Hydrogen LDVs
- Hybrid LDVs
- Gasoline LDVs
- Diesel LDVs
- Passenger aircrafts
Freight transportation
- Freight trains
- Heavy duty vehicles
- Freight aircrafts
- Freight ships
Industry
- Steel production
- Aluminium production
- Cement production
- Petrochemical production
- Paper production
- Plastics production
- Pulp production
Residential and commercial
- Space heating
- Space cooling
- Cooking
- Refrigeration
- Washing
- Lighting
Land-use
Model documentation: Land-use - MESSAGE-GLOBIOM
Land cover
- Cropland
- Cropland irrigated
- Cropland food crops
- Cropland feed crops
- Cropland energy crops
- Forest
- Managed forest
- Natural forest
- Pasture
- Shrubland
- Built-up area
Agriculture and forestry demands
- Agriculture food
- Agriculture food crops
- Agriculture food livestock
- Agriculture feed
- Agriculture feed crops
- Agriculture feed livestock
- Agriculture non-food
- Agriculture non-food crops
- Agriculture non-food livestock
- Agriculture bioenergy
- Agriculture residues
- Forest industrial roundwood
- Forest fuelwood
- Forest residues
Agricultural commodities
- Wheat
- Rice
- Other coarse grains
- Oilseeds
- Sugar crops
- Ruminant meat
- Non-ruminant meat and eggs
- Dairy products
Emission, climate and impacts
Model documentation: Emissions - MESSAGE-GLOBIOM, Climate - MESSAGE-GLOBIOM, Non-climate sustainability dimension - MESSAGE-GLOBIOM
Greenhouse gases
- CO2 fossil fuels
- CO2 cement
- CO2 land use
- CH4 energy
- CH4 land use
- CH4 other
- N2O energy
- N2O land use
- N2O other
- CFCs
- HFCs
- SF6
- PFCs
Pollutants
- CO energy
- CO land use
- CO other
- NOx energy
- NOx land use
- NOx other
- VOC energy
- VOC land use
- VOC other
- SO2 energy
- SO2 land use
- SO2 other
- BC energy
- BC land use
- BC other
- OC energy
- OC land use
- OC other
- NH3 energy
- NH3 land use
- NH3 other
Climate indicators
- Concentration: CO2
- Concentration: CH4
- Concentration: N2O
- Concentration: Kyoto gases
- Radiative forcing: CO2
- Radiative forcing: CH4
- Radiative forcing: N2O
- Radiative forcing: F-gases
- Radiative forcing: Kyoto gases
- Radiative forcing: aerosols
- Radiative forcing: land albedo
- Radiative forcing: AN3A
- Radiative forcing: total
- Temperature change
- Sea level rise
- Ocean acidification
Carbon dioxide removal
- Bioenergy with CCS
- Reforestation
- Afforestation
- Soil carbon enhancement
- Direct air capture
- Enhanced weathering
Climate change impacts
- Agriculture
- Energy supply
- Energy demand
- Economic output
- Built capital
- Inequality
Co-Linkages
- Energy security: Fossil fuel imports & exports (region)
- Energy access: Household energy consumption
- Air pollution & health: Source-based aerosol emissions
- Air pollution & health: Health impacts of air Pollution
- Food access
- Water availability
- Biodiversity
Model Documentation - MESSAGE-GLOBIOM
The IIASA IAM framework consists of a combination of five different models or modules - the energy model MESSAGEix, the land use model GLOBIOM, the air pollution and GHG model GAINS, the aggregated macro-economic model MACRO, and the simple climate model MAGICC - which complement each other and are specialized in different areas. All models and modules together build the IIASA IAM framework, also referred to as MESSAGEix-GLOBIOM because the energy model MESSAGEix and the land use model GLOBIOM are its most important components. The five models provide input to and iterate between each other during a typical SSP scenario development cycle.
1) Model scope and methods - MESSAGE-GLOBIOM
MESSAGEix[1] represents the core of the IIASA IAM framework and its main task is to optimize the energy system so that it can satisfy specified energy demand at the lowest cost. MESSAGEix carries out this optimization in an iterative setup with MACRO, which provides estimates of the macro-economic demand response that results from energy system and services costs computed by MESSAGEix. For the six commercial end-use demand categories depicted in MESSAGEix (see Demand section of MESSAGEix-GLOBIOM), MACRO adjusts useful energy demand based on demand prices until the two models have reached equilibrium (see Macro-economy section of MESSAGEix-GLOBIOM). It thus reflects price-induced energy efficiency improvements that can occur when energy prices change. MESSAGEix can represent different energy- and climate-related policies (see Policy section of MESSAGEix-GLOBIOM).
GLOBIOM provides MESSAGEix with information on land use and its implications, like the availability and cost of bio-energy, and the availability and cost of emission mitigation in the AFOLU (Agriculture, Forestry and Land Use) sector (see Land-use of MESSAGEix-GLOBIOM). To reduce computational costs, MESSAGEix iteratively queries a GLOBIOM emulator which provides an approximation of land-use outcomes during the optimization process instead of requiring the GLOBIOM model to be rerun iteratively. Once the iteration between MESSAGEix and MACRO has converged, the resulting bioenergy demands along with corresponding carbon prices are used for a concluding analysis with the full-fledged GLOBIOM model. This ensures full consistency in the results from MESSAGEix and GLOBIOM, and also allows a more extensive set of land-use related indicators, including spatially explicit information on land use, to be reported.
Air pollution implications of the energy system are computed in MESSAGEix by applying technology-specific pollution coefficients from GAINS (see Pollutants and non-GHG forcing agents for MESSAGEix-GLOBIOM and Air pollution and health of MESSAGEix-GLOBIOM). This approach had been applied to the SSP process (Rao et al. 2017)[2]. Alternatively, GAINS can be run ex-post based on MESSAGEix-GLOBIOM scenarios to estimate air pollution emissions, concentrations and the related health impacts. This approach allows for the analysis of different air polllution policy packages (e.g., current legislation, maximum feasible reduction), including the estimation of costs for air pollution control measures. Examples for applying this way of linking MESSAGEix-GLOBIOM and GAINS can be found in McCollum et al (2018)[3] and Grubler et al. (2018)[4].
In general, cumulative global GHG emissions from all sectors are constrained at different levels, with equivalent pricing applied to other GHGs, to reach the desired radiative forcing levels (cf. right-hand side). <xr id="fig:MESSAGE-GLOBIOM_iiasaiam"></xr>The climate constraints are thus taken up in the coupled MESSAGEix-GLOBIOM optimization, and the resulting carbon price is fed back to the full-fledged GLOBIOM model for full consistency. Finally, the combined results for land use, energy, and industrial emissions from MESSAGEix and GLOBIOM are merged and fed into MAGICC (see Climate of MESSAGEix-GLOBIOM), a global carbon-cycle and climate model, which then provides estimates of the climate implications in terms of atmospheric concentrations, radiative forcing, and global-mean temperature increase. Importantly, climate impacts and impacts of the carbon cycle are - depending on the specific application - only partly accounted for in the IIASA IAM framework. The entire framework is linked to an online database infrastructure which allows straightforward visualisation, analysis, comparison and dissemination of results (Riahi et al., 2017)[5].
The scientific software underlying the global MESSAGEix-GLOBIOM model is called the MESSAGEix framework, an open-source, versatile implementation of a linear optimization problem, with the option of coupling to the computable general equilibrium (CGE) model MACRO to incorporate the effect of price changes on economic activity and demand for commodities and resources. MESSAGEix is integrated with the ix modelling platform (ixmp), a "data warehouse" for version control of reference timeseries, input data and model results. ixmp provides interfaces to the scientific programming languages Python and R for efficient, scripted workflows for data processing and visualisation of results (Huppmann et al., 2019)[6].
1.1) Model concept, solver and details - MESSAGE-GLOBIOM
1.3) Temporal dimension - MESSAGE-GLOBIOM
MESSAGEix models the time horizon from 2010 to 2110, generally in 10-year periods (2020, 2030, 2040, 2050, 2060, 2070, 2080, 2090, 2100, 2110), using 2010 as the base year. The 2020 period is partly calibrated so far, with some recent trends included in this time period, but some flexibility remains. The reporting years are the final years in periods, which implies that investments that lead to the capacity in the reporting year are the average annual investments over the entire period the reporting year belongs to. In some model versions, the model has been calibrated to 2015, running with 5-year modeling periods to roughly the middle of the century (2020, 2025, 2030, 2035, 2040, 2045, 2050, 2055, 2060) and 10-year periods between 2060 and 2110.
MESSAGEix can both operate perfect foresight over the entire time horizon, limited foresight (e.g., two or three periods into the future) or myopically, optimizing one period at a time (Keppo and Strubegger, 2010 MSG-GLB_keppo_short_2010). Most frequently MESSAGEix is run with perfect foresight, but for specific applications such as delayed participation in a global climate regime without anticipation (Krey and Riahi, 2009 MSG-GLB_krey_implications_2009; O'Neill et al., 2010 MSG-GLB_oneill_mitigation_2010), limited foresight is used.
GLOBIOM models the time horizon from 2000 to 2100 in 10-year time steps (2000, 2010, 2020, 2030, 2040, 2050, 2060, 2070, 2080, 2090, 2100), with the year 2000 as the base year. The model is recursive-dynamic, i.e. it is solved for each period individually and then passes on results to the subsequent periods. The linkage between MESSAGEix and GLOBIOM relies on the model results of the periods 2020 to 2100.
1.4) Spatial dimension - MESSAGE-GLOBIOM
The combined MESSAGEix-GLOBIOM framework has global coverage and divides the world into 11 regions which are also the native regions of the MESSAGEix model (see Table below). GLOBIOM itseld has 30 regions which are aggregated to the 11 MESSAGEix regions when the two models are linked. In some scenarios, the MESSAGEix region of FSU (Former Soviet Union) is disaggregated into four sub-regions resulting in a 14-region MESSAGEix model.
In addition to the 11 geographical regions, there is a global trade region in MESSAGEix for market clearing of global energy markets and which also represents international shipping bunker fuel demand, uranium resource extraction and the nuclear fuel cycle. <figure id="fig:MESSAGEix-GLOBIOM_regions">
</figure>
<figtable id="tab:MESSAGEix-GLOBIOM_regions">
11 MESSAGE regions | Definition | List of countries |
---|---|---|
NAM | North America | Canada, Guam, Puerto Rico, United States of America, Virgin Islands |
WEU | Western Europe | Andorra, Austria, Azores, Belgium, Canary Islands, Channel Islands, Cyprus, Denmark, Faeroe Islands, Finland, France, Germany, Gibraltar, Greece, Greenland, Iceland, Ireland, Isle of Man, Italy, Liechtenstein, Luxembourg, Madeira, Malta, Monaco, Netherlands, Norway, Portugal, Spain, Sweden, Switzerland, Turkey, United Kingdom |
PAO | Pacific OECD | Australia, Japan, New Zealand |
EEU | Central and Eastern Europe | Albania, Bosnia and Herzegovina, Bulgaria, Croatia, Czech Republic, The former Yugoslav Rep. of Macedonia, Hungary, Poland, Romania, Slovak Republic, Slovenia, Yugoslavia, Estonia, Latvia, Lithuania |
FSU | Former Soviet Union | Armenia, Azerbaijan, Belarus, Georgia, Kazakhstan, Kyrgyzstan, Republic of Moldova, Russian Federation, Tajikistan, Turkmenistan, Ukraine, Uzbekistan |
CPA | Centrally Planned Asia and China | Cambodia, China (incl. Hong Kong), Korea (DPR), Laos (PDR), Mongolia, Viet Nam |
SAS | South Asia | Afghanistan, Bangladesh, Bhutan, India, Maldives, Nepal, Pakistan, Sri Lanka |
PAS | Other Pacific Asia | American Samoa, Brunei Darussalam, Fiji, French Polynesia, Gilbert-Kiribati, Indonesia, Malaysia, Myanmar, New Caledonia, Papua, New Guinea, Philippines, Republic of Korea, Singapore, Solomon Islands, Taiwan (China), Thailand, Tonga, Vanuatu, Western Samoa |
MEA | Middle East and North Africa | Algeria, Bahrain, Egypt (Arab Republic), Iraq, Iran (Islamic Republic), Israel, Jordan, Kuwait, Lebanon, Libya/SPLAJ, Morocco, Oman, Qatar, Saudi Arabia, Sudan, Syria (Arab Republic), Tunisia, United Arab Emirates, Yemen |
LAM | Latin America and the Caribbean | Antigua and Barbuda, Argentina, Bahamas, Barbados, Belize, Bermuda, Bolivia, Brazil, Chile, Colombia, Costa Rica, Cuba, Dominica, Dominican Republic, Ecuador, El Salvador, French Guyana, Grenada, Guadeloupe, Guatemala, Guyana, Haiti, Honduras, Jamaica, Martinique, Mexico, Netherlands Antilles, Nicaragua, Panama, Paraguay, Peru, Saint Kitts and Nevis, Santa Lucia, Saint Vincent and the Grenadines, Suriname, Trinidad and Tobago, Uruguay, Venezuela |
AFR | Sub-Saharan Africa | Angola, Benin, Botswana, British Indian Ocean Territory, Burkina Faso, Burundi, Cameroon, Cape Verde, Central African Republic, Chad, Comoros, Cote d’Ivoire, Congo, Democratic Republic of Congo, Djibouti, Equatorial Guinea, Eritrea, Ethiopia, Gabon, Gambia, Ghana, Guinea, Guinea-Bissau, Kenya, Lesotho, Liberia, Madagascar, Malawi, Mali, Mauritania, Mauritius, Mozambique, Namibia, Niger, Nigeria, Reunion, Rwanda, Sao Tome and Principe, Senegal, Seychelles, Sierra Leone, Somalia, South Africa, Saint Helena, Swaziland, Tanzania, Togo, Uganda, Zambia, Zimbabwe |
</figtable>
1.5) Policy - MESSAGE-GLOBIOM
A number of different energy- and climate-related policies are, depending on the scenario setup and the research question, explicitly represented in MESSAGEix. This includes the following list of policies:
- GHG emission pricing
- GHG emission caps and trading permits
- Renewable energy portfolio standards (e.g., share of renewable energy in electricity generation)
- Renewable energy and other technology capacity targets
- Energy import taxes
- Fuel subsidies and micro-financing for achieving universal access to modern energy services in developing countries
- Air pollution legislation packages (fixed legislation, current and planned legislation, stringent legislation, maximum feasible reduction)
In general, these policies are implemented via constraints or cost coefficients (negative and positive) in the optimization problem. For air pollution policies, the different legislation packages are implemented via a set of emission coefficients and associated costs derived from the GAINS model. The cost coefficients are, however, not part of the optimization procedure, but instead allow an ex-post quantification of air pollution policy costs for a specific energy scenario.
2) Socio-economic drivers - MESSAGE-GLOBIOM
Socio-economic drivers are typically informed by a scenario narrative that describes in qualitative terms the overall logic behind the scenarios. In MESSAGEix-GLOBIOM, the Shared Socio-economic Pathways (SSPs, see O’Neill et al., 2014 MSG-GLB_oneill_new_2014) provide the overall scenario logic with which the main socio-economic drivers, population and GDP have been quantified.
SSP Narratives
Narratives have been developed for the SSPs (O'Neill et al., 2015)[7]. These descriptions of alternative future societal developments span a range of possible worlds that stretch along two climate change-related dimensions: mitigation and adaptation challenges. The SSPs reflect five different development pathways for the world that are characterized by varying levels of glboal challenges (see Riahi et al., 2017)[8]. The three narratives that have been translated into quantitative scenarios with MESSAGEix-GLOBIOM are presented below and in Fricko et al. (2017)[9].
SSP1 - Sustainability - Taking the green road
“The world shifts gradually, but pervasively, toward a more sustainable path, emphasizing more inclusive development that respects perceived environmental boundaries. Increasing evidence of and accounting for the social, cultural, and economic costs of environmental degradation and inequality drive this shift. Management of the global commons slowly improves, facilitated by increasingly effective and persistent cooperation and collaboration of local, national, and international organizations and institutions, the private sector, and civil society. Educational and health investments accelerate the demographic transition, leading to a relatively low population. Beginning with current high-income countries, the emphasis on economic growth shifts toward a broader emphasis on human well-being, even at the expense of somewhat slower economic growth over the longer term. Driven by an increasing commitment to achieving development goals, inequality is reduced both across and within countries. Investment in environmental technology and changes in tax structures lead to improved resource efficiency, reducing overall energy and resource use and improving environmental conditions over the longer term. Increased investment, financial incentives and changing perceptions make renewable energy more attractive. Consumption is oriented toward low material growth and lower resource and energy intensity. The combination of directed development of environmentally friendly technologies, a favorable outlook for renewable energy, institutions that can facilitate international cooperation, and relatively low energy demand results in relatively low challenges to mitigation. At the same time, the improvements in human well-being, along with strong and flexible global, regional, and national institutions imply low challenges to adaptation.” (O'Neill et al., 2015).[7]
SSP2 - Middle of the road
“The world follows a path in which social, economic, and technological trends do not shift markedly from historical patterns. Development and income growth proceed unevenly, with some countries making relatively good progress while others fall short of expectations. Most economies are politically stable. Globally connected markets function imperfectly. Global and national institutions work toward but make slow progress in achieving sustainable development goals, including improved living conditions and access to education, safe water, and health care. Technological development proceeds apace, but without fundamental breakthroughs. Environmental systems experience degradation, although there are some improvements and overall the intensity of resource and energy use declines. Even though fossil fuel dependency decreases slowly, there is no reluctance to use unconventional fossil resources. Global population growth is moderate and levels off in the second half of the century as a consequence of completion of the demographic transition. However, education investments are not high enough to accelerate the transition to low fertility rates in low-income countries and to rapidly slow population growth. This growth, along with income inequality that persists or improves only slowly, continuing societal stratification, and limited social cohesion, maintain challenges to reducing vulnerability to societal and environmental changes and constrain significant advances in sustainable development. These moderate development trends leave the world, on average, facing moderate challenges to mitigation and adaptation, but with significant heterogeneities across and within countries.” (O'Neill et al., 2015).[7]
SSP3 - Regional rivalry - A rocky road
“A resurgent nationalism, concerns about competitiveness and security, and regional conflicts push countries to increasingly focus on domestic or, at most, regional issues. This trend is reinforced by the limited number of comparatively weak global institutions, with uneven coordination and cooperation for addressing environmental and other global concerns. Policies shift over time to become increasingly oriented toward national and regional security issues, including barriers to trade, particularly in the energy resource and agricultural markets. Countries focus on achieving energy and food security goals within their own regions at the expense of broader-based development, and in several regions move toward more authoritarian forms of government with highly regulated economies. Investments in education and technological development decline. Economic development is slow, consumption is material-intensive, and inequalities persist or worsen over time, especially in developing countries. There are pockets of extreme poverty alongside pockets of moderate wealth, with many countries struggling to maintain living standards and provide access to safe water, improved sanitation, and health care for disadvantaged populations. A low international priority for addressing environmental concerns leads to strong environmental degradation in some regions. The combination of impeded development and limited environmental concern results in poor progress toward sustainability. Population growth is low in industrialized and high in developing countries. Growing resource intensity and fossil fuel dependency along with difficulty in achieving international cooperation and slow technological change imply high challenges to mitigation. The limited progress on human development, slow income growth, and lack of effective institutions, especially those that can act across regions, implies high challenges to adaptation for many groups in all regions.” (O'Neill et al., 2015)[7]
2.1) Population - MESSAGE-GLOBIOM
Demographic development has, next to economic growth, strong implications for the anticipated mitigation and adaptation challenges. For example, a larger, poorer population will have more difficulty adapting to the detrimental effects of climate change (O’Neill et al., 2014 MSG-GLB_oneill_new_2014). The primary drivers of future energy demand in MESSAGEix are projections of total population and GDP at purchasing power parity, denoted as GDP (PPP). In addition to total population, the urban/rural split of population is relevant for the MESSAGEix-Access version of the model which distinguishes rural and urban population with different household incomes in developing country regions.
Demographic projections used in MESSAGEix-GLOBIOM are based on the Shared Socio-economic Pathways (SSPs) at the country level SSP database. Population growth evolves in response to how fertility, mortality, migration, and education of various social strata are assumed to change over time. In SSP2, global population peaks at 9.4 billion people around 2070, and slowly declines thereafter (KC and Lutz, 2015 MSG-GLB_kc_human_2014). However, modest improvements of educational attainment levels result in declines in education-specific fertility rates, leading to incomplete economic convergence across different world regions. This is particularly an issue for Africa. Overall, the population development in SSP2 is designed to be situated in the middle of the road between SSP1 and SSP3, see KC and Lutz (2015) MSG-GLB_kc_human_2014 for details. (Fricko et al., 2016 MSG-GLB_fricko_marker_2016)
2.2) Economic activity - MESSAGE-GLOBIOM
In addition to population economic development has a strong impact on the challenges to mitigation and adaptation. Generally, a poorer, less educated population will have more difficulty adapting to the detrimental effects of climate change (O’Neill et al., 2014 MSG-GLB_oneill_new_2014). The primary drivers of future energy demand in MESSAGEix are projections of total population and GDP per capita at purchasing power parity, denoted as GDP (PPP). In the MESSAGEix-Access version of the model households are represented by income level (and rural/urban split) in developing country regions.
MESSAGEix-GLOBIOM utilizes GDP (PPP) projections based on the Shared Socio-economic Pathways (SSPs) that are available at the country level SSP database. In the SSPs, GDP development follows regional historical trends (Dellink et al., 2015 MSG-GLB_dellink_long-term_2015). In SSP2 specifically, average income grows by a factor of six and reaches about 60,000 USD/capita by the end of the century (all GDP/capita figures use USD2005 and purchasing-power-parity – PPP). The SSP2 GDP projection is situated in-between the estimates for SSP1 and SSP3, which reach global average income levels of 82,000 USD2005 and 22,000 USD2005, respectively, by the end of the century. SSP2 depicts a future of global progress where developing countries achieve significant economic growth. Today, average per capita income in the global North is about five times higher than in the global South. In SSP2, developing countries reach today’s average income levels of the OECD between 2060 and 2090, depending on the region. Overall, the GDP developments in SSP2 are designed to be situated in the middle of the road between SSP1 and SSP3, see Dellink et al (2015) MSG-GLB_dellink_long-term_2015 for details. (Fricko et al., 2016 MSG-GLB_fricko_marker_2016)
3) Macro-economy - MESSAGE-GLOBIOM
The detailed energy supply model (MESSAGEix) is soft-linked to an aggregated, single-sector macro-economic model (MACRO) which has been derived from the so-called Global 2100 or ETA-MACRO model (Manne and Richels, 1992 MSG-GLB_manne_buying_1992), a predecessor of the MERGE model. The two models are linked to consistently reflect the influence of energy supply costs, as calculated by MESSAGEix, in the mix of production factors considered in MACRO, and the effect of energy price changes on energy service demand. The combined MESSAGEix-MACRO model (Messner and Schrattenholzer, 2000 MSG-GLB_messner_messagemacro:_2000) can generate a consistent economic response to changes in energy prices and estimate the overall economic consequences (e.g., changes in GDP or household consumption) of energy or climate policies.
ACRO is a macroeconomic model maximizing the intertemporal utility function of a single representative producer-consumer in each world region. The optimization result is a sequence of optimal savings, investment, and consumption decisions. The main variables of the model are capital stock, available labor, and energy inputs, which together determine an economy's total output according to a nested CES (constant elasticity of substitution) production function. End-use service demand in the (commercial) demand categories of MESSAGEix (see Energy demand of MESSAGEix-GLOBIOM) is determined within the MACRO model, and is consistent with energy supply from MESSAGEix, which is an input to the model.
The model’s most important driving input variables are the projected growth rates of total labor, i.e., the combined effect of labor force and labor productivity growth, and the annual rates of reference energy intensity reduction, i.e. the so-called autonomous energy efficiency improvement (AEEI) coefficients. The latter are calibrated to the developments in a MESSAGEix baseline scenario to ensure consistency between the two models. Labor supply growth is also referred to as reference or potential GDP growth. In the absence of price changes, energy demands grow at rates that are the approximate result of potential GDP growth rates, reduced by the rates of overall energy intensity reduction. Price changes in the six demand categories, for example induced by energy or climate policies, can alter this path significantly.
MACRO's production function includes six commercial energy demand categories represented in MESSAGEix. To optimize, MACRO requires cost information for each demand category. The exact definitions of these costs as a function over all positive quantities of energy cannot be given in closed form because each point of the function would be a result of a full MESSAGEix run. However, the optimality conditions implicit in the formulation of MACRO only require the functional values and its derivatives at the optimal point to be consistent between the two models. Since these requirements are therefore only local, most functions with this feature will simulate the combined energy-economic system in the neighborhood of the optimal point. The regional costs (of energy use and imports) and revenues (from energy exports) of providing energy in MACRO are approximated by a Taylor expansion to the first order of the energy system costs as calculated by MESSAGEix. From an initial MESSAGEix model run, the total energy system cost (including costs/revenues from energy trade) and additional abatement costs (e.g., abatement costs from non-energy sources) as well as the shadow prices of the six commercial demand categories by region are passed to MACRO. In addition to the economic implications of energy trade, the data exchange from MESSAGEix to MACRO may also include the revenues or costs of trade if GHG permits.
For a more elaborate description of MACRO, including the system of equations and technical details of the implementation, please consult the annex presenting the mathematical formulation of MACRO in Appendices of MESSAGEix-GLOBIOM.
3.4) Trade - MESSAGE-GLOBIOM
4) Energy - MESSAGE-GLOBIOM
The MESSAGEix modelling framework (Model for Energy Supply Strategy Alternatives and their General Environmental Impact), MESSAGEix for short, is a linear programming (LP) energy engineering model with global coverage. As a systems engineering optimization model, MESSAGEix is used for medium- to long-term energy system planning, energy policy analysis, and scenario development (Huppman et al., 2019[10]; Messner and Strubegger, 1995[11] MSG-GLB_messner_users_1995). The model provides a framework for representing an energy system with all its interdependencies from resource extraction, imports and exports, conversion, transport, and distribution, to the provision of energy end-use services such as light, space conditioning, industrial production processes, and transportation. In addition, MESSAGEix links to GLOBIOM (GLObal BIOsphere Model, cf. Section Land-use of MESSAGEix-GLOBIOM) to consistently assess the implications of utilizing bioenergy of different types and to integrate the GHG emissions from energy and land us,e and to the aggregated macro-economic model MACRO (cf. Section Macro-economy of MESSAGEix-GLOBIOM) to assess economic implications and to capture economic feedbacks.
MESSAGEix covers all greenhouse gas (GHG)-emitting sectors, including energy, industrial processes as well as - through its linkage to GLOBIOM - agriculture and forestry. The emissions of the full basket of greenhouse gases, including CO2, CH4, N2O and F-gases (CF4, C2F6, HFC125, HFC134a, HFC143a, HFC227ea, HFC245ca and SF6), as well as other radiatively active gases, such as NOx, volatile organic compounds (VOCs), CO, SO2, and BC/OC, is represented in the model. MESSAGEix is used in conjunction with MAGICC (Model for Greenhouse gas Induced Climate Change) version 6.8 (cf. Section Climate of MESSAGEix-GLOBIOM) to calculate atmospheric concentrations, radiative forcing, and annual-mean global surface air temperature increase.
The model is designed to formulate and evaluate alternative energy supply strategies consistent with user-defined constraints such as limits on new investment, fuel availability and trade, environmental regulations and policies as well as diffusion rates of new technology. Environmental aspects can be analysed by accounting for, and if necessary limiting, the amount of pollutants emitted by various technologies at various steps in energy supply. This helps to evaluate the impact of environmental regulations on energy system development.
Its principal results comprise, among others, estimates of technology-specific multi-sector response strategies for specific climate stabilization targets. The model thus identifies the least-cost portfolio of mitigation technologies. The choice of individual mitigation options across gases and sectors is driven by the relative economics of abatement measures, assuming full temporal and spatial flexibility (i.e., emissions-reduction measures are assumed to occur when and where they are cheapest to implement).
The Reference Energy System (RES) defines the total set of available energy conversion technologies. In MESSAGEix terms, energy conversion technology refers to all types of energy technology, from resource extraction to transformation, transport, distribution of energy carriers, and end-use technologies.
Because few conversion technologies convert resources directly into useful energy, the energy system in MESSAGEix is divided into 5 energy levels:
- Resource (r) - raw resources (e.g., coal, oil, natural gas in the ground or biomass on the field)
- Primary (a) energy - raw product at a generation site (e.g., crude oil input to the refinery)
- Secondary (x) energy - final product at a generation site (e.g., gasoline or diesel fuel output from the refinery)
- Final (f) energy - final product at its consumption point (e.g., gasoline in the tank of a car or electricity leaving a socket)
- Useful (u) energy - final product satisfying demand for services (e.g., heating, lighting or moving people)
Technologies can take in energy commodities from one level and put them out at another level (e.g., refineries produce refined oil products at a secondary level from crude oil input at the primary level) or at the same level (e.g., hydrogen electrolyzers produce hydrogen at the secondary energy level from electricity at the secondary level). The energy forms defined in each level can be envisioned as a transfer hub that the various technologies feed into or pump away from. The useful energy demand is given as a time series. Technology characteristics generally vary over time periods.
The mathematical formulation of MESSAGEix ensures that the flows are consistent: demand is met, inflows equal outflows and constraints are not exceeded. In other words, MESSAGEix is itself a partial equilibrium model. However, through its linkag to MACRO, general equilibrium effects are taken into account.
4.1) Energy resource endowments - MESSAGE-GLOBIOM
Information about the MESSAGEix-GLOBIOM energy resource endowments can be found in the following subsections:
- Fossil energy resources - MESSAGEix-GLOBIOM
- Uranium and other fissile resources - MESSAGEix-GLOBIOM
- Bioenergy - MESSAGEix-GLOBIOM
- Non-biomass renewables - MESSAGEix-GLOBIOM
4.1.1) Fossil energy resources - MESSAGE-GLOBIOM
Fossil Fuel Reserves and Resources
The availability and costs of fossil fuels influences the future direction of the energy system, and therewith future mitigation challenges. Understanding the variations in fossil fuel availability and the underlying extraction cost assumptions across the SSPs is hence important. Our fossil energy resource assumptions are derived from various sources, including global databases such as The Federal Institute for Geosciences and Natural Resources (BGR) and the U.S. Geological Survey (USGS), as well as market reports and outlooks provided by different energy institutes and agencies. The availability of fossil energy resources in different regions under different socio-economic assumptions are then aligned with the storylines of the individual SSPs (Rogner, 1997 MSG-GLB_rogner_assessment_1997; Riahi et al., 2012 MSG-GLB_riahi_chapter_2012). While the physical resource base is identical across the SSPs, considerable differences are assumed regarding the technical and economic availability of overall resources, for example, of unconventional oil and gas.
What ultimately determines the attractiveness of a particular type of resource is not just the cost at which it can be brought to the surface, but the cost at which it can be used to provide energy services. Assumptions about fossil energy resources should thus be considered together with those of related conversion technologies. In line with the narratives, technological change in fossil fuel extraction and conversion technologies is assumed to be slowest in SSP1, while comparatively faster technological change occurs in SSP3 thereby considerably enlarging the economic potential of coal and unconventional hydrocarbons ( <xr id="tab:MESSAGE-GLOBIOM_globff"></xr>, <xr id="fig:MESSAGE-GLOBIOM_supply"></xr>). However, driven by the tendency toward regional fragmentation, the focus in SSP3 is assumed to be on developing coal technologies which leads to a replacement of oil products by synthetic fuels in the longer-term based on coal-to-liquids technology. In contrast, for SSP2 we assume a continuation of recent trends, focusing more on developing extraction technologies for unconventional hydrocarbon resources, thereby leading to higher potential cumulative oil extraction than in the other SSPs (<xr id="fig:MESSAGE-GLOBIOM_supply"></xr>, middle panel).
<xr id="tab:MESSAGE-GLOBIOM_globff"></xr> shows the assumed total quantities of fossil fuel resources in the MESSAGE model for the base year 2005. <xr id="fig:MESSAGE-GLOBIOM_supply"></xr> gives these resource estimates as supply curves. In addition, the assumptions are compared with estimates from the Global Energy Assessment (Rogner et al., 2012 MSG-GLB_rogner_chapter_2012) and the databases mentioned earlier. Estimating fossil fuel reserves is built on both economic and technological assumptions. With an improvement in technology or a change in purchasing power, the amount that may be considered a “reserve” vs. a “resource” (generically referred to here as resources) can actually vary quite widely.
‘Reserves’ are generally defined as being those quantities for which geological and engineering information indicate with reasonable certainty that they can be recovered in the future from known reservoirs under existing economic and operating conditions. ‘Resources’ are detected quantities that cannot be profitably recovered with current technology, but might be recoverable in the future, as well as those quantities that are geologically possible, but yet to be found. The remainder are ‘Undiscovered resources’ and, by definition, one can only speculate on their existence. Definitions are based on Rogner et al. (2012) MSG-GLB_rogner_chapter_2012.
<figtable id="tab:MESSAGE-GLOBIOM_globff">
Source | MESSAGE (Rogner et al., 1997 MSG-GLB_rogner_assessment_1997) | Rogner et al., 2012 MSG-GLB_rogner_chapter_2012 | |
---|---|---|---|
Reserves+Resources [ZJ] | Reserves [ZJ] | Resources [ZJ] | |
Coal | 259 | 17.3 – 21.0 | 291 – 435 |
Conventional Oil | 9.8 | 4.0 – 7.6 | 4.2 – 6.2 |
Unconventional Oil | 23.0 | 3.8 – 5.6 | 11.3 – 14.9 |
Conventional Gas | 16.8 | 5.0 – 7.1 | 7.2 – 8.9 |
Unconventional Gas | 23.0 | 20.1 – 67.1 | 40.2 – 122 |
</figtable>
The following table (<xr id="tab:MESSAGE-GLOBIOM_ffavail"></xr>) presents the fossil resource availability in ZJ (2010-2100) for coal, oil and gas, for SSP1, SSP2 and SSP3, respectively.
<figtable id="tab:MESSAGE-GLOBIOM_ffavail">
Type | SSP1 [ZJ] | SSP2 [ZJ] | SSP3 [ZJ] |
---|---|---|---|
Coal | 93 | 92 | 243 |
Oil | 17 | 40 | 17 |
Gas | 39 | 37 | 24 |
</figtable>
Coal is the largest resource among fossil fuels; it accounts for more than 50% of total fossil reserve plus resource estimates even at the higher end of the assumptions, which includes considerable amounts of unconventional hydrocarbons. Oil is the most vulnerable fossil fuel at less than 10 ZJ of conventional oil and possibly less than 10 ZJ of unconventional oil. Natural gas is more abundant in both the conventional and unconventional categories.
<xr id="fig:MESSAGE-GLOBIOM_supply"></xr> presents the cumulative global resource supply curves for coal, oil and gas in the IIASA IAM framework. Green shaded resources are technically and economically extractable in all SSPs, purple shaded resources are additionally available in SSP1 and SSP2 and blue shaded resources are additionally available in SSP2. Coloured vertical lines represent the cumulative use of each resource between 2010 and 2100 in the SSP baselines (see top panel for colour coding), and are thus the result of the combined effect of the assumptions on fossil resource availability and conversion technologies in the SSP baselines.
<figure id="fig:MESSAGE-GLOBIOM_supply">
</figure>
Conventional oil and gas are distributed unevenly throughout the world, with only a few regions dominating the reserves. Nearly half of the reserves of conventional oil is found in Middle East and North Africa, and close to 40% of conventional gas is found in Russia and the former Soviet Union states. The situation is somewhat different for unconventional oil of which North and Latin America potentially possess significantly higher global shares. Unconventional gas in turn is distributed quite well throughout the world, with North America holding most (roughly 25% of global resources). The distribution of coal reserves shows the highest geographical diversity which in the more fragmented SSP3 world contributes to increased overall reliance on this resource. Russia and the former Soviet Union states, Pacific OECD, North America, and Centrally Planned Asia and China all possess more than 10 ZJ of reserves.
4.1.2) Uranium and other fissile resources - MESSAGE-GLOBIOM
Nuclear Resources
Estimates of available uranium resources in the literature vary considerably, which could become relevant if advanced nuclear fuel cycles (e.g., the plutonium cycle including fast breeder reactors, the thorium cycle) are not available. In MESSAGE advanced nuclear cycles such as the plutonium cycle and nuclear fuel reprocessing are in principle represented, but their availability varies following the scenario narrative. <xr id="fig:MESSAGE-GLOBIOM_uran"/> below shows the levels of uranium resources assumed available in MESSAGE scenarios, building upon the Global Energy Assessment scenarios (see Riahi et al., 2012 MSG-GLB_riahi_chapter_2012). These span a considerable range of the estimates in the literature, but at the same time none of them fall at the extreme ends of the spectrum (see Rogner et al., 2012 MSG-GLB_rogner_chapter_2012, Section 7.5.2 for a more detailed discussion of uranium resources). Nuclear resources and fuel cycle are modeled at the global level.
<figure id="fig:MESSAGE-GLOBIOM_uran">
</figure>
<xr id="fig:MESSAGE-GLOBIOM_uran"/> presents the global uranium resources in the MESSAGE interpretation of the SSPs compared to seven supply curves from a literature review (Schneider and Sailor, 2008 MSG-GLB_schneider_long-term_2008). Conservative Crustal and Optimistic Crustal refer to simple crustal models of uranium distribution in the crust and the of extraction costs on the concentration. Pure-KCR refers to a fit of a simple crustal model to known conventional resources (KCR) as estimated by the Red Book 2003 (OECD/NEA 2004 MSG-GLB_oecd_uranium_2003). PPM-Cost over the simple crustal models include a relationship between uranium grade and extraction costs. FCCCG(1) and (2) as well as DANESS refer to estimates from more complicated models of the dependency of extraction costs on uranium concentration (and therefore resource grade).
4.1.3) Bioenergy - MESSAGE-GLOBIOM
Biomass energy is another potentially important renewable energy resource in the MESSAGE-GLOBIOM model. This includes both commercial and non-commercial use. Commercial refers to the use of bioenergy in, for example, power plants or biofuel refineries, while non-commercial refers to the use of bioenergy for residential heating and cooking, primarily in rural households of today’s developing countries. Bioenergy potentials differ across SSPs as a result of different levels of competition over land for food and fibre, but ultimately only vary to a limited degree (<xr id="fig:MESSAGE-GLOBIOM_beavail"/>). The drivers underlying this competition are different land-use developments in the SSPs, which are determined by agricultural productivity and global demand for food consumption. (Fricko et al., 2016 MSG-GLB_fricko_marker_2016)
Availability of bioenergy is presented in <xr id="fig:MESSAGE-GLOBIOM_beavail"/> at different price levels in the MESSAGE-GLOBIOM model for the three SSPs (Fricko et al., 2016 MSG-GLB_fricko_marker_2016). Typically non-commercial biomass is not traded or sold, however in some cases there is a market – prices range from 0.1-1.5$/GJ (Pachauri et al., 2013 MSG-GLB_pachauri_pathways_2013) ($ equals 2005 USD).
4.1.4) Non-biomass renewables - MESSAGE-GLOBIOM
<xr id="tab:MESSAGE-GLOBIOM_depl"/> shows the assumed total potentials of non-biomass renewable energy deployment (by resource type) in the MESSAGE model. In addition, the assumptions are compared with technical potential estimates from the Global Energy Assessment (Rogner et al., 2012 MSG-GLB_rogner_chapter_2012). In this context, it is important to note that typical MESSAGE scenarios do not consider the full technical potential of renewable energy resources, but rather only a subset of those potentials, owing to additional constraints (e.g., sustainability criteria, technology diffusion and systems integration issues, and other economic considerations) that may not be fully captured within the model. These constraints may lead to a significant reduction of the technical potential.
<figtable id="tab:MESSAGE-GLOBIOM_depl">
Source | MESSAGE | Rogner et al., 2012 MSG-GLB_rogner_chapter_2012 |
---|---|---|
Deployment Potential [EJ/yr] | Technical Potential [EJ/yr] | |
Hydro | 38 | 50 - 60 |
Wind (on-/offshore) | 689/287 | 1250 - 2250 |
Solar PV | 6064 | 62,000 - 280,000 |
CSP | 2132 | same as Solar PV above |
Geothermal | 23 | 810 - 1400 |
</figtable>
Notes: MESSAGE-GLOBIOM renewable energy potentials are based on Pietzcker et al. (2014) MSG-GLB_pietzcker_solar_2014, Eurek et al. (in review) MSG-GLB_eurek_wind_2016, Christiansson (1995) MSG-GLB_christiansson_diffusion_1995, and Rogner et al (2012) MSG-GLB_rogner_chapter_2012. The potentials for non-combustible renewable energy sources are specified in terms of the electricity or heat that can be produced by specific technologies (i.e., from a secondary energy perspective). By contrast, the technical potentials from Rogner et al (2012) MSG-GLB_rogner_chapter_2012 refer to the flows of energy that could become available as inputs for technology conversion. So for example, the technical potential for wind is given as the kinetic energy available for wind power generation, whereas the deployment potential would only be the electricity that could be generated by the wind turbines.
Regional resource potentials for solar and wind are classified according to resource quality (annual capacity factor) based on Pietzcker et al. (2014 MSG-GLB_pietzcker_solar_2014) and Eurek et al. (in review MSG-GLB_eurek_wind_2016). Regional resource potentials as implemented into MESSAGE-GLOBIOM are provided by region and capacity factor for solar PV, concentrating solar power (CSP), and onshore/offshore wind in Johnson et al. (in review MSG-GLB_johnson_vre_2016). The physical potential of these sources is assumed to be the same across all SSPs. <xr id="tab:MESSAGE-GLOBIOM_pv"/>, <xr id="tab:MESSAGE-GLOBIOM_csp"/>, <xr id="tab:MESSAGE-GLOBIOM_onshorewind"/>, <xr id="tab:MESSAGE-GLOBIOM_offshorewind"/> show the resource potential for solar PV, CSP, on- and offshore wind respectively. For wind, <xr id="tab:MESSAGE-GLOBIOM_capfactonshore"/> and <xr id="tab:MESSAGE-GLOBIOM_capfactoffshore"/> list the capacity factors corresponding to the wind classes used in the resource tables. It is important to note that part of the resource that is useable at economically competitive costs is assumed to differ widely (see Section Energy Conversion of MESSAGE-GLOBIOM).
<figtable id="tab:MESSAGE-GLOBIOM_pv">
Capacity Factor (fraction per year) | ||||||||
---|---|---|---|---|---|---|---|---|
0.28 | 0.21 | 0.20 | 0.19 | 0.18 | 0.17 | 0.15 | 0.14 | |
AFR | 0.0 | 1.1 | 46.5 | 176.6 | 233.4 | 218.2 | 169.9 | 61.9 |
CPA | 0.0 | 0.0 | 0.0 | 10.3 | 194.3 | 315.5 | 159.4 | 41.9 |
EEU | 0.0 | 0.0 | 0.0 | 0.0 | 0.0 | 0.0 | 0.1 | 1.0 |
FSU | 0.0 | 0.0 | 0.0 | 0.2 | 2.8 | 23.6 | 94.9 | 116.6 |
LAM | 0.1 | 4.9 | 49.4 | 165.6 | 157.5 | 167.4 | 81.4 | 48.5 |
MEA | 0.2 | 3.1 | 100.8 | 533.6 | 621.8 | 310.1 | 75.3 | 14.5 |
NAM | 0.0 | 0.3 | 24.3 | 140.4 | 131.0 | 116.3 | 155.7 | 106.4 |
PAO | 0.0 | 0.0 | 0.1 | 2.2 | 53.1 | 226.4 | 311.2 | 158.9 |
PAS | 0.0 | 0.0 | 0.0 | 0.2 | 0.8 | 17.0 | 31.2 | 12.8 |
SAS | 0.0 | 0.0 | 6.1 | 42.7 | 67.2 | 82.3 | 23.7 | 4.1 |
WEU | 0.0 | 0.1 | 0.2 | 3.0 | 12.8 | 39.4 | 58.3 | 33.3 |
Global | 0.3 | 9.6 | 227.4 | 1074.7 | 1474.6 | 1516.3 | 1160.9 | 600.0 |
</figtable>
<figtable id="tab:MESSAGE-GLOBIOM_csp">
Capacity Factor (fraction of year) | ||||||||
---|---|---|---|---|---|---|---|---|
SM1 | 0.27 | 0.25 | 0.23 | 0.22 | 0.20 | 0.18 | 0.17 | 0.15 |
SM3 | 0.75 | 0.68 | 0.64 | 0.59 | 0.55 | 0.50 | 0.46 | 0.41 |
AFR | 0.0 | 3.6 | 19.0 | 81.6 | 106.7 | 62.8 | 59.6 | 37.8 |
CPA | 0.0 | 0.0 | 0.0 | 0.0 | 0.0 | 0.3 | 11.5 | 53.0 |
EEU | 0.0 | 0.0 | 0.0 | 0.0 | 0.0 | 0.0 | 0.0 | 0.0 |
FSU | 0.0 | 0.0 | 0.0 | 0.0 | 0.0 | 0.1 | 0.4 | 6.1 |
LAM | 0.0 | 2.0 | 7.0 | 11.8 | 29.3 | 57.1 | 56.8 | 53.5 |
MEA | 0.1 | 3.7 | 24.8 | 122.4 | 155.3 | 144.5 | 68.4 | 34.0 |
NAM | 0.0 | 0.0 | 0.0 | 6.3 | 19.7 | 20.2 | 29.6 | 43.2 |
PAO | 0.0 | 3.0 | 75.1 | 326.9 | 158.3 | 140.4 | 40.2 | 10.2 |
PAS | 0.0 | 0.0 | 0.0 | 0.0 | 0.0 | 0.0 | 0.1 | 0.6 |
SAS | 0.0 | 0.0 | 0.0 | 0.1 | 3.9 | 8.7 | 16.1 | 9.8 |
WEU | 0.0 | 0.0 | 0.0 | 0.0 | 0.2 | 0.7 | 2.4 | 3.0 |
Global | 0.1 | 12.3 | 126.0 | 549.2 | 473.3 | 434.8 | 285.0 | 251.3 |
</figtable>
<figtable id="tab:MESSAGE-GLOBIOM_onshorewind">
Wind Class | ||||||
---|---|---|---|---|---|---|
3 | 4 | 5 | 6 | 7 | 8+ | |
AFR | 38.2 | 21.3 | 13.4 | 6.8 | 2.6 | 2.1 |
CPA | 24.7 | 11.4 | 5.4 | 2.6 | 0.3 | 0.0 |
EEU | 6.1 | 5.7 | 0.3 | 0.0 | 0.0 | 0.0 |
FSU | 52.3 | 83.8 | 5.8 | 0.8 | 0.0 | 0.0 |
LAM | 33.5 | 15.9 | 9.6 | 5.7 | 3.9 | 3.7 |
MEA | 56.1 | 22.2 | 6.0 | 2.1 | 0.9 | 0.3 |
NAM | 28.6 | 66.4 | 23.7 | 1.5 | 0.4 | 0.0 |
PAO | 18.9 | 18.8 | 3.6 | 1.4 | 1.8 | 0.5 |
PAS | 5.2 | 2.9 | 0.8 | 0.2 | 0.0 | 0.0 |
SAS | 12.3 | 7.9 | 2.4 | 1.6 | 0.9 | 0.3 |
WEU | 16.1 | 10.5 | 6.6 | 8.2 | 3.7 | 0.6 |
World | 292.1 | 266.8 | 77.5 | 30.9 | 14.3 | 7.5 |
</figtable>
<figtable id="tab:MESSAGE-GLOBIOM_capfactonshore">
Wind Class | ||||||
---|---|---|---|---|---|---|
3 | 4 | 5 | 6 | 7 | 8+ | |
AFR | 0.24 | 0.28 | 0.32 | 0.36 | 0.40 | 0.45 |
CPA | 0.24 | 0.28 | 0.32 | 0.36 | 0.38 | 0.45 |
EEU | 0.24 | 0.27 | 0.31 | 0.36 | 0.38 | 0.45 |
FSU | 0.24 | 0.28 | 0.31 | 0.35 | 0.38 | 0.45 |
LAM | 0.24 | 0.28 | 0.32 | 0.36 | 0.39 | 0.46 |
MEA | 0.24 | 0.27 | 0.32 | 0.35 | 0.39 | 0.45 |
NAM | 0.24 | 0.28 | 0.31 | 0.36 | 0.39 | 0.45 |
PAO | 0.24 | 0.28 | 0.32 | 0.36 | 0.40 | 0.43 |
PAS | 0.24 | 0.27 | 0.32 | 0.35 | 0.40 | 0.45 |
SAS | 0.24 | 0.27 | 0.32 | 0.36 | 0.39 | 0.42 |
WEU | 0.24 | 0.28 | 0.32 | 0.36 | 0.39 | 0.43 |
</figtable>
<figtable id="tab:MESSAGE-GLOBIOM_offshorewind">
Wind Class | ||||||
---|---|---|---|---|---|---|
3 | 4 | 5 | 6 | 7 | 8+ | |
AFR | 3.1 | 2.4 | 2.0 | 2.0 | 1.1 | 1.7 |
CPA | 3.5 | 4.3 | 2.6 | 0.9 | 1.3 | 0.1 |
EEU | 0.7 | 0.6 | 1.0 | 0.0 | 0.0 | 0.0 |
FSU | 1.8 | 4.6 | 14.2 | 13.3 | 4.3 | 0.7 |
LAM | 7.1 | 7.3 | 5.3 | 2.7 | 2.6 | 5.9 |
MEA | 3.2 | 0.9 | 0.8 | 0.9 | 0.6 | 0.9 |
NAM | 4.5 | 18.2 | 24.0 | 16.0 | 7.3 | 2.1 |
PAO | 5.8 | 11.2 | 15.3 | 9.8 | 2.6 | 2.5 |
PAS | 5.3 | 6.6 | 4.7 | 1.5 | 0.1 | 0.0 |
SAS | 1.9 | 0.9 | 0.6 | 0.5 | 0.0 | 0.0 |
WEU | 3.5 | 4.7 | 8.8 | 12.9 | 10.3 | 0.9 |
World | 40.4 | 61.5 | 79.4 | 60.5 | 30.3 | 14.8 |
</figtable>
<figtable id="tab:MESSAGE-GLOBIOM_capfactoffshore">
Wind Class | ||||||
---|---|---|---|---|---|---|
3 | 4 | 5 | 6 | 7 | 8+ | |
AFR | 0.24 | 0.28 | 0.32 | 0.36 | 0.41 | 0.47 |
CPA | 0.24 | 0.28 | 0.32 | 0.36 | 0.40 | 0.42 |
EEU | 0.24 | 0.29 | 0.32 | 0.34 | 0.40 | 0.42 |
FSU | 0.25 | 0.28 | 0.32 | 0.35 | 0.39 | 0.43 |
LAM | 0.24 | 0.28 | 0.32 | 0.36 | 0.40 | 0.49 |
MEA | 0.24 | 0.28 | 0.32 | 0.36 | 0.40 | 0.45 |
NAM | 0.25 | 0.28 | 0.32 | 0.36 | 0.40 | 0.43 |
PAO | 0.24 | 0.28 | 0.32 | 0.36 | 0.40 | 0.47 |
PAS | 0.24 | 0.28 | 0.32 | 0.35 | 0.39 | 0.42 |
SAS | 0.24 | 0.27 | 0.32 | 0.36 | 0.40 | 0.42 |
WEU | 0.24 | 0.28 | 0.32 | 0.36 | 0.40 | 0.42 |
</figtable>
4.2) Energy conversion - MESSAGE-GLOBIOM
Energy technologies are characterized by numerical model inputs describing their economic (e.g., investment costs, fixed and variable operation and maintenance costs), technical (e.g., conversion efficiencies), ecological (e.g., GHG and pollutant emissions), and sociopolitical characteristics. An example for the sociopolitical situation in a world region would be the decision by a country or world region to ban certain types of power plants (e.g., nuclear plants). Model input data reflecting this situation would be upper bounds of zero for these technologies or, equivalently, their omission from the data set for this region altogether.
Each energy conversion technology is characterized in MESSAGE by the following data:
- Energy inputs and outputs together with the respective conversion efficiencies. Most energy conversion technologies have one energy input and one output and thereby one associated efficiency. But technologies may also use different fuels (either jointly or alternatively), may have different operation modes and different outputs, which also may have varying shares. An example of different operation modes would be a passout turbine, which can generate electricity and heat at the same time when operated in co-generation mode or which can produce electricity only. For each technology, one output and one input are defined as main output and main input respectively. The activity variables of technologies are given in the units of the main input consumed by the technology or, if there is no explicit input (as for solar-energy conversion technologies), in units of the main output.
- Specific investment costs (e. g., per kilowatt, kW) and time of construction as well as distribution of capital costs over construction time.
- Fixed operating and maintenance costs (per unit of capacity, e.g., per kW).
- Variable operating costs (per unit of output, e.g. per kilowatt-hour, kWh, excluding fuel costs).
- Plant availability or maximum utilization time per year. This parameter also reflects maintenance periods and other technological limitations that prevent the continuous operation of the technology.
- Technical lifetime of the conversion technology in years.
- Year of first commercial availability and last year of commercial availability of the technology.
- Consumption or production of certain materials (e.g. emissions of kg of CO2 or SO2 per produced kWh).
- Limitations on the (annual) activity and on the installed capacity of a technology.
- Constraints on the rate of growth or decrease of the annually new installed capacity and on the growth or decrease of the activity of a technology.
- Technical application constraints, e.g., maximum possible shares of wind or solar power in an electricity network without storage capabilities.
- Inventory upon startup and shutdown, e.g., initial nuclear core needed at the startup of a nuclear power plant.
- Lag time between input and output of the technology.
- Minimum unit size, e.g. for nuclear power plants it does not make sense to build plants with a capacity of a few kilowatt power (optional, not used in current model version).
- Sociopolitical constraints, e.g., ban of nuclear power plants, or inconvenience costs of household cook stoves.
- Inconvenience costs which are specified only for end-use technologies (e.g. cook stoves)
The specific technologies represented in various parts of the energy conversion sector are discussed in the following sections on Electricity, Heat, Other conversion, and Grid, Infrastructure and System Reliability below.
4.2.1) Electricity - MESSAGE-GLOBIOM
MESSAGE covers a large number of electricity generation options utilizing a wide range of primary energy sources. For fossil-based electricity generation technologies, typically a number of different technologies with different efficiencies, environmental characteristics and costs is represented. For example, in the case of coal, MESSAGE distinguishes subcritical and supercritical pulverized coal (PC) power plants where the subcritical variant is available with and without flue gas desulpherization/denox and one internal gasification combined cycle (IGCC) power plant. The superciritical PC and IGCC plants are also available with carbon capture and storage (CCS) which also can be retrofitted to some of the existing PC power plants. <xr id="tab:MESSAGE-GLOBIOM_elec"/> below shows the different power plant types represented in MESSAGE.
Four different nuclear power plant types are represented in MESSAGE-GLOBIOM, i.e. two light water reactor types, a fast breeder reactor and a high temperature reactor, but only the two light water types are included in the majority of scenarios being developed with MESSAGE in the recent past. In addition, MESSAGE includes a representation of the nuclear fuel cycle, including reprocessing and the plutonium fuel cycle, and keeps track of the amounts of nuclear waste being produced.
The conversion of five renewable energy sources to electricity is represented in MESSAGE-GLOBIOM (see <xr id="tab:MESSAGE-GLOBIOM_elec"/>). For wind power, both on- and offshore electricity generation are covered and for solar energy, photovoltaics (PV) and solar thermal (concentrating solar power, CSP) electricity generation are included in MESSAGE (see also sections on Non-biomass renewables of MESSAGE-GLOBIOM and Grid, pipelines and other infrastructure of MESSAGE-GLOBIOM).
Most thermal power plants offer the option of coupled heat production (CHP, see <xr id="tab:MESSAGE-GLOBIOM_elec"/>). This option is modeled as a passout turbine via a penalty on the electricity generation efficiency. In addition to the main electricity generation technologies described in this section, also the co-generation of electricity in conversion technologies primarily devoted to producing non-electric energy carriers (e.g., synthetic liquid fuels) is included in MESSAGE (see sections on Liquid fuels of MESSAGE-GLOBIOM and Gaesous fuels of MESSAGE-GLOBIOM).
<figtable id="tab:MESSAGE-GLOBIOM_elec">
Energy source | Technology | CHP option |
---|---|---|
Coal | subcritical PC power plant without desulphurization/denox | yes |
subcritical PC power plant with desulphurization/denox | yes | |
supercritical PC power plant with desulphurization/denox | yes | |
supercritical PC power plant with desulphurization/denox and CCS | yes | |
IGCC power plant | yes | |
IGCC power plant with CCS | yes | |
Oil | heavy fuel oil steam power plant | yes |
light fuel oil steam power plant | yes | |
light fuel oil combined cycle power plant | yes | |
Gas | gas steam power plant | yes |
gas combustion turbine gas | yes | |
combined cycle power plant | yes | |
Nuclear | nuclear light water reactor (Gen II) | yes |
nuclear light water reactor (Gen III+) | yes | |
fast breeder reactor | ||
high temperature reactor | ||
Biomass | biomass steam power plant | yes |
biomass IGCC power plant | yes | |
biomass IGCC power plant with CCS | yes | |
Hydro | hydro power plant (2 cost categories) | no |
Wind | onshore wind turbine | no |
offshore wind turbine | no | |
Solar | solar photovoltaics (PV) | no |
concentrating solar power (CSP) | ||
Geothermal | geothermal power plant | yes |
</figtable>
Technological change in MESSAGE is generally treated exogenously, although pioneering work on the endogenization of technological change in energy-engineering type models has been done with MESSAGE (Messner, 1997 MSG-GLB_messner_endogenized_1997). The current cost and performance parameters, including conversion efficiencies and emission coefficients is generally derived from the relevant engineering literature. For the future alternative cost and performance projections are usually developed to cover a relatively wide range of uncertainties that influences model results to a good extent. As an example, <xr id="fig:MESSAGE-GLOBIOM_ther"/> and <xr id="fig:MESSAGE-GLOBIOM_nonth"/> below provide an overview of costs ranges for a set of key energy conversion technologies (Fricko et al., 2016 MSG-GLB_fricko_marker_2016).
In <xr id="fig:MESSAGE-GLOBIOM_ther"/>, the black ranges show historical cost ranges for 2005. Green, blue, and red ranges show cost ranges in 2100 for SSP1, SSP2, and SSP3, respectively (see descriptions of the SSP narratives in section ADD LINKS ONCE SSP INFO HAS BEEN ADDED). Global values are represented by solid ranges. Values in the global South are represented by dashed ranges. The diamonds show the costs in the “North America” region. CCS – Carbon Capture and Storage; IGCC – Integrated gasification combined cycles; ST – Steam turbine; CT – Combustion turbine; CCGT – Combined cycle gas turbine (Fricko et al., 2016 MSG-GLB_fricko_marker_2016).
In <xr id="fig:MESSAGE-GLOBIOM_nonth"/>, the black ranges show historical cost ranges for 2005. Green, blue, and red ranges show cost ranges in 2100 for SSP1, SSP2, and SSP3, respectively. Global values are represented by solid ranges. Values in the global South are represented by dashed ranges. The diamonds show the costs in the “North America” region. PV – Photovoltaic (Fricko et al., 2016 MSG-GLB_fricko_marker_2016).
4.2.2) Heat - MESSAGE-GLOBIOM
A number centralized district heating technologies based on fossil and renewable energy sources are represented in MESSAGE (see <xr id="tab:MESSAGE-GLOBIOM_heat"/>). Similar to coupled heat and power (CHP) technologies that are described in the Electricity section of MESSAGE-GLOBIOM, these heating plants feed low temperature heat into the district heating system that is then used in the end-use sectors. In addition, there are (decentralized) heat generation options in Industrial sector of MESSAGE-GLOBIOM and Residential and commercial sectors of MESSAGE-GLOBIOM.
<figtable id="tab:MESSAGE-GLOBIOM_heat">
Energy Source | Technology |
---|---|
coal | coal district heating plant |
oil | light fuel oil district heating plant |
gas | gas district heating plant |
biomass | solid biomass district heating plant |
geothermal | geothermal district heating plant |
</figtable>
4.2.3) Gaseous fuels - MESSAGE-GLOBIOM
See <xr id="tab:MESSAGE-GLOBIOM_gasfuel"/> for a list of gaseous fuel production technologies in MESSAGE.
<figtable id="tab:MESSAGE-GLOBIOM_gasfuel">
Energy Source | Technology |
---|---|
Biomass | biomass gasification |
biomass gasification with CCS | |
Coal | coal |
coal gasification with CCS |
</figtable>
Hydrogen Production
See <xr id="tab:MESSAGE-GLOBIOM_hydtech"/> for a list of hydrogen production technologies in MESSAGE.
<figtable id="tab:MESSAGE-GLOBIOM_hydtech">
Energy source | Technology | Electricity cogeneration |
---|---|---|
Gas | steam methane reforming | yes |
steam methane reforming with CCS | no | |
Electricity | electrolysis | no |
Coal | coal gasification | yes |
coal gasification with CCS | yes | |
Biomass | biomass gasification | yes |
biomass gasification with CCS | yes |
</figtable>
As already mentioned in the section for :ref:`electricity`, technological change in MESSAGE is generally treated exogenously, although pioneering work on the endogenization of technological change in energy-engineering type models has been done with MESSAGE (Messner, 1997 MSG-GLB_messner_endogenized_1997). The current cost and performance parameters, including conversion efficiencies and emission coefficients is generally derived from the relevant engineering literature. For the future alternative cost and performance projections are usually developed to cover a relatively wide range of uncertainties that influences model results to a good extent. As an example, <xr id="fig:MESSAGE-GLOBIOM_costind"/> below provides an overview of costs ranges for a set of key energy conversion technologies (Fricko et al., 2016 MSG-GLB_fricko_marker_2016).
<figure id="fig:MESSAGE-GLOBIOM_costind">
</figure>
In <xr id="fig:MESSAGE-GLOBIOM_costind"/>, the black ranges show historical cost ranges for 2005. Green, blue, and red ranges show cost ranges in 2100 for SSP1, SSP2, and SSP3, respectively. Global values are represented by solid ranges. Values in the global South are represented by dashed ranges. The diamonds show the costs in the “North America” region. CCS – Carbon capture and storage; CTL – Coal to liquids; GTL – Gas to liquids; BTL – Biomass to liquids (Fricko et al., 2016 MSG-GLB_fricko_marker_2016).
4.2.4) Liquid fuels - MESSAGE-GLOBIOM
Apart from oil refining as predominant supply technology for liquid fuels at present a number of alternative liquid fuel production routes from different feedstocks are represented in MESSAGE (see <xr id="tab:MESSAGE-GLOBIOM_liqfuel"/>). Different processes for coal liquefaction, gas-to-liquids technologiesand biomass-to-liquids technologies both with and without CCS are covered. Some of these technologies include co-generation of electricity, for example, by burning unconverted syngas from a Fischer-Tropsch synthesis in a gas turbine (c.f. Larson et al., 2012 MSG-GLB_larson_chapter_2012). Technology costs for the synthetic liquid fuel production options are based on Larson et al. (2012) (MSG-GLB_larson_chapter_2012).
<figtable id="tab:MESSAGE-GLOBIOM_liqfuel">
Energy Source | Technology | Electricity cogeneration |
---|---|---|
Biomass | Fischer-Tropsch biomass-to-liquids | yes |
Fischer-Tropsch biomass-to-liquids with CCS | yes | |
Coal | Fischer-Tropsch coal-to-liquids | yes |
Fischer-Tropsch coal-to-liquids with CCS | yes | |
coal methanol-to-gasoline | yes | |
coal methanol-to-gasoline with CCS | yes | |
Gas | Fischer-Tropsch gas-to-liquids | no |
Fischer-Tropsch gas-to-liquids with CCS | no | |
Oil | simple refinery | no |
complex refinery | no |
</figtable>
4.2.6) Grid, pipelines and other infrastructure - MESSAGE-GLOBIOM
Energy transport and distribution infrastructure is included in MESSAGE at a level relevant to represent the associated costs. Within regions the capital stock of transmission and distribution infrastructure and its turnover is modeled for the following set of energy carriers:
- electricity
- district heat
- natural gas
- hydrogen
For all solid (coal, biomass) and liquid energy carriers (oil products, biofuels, fossil synfuels) a simpler approach is taken and only transmission and distribution losses and costs are taken into account.
Inter-regional energy transmission infrastructure, such as natural gas pipelines and high voltage electricity grids, are also represented between geographically adjacent regions. Solid and liquid fuel trade is, similar to the transmission and distribution within regions, modeled by taking into account distribution losses and costs. A special case are gases that can be traded in liquified form, i.e. liquified natural gas (LNG) and liquid hydrogen, where liquefaction and re-gasification infrastructure is represented in addition to the actual transport process.
Systems Integration and Reliability
The MESSAGE framework includes a single annual time period characterized by average annual load and 11 geographic regions that span the globe. Seasonal and diurnal load curves and spatial issues such as transmission constraints or renewable resource heterogeneity are treated in a stylized way in the model. The reliability extension described below elevates the stylization of temporal resolution by introducing two concepts, peak reserve capacity and general-timescale flexibility, to the model (Sullivan et al., 2013 MSG-GLB_sullivan_electric_2013). To represent capacity reserves in MESSAGE, a requirement is defined that each region build sufficient firm generating capacity to maintain reliability through reasonable load and contingency events. As a proxy for complex system reliability metrics, a reserve margin-based metric was used, setting the capacity requirement at a multiple of average load, based on electric-system parameters. While many of the same issues apply to both electricity from wind and solar energy, the description below focuses on wind.
Toward meeting the firm capacity requirement, conventional generating technologies contribute their nameplate generation capacity while variable renewables contribute a capacity value that declines as the market share of the technology increases. This reflects the fact that wind and solar generators do not always generate when needed, and that their output is generally self-correlated. In order to adjust wind capacity values for different levels of penetration, it was necessary to introduce a stepwise-linear supply curve for wind power (shown in the <xr id="fig:MESSAGE-GLOBIOM_windcap"/> below). Each bin covers a range of wind penetration levels as fraction of load and has discrete coefficients for the two constraints. The bins are predefined, and therefore are not able to allow, for example, resource diversification to increase capacity value at a given level of wind penetration.
The capacity value bins are independent of the wind supply curve bins that already existed in MESSAGE, which are based on quality of the wind resource. That supply curve is defined by absolute wind capacity built, not fraction of load; and the bins differ based on their annual average capacity factor, not capacity value. Solar PV is treated in a similar way as wind with the parameters obviously being different ones. In contrast, concentrating solar power (CSP) is modeled very much like dispatchable power plants in MESSAGE, because it is assumed to come with several hours of thermal storage, making it almost capable of running in baseload mode.
In order to ensure adequate reserve dispatch, dynamic shadow prices are placed on capacity investments of intermittent technologies (e.g., wind and solar). The prices are a function of the cumulative installed capacity of the intermittent technologies, the ability for the convential power supply to act as reserve dispatch, and the demand-side reliability requirements. For instance, a large amount of storage capacity should, all else being equal, lower the shadow price for additional wind. Conversely, an inflexible, coal- or nuclear-heavy generating base should increase the cost of investment in wind by demanding additional expenditures in the form of natural gas or storage or improved demand-side management to maintain system reliability.
Starting from the energy metric used in MESSAGE (electricity is considered as annual average load; there are no time-slices or load-curves), the flexibility requirement uses MWh of generation as its unit of note. The metric is inherently limited because operating reserves are often characterized by energy not-generated: a natural gas combustion turbine (gas-CT) that is standing by, ready to start-up at a moment’s notice; a combined-cycle plant operating below its peak output to enable ramping in the event of a surge in demand. Nevertheless, because there is generally a portion of generation associated with providing operating reserves (e.g. that on-call gas-CT plant will be called some fraction of the time), it is posited that using generated energy to gauge flexibility is a reasonable metric considering the simplifications that need to be made. Furthermore, ancillary services associated with ramping and peaking often do involve real energy generation, and variable renewable technologies generally increase the need for ramping.
Electric-sector flexibility in MESSAGE is represented as follows: each generating technology is assigned a coefficient between -1 and 1 representing (if positive) the fraction of generation from that technology that is considered to be flexible or (if negative) the additional flexible generation required for each unit of generation from that technology. Load also has a parameter (a negative one) representing the amount of flexible energy the system requires solely to meet changes and uncertainty in load. <xr id="tab:MESSAGE-GLOBIOM_flex"/> below displays the parameters that were estimated using a unit-commitment model that commits and dispatches a fixed generation system at hourly resolution to meet load an ancilliary service requirements while hewing to generator and transmission operation limitations (Sullivan et al., 2013 MSG-GLB_sullivan_electric_2013). Technologies that were not included in the unit-commitment model (nuclear, H2 electrolysis, solar PV) have estimated coefficients.
<figtable id="tab:MESSAGE-GLOBIOM_flex">
Technology | Flexibility Parameter |
---|---|
Load | -0.1 |
Wind | -0.08 |
Solar PV | -0.05 |
Geothermal | 0 |
Nuclear | 0 |
Coal | 0.15 |
Biopower | 0.3 |
Gas-CC | 0.5 |
Hydropower | 0.5 |
H2 Electrolysis | 0.5 |
Oil/Gas Steam | 1 |
Gas-CT | 1 |
Electricity Storage | 1 |
</figtable>
Thus, a technology like a simple-cycle natural gas plant, used almost exclusively for ancillary services, has a flexibility coefficient of 1, while a coal plant, which provides mostly bulk power but can supply some ancillary services, has a small, positive coefficient. Electric storage systems (e.g. pumped hydropower, compressed air storage, flow batteries) and flexible demand-side technologies like hydrogen-production contribute as well. Meanwhile, wind power and solar PV, which require additional system flexibility to smooth out fluctuations,
have negative flexibility coefficients.
4.3) Energy end-use - MESSAGE-GLOBIOM
4.3.1) Transport - MESSAGE-GLOBIOM
The most commonly applied MESSAGE transport sector representation is very stylized and essentially includes fuel switching and price-elastic demands (via MACRO linkage) as the main responses to energy and climate policy (see <xr id="fig:MESSAGE-GLOBIOM_trans"/>).
In this stylized transport sector representation fuel switching is a main option, i.e. different final energy forms that provide energy for transportation can be chosen from. In addition to the alternative energy carriers that serve as input to these stylized transportation options, their relative efficiencies are also different. The useful energy demand in the transportation sector is specified as internal combustion engine (ICE) equivalent demands which therefore by definition has a conversion efficiency of final to useful energy of 1. Relative to that the conversion efficiency of alternative fuels is higher, for example, electricity in 2010 has about a factor of three higher final to useful efficiency than the regular oil-product based ICE. The overall efficiency improvements of the ICE in the transportation sector and modal switching over time is implicitly included in the demand specifications, coming from the scenario generator (see section on Energy demand of MESSAGE-GLOBIOM). Additional demand reduction in response to price increases in policy scenarios then occurs via the fuel switching option (due to the fuel-specific relative efficiencies) as well as via the linkage with the macro-economic model MACRO as illustrated in <xr id="fig:MESSAGE-GLOBIOM_trans"/> below.
Limitations of switching to alternative fuels may occur for example as a result of restricted infrastructure availability (e.g., rail network) or some energy carriers being unsuitable for certain transport modes (e.g., electrification of aviation). To reflect these limitations, share constraints of energy carriers (e.g., electricity) and energy carrier groups (e.g., liquid fuels) are used in the transport sector. In addition, the diffusion of speed of alternative fuels is limited to mimic bottlenecks in the supply chains, not explicitly represented in MESSAGE (e.g., non-energy related infrastructure). Both the share as well as the diffusion constraints are usually parametrized based on transport sector studies that analyze such developments and their feasibility in much greater detail.
<figure id="fig:MESSAGE-GLOBIOM_trans">
</figure>
The demand for international shipping is modeled in a simplified way with a number of different energy carrier options (light and heavy fuel oil, biofuels, natural gas, and hydrogen). The demand for international shipping is coupled to global GDP development with an income elasticity, but to date no demand response in mitigation scenarios is implemented.
<xr id="tab:MESSAGE-GLOBIOM_trans"/> presents the quantitative translation of the the storyline elements of SSP1, SSP2 and SSP3 in terms of electrification rate for transport (Fricko et al., 2016 MSG-GLB_fricko_marker_2016).
<figtable id="tab:MESSAGE-GLOBIOM_trans">
SSP1 | SSP2 | SSP3 | |
---|---|---|---|
Transport | High electrification (max. 75% of total transport possible) | Medium electrification (max. 50% of total transport possible) | Low electrification (max 10% of total transport possible) |
</figtable>
4.3.2) Residential and commercial sectors - MESSAGE-GLOBIOM
The residential and commercial sector in MESSAGE distinguishes two demand categories, thermal and specific. Thermal demand, i.e. low temperature heat, can be supplied by a variety of different energy carriers while specific demand requires electricity (or a decentralized technology to convert other energy carriers to electricity).
This stylized residential and commercial thermal energy demand includes fuel switching as the main option, i.e. different choices about final energy forms to provide thermal energy. In addition to the alternative energy carriers that serve as input to these thermal energy supply options, their relative efficiencies also vary. For example, solid fuels such as coal have lower conversion efficiencies than natural gas, direct electric heating or electric heat pumps. Additional demand reduction in response to price increases in policy scenarios is included via the fuel switching option (due to the fuel-specific relative efficiencies) as well as via the linkage with the macro-economic model MACRO (see <xr id="fig:MESSAGE-GLOBIOM_rescom"/> below). The specific residential and commercial demand can be satisfied either by electricity from the grid or with decentralized electricity generation options such as fuel cells or CHP.
<figure id="fig:MESSAGE-GLOBIOM_rescom">
</figure>
To reflect limitations of switching to alternative fuels, for example as a result of limited infrastructure availability (e.g., district heating network) or some energy carriers being unsuitable for certain applications, share constraints of energy carriers (e.g., electricity) and energy carrier groups (e.g., liquid fuels) are used in the residential and commercial sector. In addition, the diffusion of speed of alternative fuels is limited to mimic bottlenecks in the supply chains, not explicitly represented in MESSAGE (e.g., non-energy related infrastructure).
<xr id="tab:MESSAGE-GLOBIOM_rescom"/> presents the quantitative translation of the the storyline elements of SSP1, SSP2 and SSP3 in terms of electrification rate for the residential and commercial sectors. These indicators apply to 2010-2100; Intensity improvements are in FE/GDP annually (Fricko et al., 2016 MSG-GLB_fricko_marker_2016).
<figtable id="tab:MESSAGE-GLOBIOM_rescom">
SSP1 | SSP2 | SSP3 | |
---|---|---|---|
Residential & Commercial | High electrification rate:1.44% (Regional range from 0.35% to 4%) | Medium electrification rate: 1.07% (Regional range from 0.23% to 3%) | Low electrification rate: 0.87% (Regional range from 0.37% to 2%) |
</figtable>
4.3.3) Industrial sector - MESSAGE-GLOBIOM
The industrial sector in MESSAGE distinguishes two demand categories, thermal and specific. Thermal demand, i.e. heat at different temperature levels, can be supplied by a variety of different energy carriers while specific demand requires electricity (or a decentralized technology to convert other energy carriers to electricity).
This stylized industrial thermal energy demand includes fuel switching as the main option, i.e. different final energy forms that provide energy for thermal energy can be chosen from. In addition to the alternative energy carriers that serve as input to these thermal energy supply options, their relative efficiencies also vary. For example, solid fuels such as coal have lower conversion efficiencies than natural gas, direct electric heating or electric heat pumps. To account for the fact that some technologies cannot supply temperature at high temperature levels (e.g., electric heat pumps, district heat), the share of these technologies in the provision of industrial thermal demand is constrained. Additional demand reduction in response to price increases in policy scenarios is included via the fuel switching option (due to the fuel-specific relative efficiencies) as well as via the linkage with the macro-economic model MACRO (see <xr id="fig:MESSAGE-GLOBIOM_indus"/> below). The specific industrial demand can be satisfied either by electricity from the grid or with decentralized electricity generation options (including CHP) such as fuel cells.
<figure id="fig:MESSAGE-GLOBIOM_indus">
</figure>
While cement production is not explicitly modeled at the process level in MESSAGE, the amount of cement of cement production is linked to industrial activity (more specifically the industrial thermal demand in MESSAGE) and the associated CO2 emissions from the calcination process are accounted for explicitly. In addition, adding carbon capture and storage to mitigate these process-based CO2 emission is available.
<xr id="tab:MESSAGE-GLOBIOM_indus"/> presents the quantitative translation of the the storyline elements of SSP1, SSP2 and SSP3 in terms of electrification rate for industry and feedstocks. These indicators apply to 2010-2100; Intensity improvements are in FE/GDP annually (Fricko et al., 2016 MSG-GLB_fricko_marker_2016).
<figtable id="tab:MESSAGE-GLOBIOM_indus">
SSP1 | SSP2 | SSP3 | |
---|---|---|---|
Industry and feedstocks | High electrification rate: 0.56% (Regional range from 0.2% to 1.2%)
High feedstock reduction rate: -0.33% (Regional range from -0.51 to 0.59%) |
Medium electrification rate: 0.47% (Regional range from 0.07% to 1.08%)
Medium feedstock reduction rate: -0.27% (Regional range from -0.45% to 0.64%) |
Low electrification rate: 0.12% (Regional range from -0.03% to 0.71%)
Low feedstock reduction rate: -0.24% (Regional range from -0.38% to 0.51%) |
4.4) Energy demand - MESSAGE-GLOBIOM
Baseline energy service demands are provided exogenously to MESSAGE, though they can be adjusted endogenously based on energy prices using the MESSAGE-MACRO link. There are seven energy service demands that are provided to MESSAGE, including:
- Residential/commercial thermal
- Residential/commercial specific
- Industrial thermal
- Industrial specific
- Industrial feedstock (non-energy)
- Transportation
- Non-commercial biomass.
These demands are generated using a so-called scenario generator which is implemented in the script language [[1]]. The scenario generator uses country-level historical data of GDP per capita (PPP) and final energy use as well as projections of GDP (PPP) and population to extrapolate the seven energy service demands into the future. The sources for the historical and projected datasets are the following:
- Historical GDP (PPP) – World Bank (World Development Indicators 2012 MSG-GLB_world_bank_group_world_2012)
- Historical Population – UN Population Division (World Population Projection 2010 MSG-GLB_un_population_division_world_2010)
- Historical Final Energy – International Energy Agency Energy Balances (IEA 2012 MSG-GLB_international_energy_agency_energy_2012)
- Projected GDP (PPP) – Dellink et al (2015 MSG-GLB_dellink_long-term_2015), see Shared Socio-Economic Pathways database (scenarios)
- Projected Population – KC and Lutz (2014 MSG-GLB_kc_human_2014), see Shared Socio-Economic Pathways database(scenarios)
The scenario generator runs regressions on the historical datasets to establish the relationship between the independent variable (GDP (PPP) per capita) and several dependent variables, including total final energy intensity (MJ/2005USD) and the shares of final energy in several energy sectors (%). In the case of final energy intensity, the relationship is best modeled by a power function so both variables are log-transformed. In the case of most sectoral shares, only the independent variable is log-transformed. The exception is the industrial share of final energy, which uses a hump-shaped function inspired by Schäfer (2005) MSG-GLB_schafer_structural_2005. This portion of the model provides the historical relationships between GDP per capita and the dependent variables for each of the eleven MESSAGE regions.
The historical data are also used in regressions to develop global trend lines that represent each percentile of the cumulative distribution function (CDF) of each regressed variable. Given the regional regressions and global trend lines, final energy intensity and sectoral shares can be extrapolated based on projected GDP per capita, or average income. Several user-defined inputs allow the user to tailor the extrapolations to individual socio-economic scenarios. In the case of final energy intensity (FEI), the extrapolation is produced for each region by defining the quantile at which FEI converges (e.g., the 20th percentile) and the income at which the convergence occurs. For example, while final energy intensity converges quickly to the lowest quantile (0.001) in SSP1, it converges more slowly to a larger quantile (0.5 to 0.7 depending on the region) in SSP3. Convergence quantiles and incomes are provided for each SSP and region in <xr id="tab:MESSAGE-GLOBIOM_quantssp1"/>, <xr id="tab:MESSAGE-GLOBIOM_quantssp2"/> and <xr id="tab:MESSAGE-GLOBIOM_quantssp3"/>. The convergence quantile allows one to identify the magnitude of FEI while the convergence income establishes the rate at which the quantile is approached. For the sectoral shares, the user can specify the global quantile at which the extrapolation should converge, the income at which the extrapolation diverges from the regional regression line and turns parallel to the specified convergence quantile (i.e., how long the sectoral share follows the historical trajectory), and the income at which the extrapolation converges to the quantile. Given these input parameters, the user can extrapolate both FEI and sectoral shares.
The total final energy in each region is then calculated by multiplying the extrapolated final energy intensity by the projected GDP (PPP) in each time period. Next, the extrapolated shares are multiplied by the total final energy to identify final energy demand for each of the seven energy service demands used in MESSAGE. Finally, final energy is converted to useful energy in each region by using the average final-to-useful energy efficiencies reported by the IEA for each country.
<figtable id="tab:MESSAGE-GLOBIOM_quantssp1">
SSP1 | AFR | CPA | EEU | FSU | LAM | MEA | NAM | PAO | PAS | SAS | WEU |
---|---|---|---|---|---|---|---|---|---|---|---|
Convergence Quantile | |||||||||||
Final Energy Intensity (FEI) | 0.001 | 0.001 | 0.001 | 0.001 | 0.001 | 0.001 | 0.001 | 0.001 | 0.001 | 0.001 | 0.001 |
Share NC Biomass | 0.01 | 0.25 | 0.01 | 0.75 | 0.01 | 0.3 | 0.01 | 0.01 | 0.01 | 0.01 | 0.01 |
Share Transport | 0.05 | 0.02 | 0.2 | 0.05 | 0.2 | 0.05 | 0.2 | 0.2 | 0.04 | 0.03 | 0.2 |
Share Res/Com | 0.25 | 0.25 | 0.2 | 0.2 | 0.28 | 0.3 | 0.25 | 0.2 | 0.28 | 0.3 | 0.2 |
Share Industry | 0.1 | 0.2 | 0.1 | 0.5 | 0.28 | 0.2 | 0.3 | 0.3 | 0.28 | 0.2 | 0.3 |
Elec Share Res/Com | 0.45 | 0.45 | 0.45 | 0.45 | 0.63 | 0.62 | 0.4 | 0.63 | 0.62 | 0.64 | 0.43 |
Feedstock Share Industry | 0.18 | 0.2 | 0.24 | 0.24 | 0.2 | 0.26 | 0.26 | 0.23 | 0.26 | 0.22 | 0.24 |
Elec Share Industry | 0.4 | 0.4 | 0.42 | 0.36 | 0.4 | 0.33 | 0.36 | 0.36 | 0.4 | 0.4 | 0.4 |
Convergence Income | |||||||||||
Final Energy Intensity (FEI) | 112295 | 98603 | 299177 | 112307 | 100188 | 113404 | 112356 | 112261 | 106323 | 112300 | 107636 |
Share NC Biomass | 5981 | 46015 | 34405 | 40951 | 20038 | 34894 | 112356 | 112261 | 16357 | 11105 | 48153 |
Share Transport | 99676 | 32868 | 112341 | 71664 | 112310 | 113404 | 123018 | 94337 | 112293 | 97169 | 141627 |
Share Res/Com | 119611 | 112276 | 179506 | 153565 | 112310 | 112270 | 123018 | 157229 | 112293 | 112300 | 141627 |
Share Industry | 39870 | 105177 | 164547 | 92139 | 40075 | 112270 | 123018 | 112261 | 126769 | 83288 | 127464 |
Elec Share Res/Com | 112295 | 112276 | 112341 | 112307 | 112310 | 87234 | 131219 | 132072 | 112293 | 112300 | 112168 |
Feedstock Share Industry | 112295 | 112276 | 112341 | 112307 | 112310 | 112270 | 123018 | 125783 | 112293 | 112300 | 112168 |
Elec Share Industry | 112295 | 98603 | 299177 | 112307 | 100188 | 113404 | 112356 | 112261 | 106323 | 112300 | 107636 |
</figtable>
<figtable id="tab:MESSAGE-GLOBIOM_quantssp2">
SSP2 | AFR | CPA | EEU | FSU | LAM | MEA | NAM | PAO | PAS | SAS | WEU |
---|---|---|---|---|---|---|---|---|---|---|---|
Convergence Quantile | |||||||||||
Final Energy Intensity (FEI) | 0.03 | 0.03 | 0.03 | 0.04 | 0.04 | 0.04 | 0.05 | 0.02 | 0.03 | 0.03 | 0.02 |
Share NC Biomass | 0.6 | 0.6 | 0.75 | 0.75 | 0.25 | 0.75 | 0.75 | 0.75 | 0.6 | 0.6 | 0.75 |
Share Transport | 0.05 | 0.04 | 0.15 | 0.1 | 0.5 | 0.3 | 0.5 | 0.14 | 0.2 | 0.05 | 0.15 |
Share Res/Com | 0.15 | 0.28 | 0.5 | 0.5 | 0.3 | 0.5 | 0.3 | 0.35 | 0.3 | 0.28 | 0.33 |
Share Industry | 0.25 | 0.4 | 0.15 | 0.25 | 0.15 | 0.25 | 0.25 | 0.25 | 0.25 | 0.6 | 0.25 |
Elec Share Res/Com | 0.42 | 0.4 | 0.35 | 0.22 | 0.58 | 0.6 | 0.14 | 0.57 | 0.6 | 0.51 | 0.18 |
Feedstock Share Industry | 0.15 | 0.22 | 0.26 | 0.26 | 0.18 | 0.27 | 0.32 | 0.27 | 0.3 | 0.22 | 0.27 |
Elec Share Industry | 0.39 | 0.38 | 0.4 | 0.45 | 0.35 | 0.4 | 0.4 | 0.4 | 0.4 | 0.43 | 0.35 |
Convergence Income | |||||||||||
Final Energy Intensity (FEI) | 200009 | 200033 | 299177 | 266179 | 199975 | 139574 | 246036 | 141506 | 199968 | 200002 | 199977 |
Share NC Biomass | 19935 | 26294 | 77786 | 40951 | 20038 | 94649 | 94724 | 132072 | 12268 | 18046 | 48153 |
Share Transport | 49838 | 105177 | 94540 | 94596 | 80150 | 94649 | 94724 | 94652 | 81787 | 27763 | 99139 |
Share Res/Com | 119611 | 65735 | 89753 | 71664 | 94577 | 69787 | 94724 | 110060 | 81787 | 83288 | 113301 |
Share Industry | 31896 | 105177 | 44877 | 102377 | 100188 | 78511 | 94724 | 141506 | 98144 | 13881 | 94607 |
Elec Share Res/Com | 69773 | 94593 | 94540 | 102377 | 94577 | 87234 | 123018 | 141506 | 94627 | 55525 | 113301 |
Feedstock Share Industry | 19935 | 94593 | 94540 | 94596 | 94577 | 94649 | 94724 | 94652 | 94627 | 94615 | 94607 |
Elec Share Industry | 200009 | 200033 | 299177 | 266179 | 199975 | 139574 | 246036 | 141506 | 199968 | 200002 | 199977 |
</figtable>
<figtable id="tab:MESSAGE-GLOBIOM_quantssp3">
SSP2 | AFR | CPA | EEU | FSU | LAM | MEA | NAM | PAO | PAS | SAS | WEU |
---|---|---|---|---|---|---|---|---|---|---|---|
Convergence Quantile | |||||||||||
Final Energy Intensity (FEI) | 0.6 | 0.55 | 0.5 | 0.7 | 0.7 | 0.5 | 0.7 | 0.5 | 0.5 | 0.7 | 0.6 |
Share NC Biomass | 0.9 | 0.6 | 0.75 | 0.75 | 0.25 | 0.75 | 0.75 | 0.75 | 0.6 | 0.9 | 0.75 |
Share Transport | 0.1 | 0.05 | 0.7 | 0.2 | 0.45 | 0.5 | 0.7 | 0.25 | 0.5 | 0.1 | 0.7 |
Share Res/Com | 0.25 | 0.25 | 0.55 | 0.55 | 0.3 | 0.5 | 0.35 | 0.6 | 0.25 | 0.2 | 0.5 |
Share Industry | 0.1 | 0.6 | 0.2 | 0.1 | 0.2 | 0.2 | 0.1 | 0.1 | 0.6 | 0.2 | 0.1 |
Elec Share Res/Com | 0.4 | 0.6 | 0.45 | 0.4 | 0.9 | 0.9 | 0.25 | 0.65 | 0.9 | 0.6 | 0.33 |
Feedstock Share Industry | 0.2 | 0.22 | 0.26 | 0.24 | 0.2 | 0.3 | 0.32 | 0.29 | 0.3 | 0.22 | 0.27 |
Elec Share Industry | 0.3 | 0.43 | 0.37 | 0.45 | 0.3 | 0.4 | 0.35 | 0.45 | 0.4 | 0.35 | 0.4 |
Convergence Income | |||||||||||
Final Energy Intensity (FEI) | 200009 | 200033 | 200000 | 200044 | 199975 | 200027 | 200109 | 199995 | 199968 | 200002 | 199977 |
Share NC Biomass | 13955 | 26294 | 80927 | 40951 | 12023 | 80953 | 80782 | 132072 | 12268 | 12771 | 48153 |
Share Transport | 13955 | 46015 | 59835 | 51188 | 70131 | 69787 | 80782 | 132072 | 32715 | 55525 | 81010 |
Share Res/Com | 23922 | 65735 | 59835 | 61426 | 80952 | 52340 | 80782 | 80816 | 199968 | 80512 | 81010 |
Share Industry | 5981 | 52588 | 200000 | 122852 | 18034 | 43617 | 200109 | 199995 | 81787 | 30539 | 198277 |
Elec Share Res/Com | 80976 | 80986 | 80927 | 61426 | 80952 | 69787 | 80782 | 80816 | 80969 | 80956 | 81010 |
Feedstock Share Industry | 19935 | 26294 | 80927 | 80980 | 80952 | 80953 | 80782 | 80816 | 80969 | 80956 | 81010 |
Elec Share Industry | 200009 | 200033 | 200000 | 200044 | 199975 | 200027 | 200109 | 199995 | 199968 | 200002 | 199977 |
</figtable>
4.5) Technological change in energy - MESSAGE-GLOBIOM
Technological change in MESSAGE is generally treated exogenously, although pioneering work on the endogenization of technological change via learning curves in energy-engineering type models (Messner, 1997 MSG-GLB_messner_endogenized_1997) and the dependence of technology costs on market structure has been done with MESSAGE (Leibowicz, 2015 MSG-GLB_leibowicz_growth_2015). The current cost and performance parameters, including conversion efficiencies and emission coefficients is generally derived from the relevant engineering literature. For the future alternative cost and performance projections are developed to cover a relatively wide range of uncertainties that influences model results to a good extent.
Technology cost
The quantitative assumptions about technology cost development are derived from the overarching qualitative SSP narratives (cf. section narratives). In SSP1, for instance, whose green-growth storyline is more consistent with a sustainable development paradigm, higher rates of technological progress and learning are assumed for renewables and other advanced technologies that may replace fossil fuels (e.g., the potential for electric mobility is assumed to be higher in SSP1 compared to SSP2 or SSP3). In contrast, SSP3 assumes limited progress across a host of advanced technologies, particularly for renewables and hydrogen; more optimistic assumptions are instead made for coal-based technologies, not only for power generation but also for liquid fuels production. Meanwhile, the middle-of-the-road SSP2 narrative is characterized by a fairly balanced view of progress for both conventional fossil and non-fossil technologies. In this sense, technological development in SSP2 is not biased toward any particular technology group.
Technological costs vary regionally in all SSPs, reflecting marked differences in engineering and construction costs across countries observed in the real world. Generally, costs start out lower in the developing world and are assumed to converge to those of present-day industrialized countries as the former becomes richer throughout the century (thus, the cost projections consider both labour and capital components). This catch-up in costs is assumed to be fastest in SSP1 and slowest in SSP3 (where differences remain, even in 2100); SSP2 is in between. Estimates for present-day and fully learned-out technology costs are from the Global Energy Assessment (Riahi et al., 2012 MSG-GLB_riahi_chapter_2012) and World Energy Outlook (IEA, 2014 MSG-GLB_international_energy_agency_world_2014). A summary of these cost assumptions can be found in sections Electricity of MESSAGE-GLOBIOM and Other conversion of MESSAGE-GLOBIOM.
Technology diffusion
MESSAGE tracks investments by vintage, an important feature to represent the inertia in the energy system due to its long-lived capital stock. In case of shocks (e.g., introduction of stringent climate policy), it is however possible to prematurely retire existing capital stock such as power plants or other energy conversion technologies and switch to more suitable alternatives.
An important factor in this context that influences technology adoption in MESSAGE are technology diffusion constraints. Technology diffusion in MESSAGE is determined by dynamic constraints that relate the construction of a technology added or the activity (level of production) of a technology in a period t to construction or the activity in the previous period t-1 (Messner and Strubegger, 1995 MSG-GLB_messner_users_1995 cf. section :ref:`upper_dynamic_constraint_capacity).
While limiting the possibility of flip-flop behavior as is frequently observed in unconstrained Linear Programming (LP) models such as MESSAGE, a drawback of such hard growth constraints is that the relative advantage of some technology over another technology is not taken into account and therefore even for very competitive technologies, no acceleration of technology diffusion is possible. In response to this limitation, so called flexible or soft dynamic constraints have been introduced into MESSAGE (Keppo and Strubegger, 2010 MSG-GLB_keppo_short_2010). These allow faster technology diffusion at additional costs and therefore generate additional model flexibility while still reducing the flip-flop behavior and sudden penetration of technologies.
<xr id="fig:MESSAGE-GLOBIOM_difconstraint"/> below illustrates the maximum technology growth starting at a level of 1 in year t=0 for a set of five diffusion constraints which jointly lead to a soft constraint.
<figure id="fig:MESSAGE-GLOBIOM_difconstraint">
</figure>
For a more detailed description of the implementation of technology diffusion constraints, see the Annex Section ref:annex_convtech.
5) Land-use - MESSAGE-GLOBIOM
Land-use dynamics are modelled with the GLOBIOM (GLobal BIOsphere Management) model, which is a recursive-dynamic partial-equilibrium model (Havlík et al., 2011 MSG-GLB_havlik_global_2011; Havlík et al., 2014 MSG-GLB_havlik_climate_2014). GLOBIOM represents the competition between different land-use based activities. It includes a bottom-up representation of the agricultural, forestry and bio-energy sector, which allows for the inclusion of detailed grid-cell information on biophysical constraints and technological costs, as well as a rich set of environmental parameters, incl. comprehensive AFOLU (agriculture, forestry and other land use) GHG emission accounts and irrigation water use. Its spatial equilibrium modelling approach represents bilateral trade based on cost competitiveness. For spatially explicit projections of the change in afforestation, deforestation, forest management, and their related CO2 emissions, GLOBIOM is coupled with the G4M (Global FORest Model) model (Kindermann et al., 2006 MSG-GLB_kindermann_predicting_2006; Kindermann et al., 2008 MSG-GLB_kindermann_global_2008; Gusti, 2010 MSG-GLB_gusti_algorithm_2010). The spatially explicit G4M model compares the income of managed forest (difference of wood price and harvesting costs, income by storing carbon in forests) with income by alternative land use on the same place, and decides on afforestation, deforestation or alternative management options. As outputs, G4M provides estimates of forest area change, carbon uptake and release by forests, and supply of biomass for bioenergy and timber. (Fricko et al., 2016 MSG-GLB_fricko_marker_2016)
As a partial equilibrium model representing land-use based activities, including agriculture, forestry and bioenergy sectors, GLOBIOM is built following a bottom-up setting based on detailed gridcell information, providing the biophysical and technical cost information. Production adjusts to meet the demand at the level of 30 economic regions. International trade representation is based on the spatial equilibrium modelling approach, where individual regions trade with each other based purely on cost competitiveness because goods are assumed to be homogenous (Takayama and Judge 1971 MSG-GLB_takayama_spatial_1971; Schneider, McCarl et al. 2007 MSG-GLB_schneider_agricultural_2007). Market equilibrium is determined through mathematical optimization which allocates land and other resources to maximize the sum of consumer and producer surplus (McCarl and Spreen 1980 MSG-GLB_mccarl_surplus_1980). As in other partial equilibrium models, prices are endogenous. The model is run recursively dynamic with a 10 year time step, along a baseline going from 2000 to 2100. The model is solved using a linear programming simplex solver and can be run on a personal computer with the GAMS software.
For more information about the land-use part of MESSAGE-GLOBIOM, please visit IIASA's MESSAGE-GLOBIOM documentation.
6) Emissions - MESSAGE-GLOBIOM
In the sub-sections of this chapter, the GHG and non-GHG emissions included in MESSAGE-GLOBIOM are presented.
6.1) GHGs - MESSAGE-GLOBIOM
Carbon-dioxide (CO2)
The MESSAGE model includes a detailed representation of energy-related and - via the link to GLOBIOM - land-use CO2 emissions (Riahi and Roehrl, 2000 MSG-GLB_riahi_greenhouse_2000; Riahi, Rubin et al., 2004 MSG-GLB_riahi_prospects_2004; Rao and Riahi, 2006 MSG-GLB_rao_role_2006; Riahi et al., 2011 MSG-GLB_riahi_rcp_2011). CO2 emission factors of fossil fuels and biomass are based on the 1996 version of the IPCC guidelines for national greenhouse gas inventories MSG-GLB_ipcc_revised_1996 (see <xr id="tab:MESSAGE-GLOBIOM_emissionfactor"/>). It is important to note that biomass is generally treated as being carbon neutral in the energy system, because the effects on the terrestrial carbon stocks are accounted for on the land use side, i.e. in GLOBIOM (see section Land-Use of MESSAGE-GLOBIOM). The CO2 emission factor of biomass is, however, relevant in the application of carbon capture and storage (CCS) where the carbon content of the fuel and the capture efficiency of the applied process determine the amount of carbon captured per unit of energy.
<figtable id="tab:MESSAGE-GLOBIOM_emissionfactor">
Fuel | Emission factor [tC/TJ] | Emission factor [tCO2/TJ] | Emission factor [tC/kWyr] |
---|---|---|---|
Hard coal | 25.8 | 94.6 | 0.814 |
Lignite | 27.6 | 101.2 | 0.870 |
Crude oil | 20.0 | 73.3 | 0.631 |
Light fuel oil | 20.0 | 73.3 | 0.631 |
Heavy fuel oil | 21.1 | 77.4 | 0.665 |
Methanol | 17.4 | 63.8 | 0.549 |
Natural gas | 15.3 | 56.1 | 0.482 |
Solid biomass | 29.9 | 109.6 | 0.942 |
</figtable>
CO2 emissions of fossil fuels for the entire energy system are accounted for at the resource extraction level by applying the CO2 emission factors listed in <xr id="tab:MESSAGE-GLOBIOM_emissionfactor"/> to the extracted fossil fuel quantities. In this economy-wide accounting, carbon emissions captured in CCS processes remove carbon from the balance equation, i.e. they contribute with a negative emission coefficient. In parallel, a sectoral accounting of CO2 emissions is performed which applies the same emission factors to fossil fuels used in individual conversion processes. In addition to conversion processes, also CO2 emissions from energy use in fossil fuel resource extraction are explicitly accounted for. An important feature of MESSAGE in this context is that CO2 emissions from the extraction process increase when moving from conventional to unconventional fossil fuel resources.
CO2 mitigation options in the energy system include technology and fuel shifts; efficiency improvements; and CCS. A large number of specific mitigation technologies are modeled bottom-up in MESSAGE with a dynamic representation of costs and efficiencies. As mentioned above, MESSAGE also includes a detailed representation of carbon capture and sequestration from both fossil fuel and biomass combustion.
Non-CO2 GHGs
MESSAGE includes a representation of non-CO2 GHGs (CH4, N2O, HFCs, SF6, PFCs) mandated by the Kyoto Protocol (Rao and Riahi, 2006 MSG-GLB_rao_role_2006) with the exception of NF3. Included is a representation of emissions and mitigation options from both energy related processes as well as non-energy sources like municipal solid waste disposal and wastewater. CH4 and N2O emissions from land are taken care of by the link to GLOBIOM.
6.2) Pollutants and non-GHG forcing agents - MESSAGE-GLOBIOM
Air pollution implications are derived with the help of the GAINS (Greenhouse gas–Air pollution INteractions and Synergies) model. GAINS allows for the development of cost-effective emission control strategies to meet environmental objectives on climate, human health and ecosystem impacts until 2030 (Amann et al., 2011 MSG-GLB_amann_cost-effective_2011). These impacts are considered in a multi-pollutant context, quantifying the contributions of sulfur dioxide (SO2), nitrogen oxides (NOx), ammonia (NH3), non-methane volatile organic compounds (VOC), and primary emissions of particulate matter (PM), including fine and coarse PM as well as carbonaceous particles (BC, OC). As a stand-alone model, it also tracks emissions of six greenhouse gases of the Kyoto basket with exception of NF3. The GAINS model has global coverage and holds essential information about key sources of emissions, environmental policies, and further mitigation opportunities for about 170 country-regions. The model relies on exogenous projections of energy use, industrial production, and agricultural activity for which it distinguishes all key emission sources and several hundred control measures. GAINS can develop finely resolved mid-term air pollutant emission trajectories with different levels of mitigation ambition (Cofala et al., 2007 MSG-GLB_cofala_scenarios_2007; Amann et al., 2013 MSG-GLB_amann_regional_2013). The results of such scenarios are used as input to global IAM frameworks to characterize air pollution trajectories associated with various long-term energy developments (see further for example Riahi et al., 2012 MSG-GLB_riahi_chapter_2012; Rao et al., 2013 MSG-GLB_rao_better_2013; Fricko et al., 2016 MSG-GLB_fricko_marker_2016).
7) Climate - MESSAGE-GLOBIOM
The response of the carbon-cycle and climate to anthropogenic climate drivers is modelled with the MAGICC model (Model for the Assessment of Greenhouse-gas Induced Climate Change). MAGICC is a reduced-complexity coupled global climate and carbon cycle model which calculates projections for atmospheric concentrations of GHGs and other atmospheric climate drivers like air pollutants, together with consistent projections of radiative forcing, global annual-mean surface air temperature, and ocean-heat uptake (Meinshausen et al., 2011a MSG-GLB_meinshausen_emulating_2011). MAGICC is an upwelling-diffusion, energy-balance model, which produces outputs for global- and hemispheric-mean temperature. MAGICC is most commonly used in a deterministic setup (Meinshausen et al., 2011b MSG-GLB_meinshausen_rcp_2011), but also a probabilistic setup (Meinshausen et al., 2009 MSG-GLB_meinshausen_greenhouse-gas_2009) is available which allows to estimate the probabilities of limiting warming to below specific temperature levels given a specified emissions path (Rogelj et al., 2013a MSG-GLB_rogelj_2020_2013; Rogelj et al., 2013b MSG-GLB_rogelj_probabilistic_2013; Rogelj et al., 2015 MSG-GLB_rogelj_mitigation_2015). Climate feedbacks on the global carbon cycle are accounted for through the interactive coupling of the climate model and a range of gas-cycle models. (Fricko et al., 2016 MSG-GLB_fricko_marker_2016)
For more information about the model, see www.magicc.org.
7.1) Modelling of climate indicators - MESSAGE-GLOBIOM
8) Non-climate sustainability dimension - MESSAGE-GLOBIOM
Even other non-climate sustainable development dimensions have been modeled with the MESSAGE-GLOBIOM model framework. These include air pollution, water use in the energy sector and energy access. These are presented in the subsections of this chapter.
8.1) Air pollution and health - MESSAGE-GLOBIOM
The different Shared Socioeconomic Pathways (SSPs) have varying impacts on air pollution emissions. SSP1 and SSP5 show the most rapid emissions reductions than the other SSPs due to more effective pollution control and lower intensity for fossil fuels. SSP3 shows a consistent decline throughout the century, which is however less sharp than the reduction presented by SSP1 and SSP5. SSP3, due to larger projected population growth and relatively more slow and heterogeneous economic growth, results in an increase in emissions until 2030, and through a slight post-2030 decline end in only slightly lower emissions levels than the current ones by 2100.
Mitigation scenarios bring co-benefits in terms of air pollutant emission reductions. The largest emissions reductions can be seen for the SSP3 scenario, which has the highest baseline emissions, and the lowest for SSP1/SSP5. In terms of pollutants, SO2 and NOx emissions result in the largest reductions, whereas BC emissions do not decline as much - this can mainly be attributed to assumptions on fuel-substitution in the residential sector. (Rao et al, 2016MSG-GLB_rao_future_2016)
<xr id="fig:MESSAGE-GLOBIOM_AP_SSP"/> presents the differences of emissions reductions between the different SSPs for both a reference case as well as for mitigation scenarios.
<figure id="fig:MESSAGE-GLOBIOM_AP_SSP">
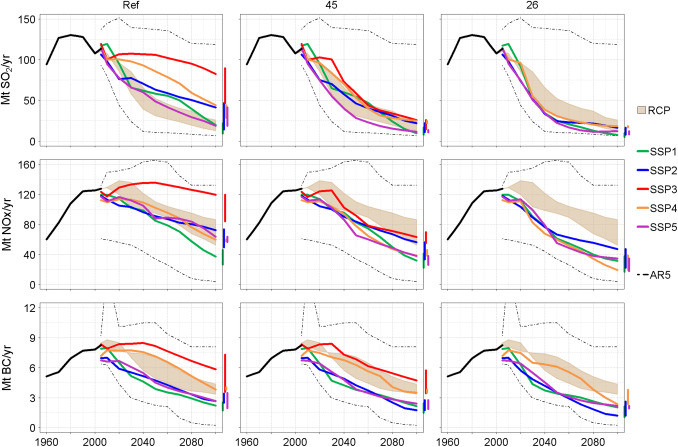
</figure>
In terms of regional air pollution impacts of the different SSPs, the strong air pollution control scenarios (SSP1/SSP5) show significantly lower concentrations across all regions than the less stringent air pollution control scenarios (SSP3/SSP4). OECD countries are expected to enhance their air pollution situation by 2050 under all SSP scenarios. For Middle East and Africa, mineral dust is responsible for most of the higher concentration levels, and therefore, in this region, mitigation measures will not be as effective as elsewhere. For Asia the low air pollution control scenarios (SSP3/SSP4) would increase the amount of people exposed to high levels of air pollutants - however, mitigation measures have the potential for significant co-benefits in terms of air pollutants for the region. <xr id="fig:MESSAGE-GLOBIOM_AP_SSPreg"/> illustrates these regional impacts across the SSPs. (Rao et al, 2016MSG-GLB_rao_future_2016)
<figure id="fig:MESSAGE-GLOBIOM_AP_SSPreg">
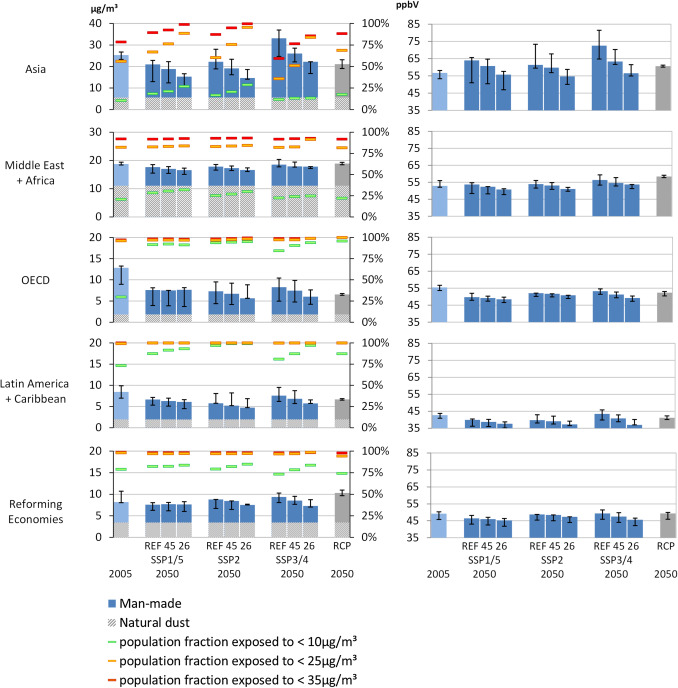
</figure>
8.2) Water - MESSAGE-GLOBIOM
Large amounts of water are currently being used in the energy sector. For assessing long-term freshwater sustainability, MESSAGE has been adapted to quantify the impact of energy system transformations on water.
The majority of energy sector freshwater withdrawal occurs in the steam-cycle and cooling systems related to thermoelectric power plants. The model distinguishes between two different water-cooling technologies, but also a technology only using air for cooling purposes, which provide an opportunity to reduce energy system reliance on water. The different technologies that are therefore distinguished in the model are:
- Once-through cooling technology: water is passed through the cooling system once and then returned to its source.
- Closed-loop cooling technology: water that is withdrawn is re-circulated.
- Air-cooling technology: instead of water, air is used for cooling purposes.
Further, the water source is distinguished across technologies between fresh or saline.
This choice of model formulation enables consistent representation of water use across power plant types and incorporates water impacts of heat-rate improvements due to anticipated long-term technological change. Moreover, the approach enables analysis of thermal water pollution from once-through cooled thermal power plants by allowing quantification of the heat energy embodied in cooling system effluents.
When applied to a broad range of climate mitigation scenarios that aim for 2 degrees Celsius, the results show a wide range of water implications across scenarios. Global demand of freshwater is expected to grow in all 2 degree scenarios due to rapidly growing electricity demand in many developing countries as a result of and the prevalence of freshwater-cooled thermal power generation. However, a shift to water-efficient cooling technologies can significantly reduce the use of water within the energy sector by reducing the freshwater withdrawals and thermal pollution related to thermoelectric power production. Further, controlling demand is another strategy that can reduce the water use, and further, it provides more flexibility in terms of cooling technology choices for thermoelectric power plants. Therefore, an integrated approach, using both technology adaptation and demand control, is seen as the most effective and flexible approach to reducing water demand in the energy sector. (Fricko et al, 2016MSG-GLB_fricko_energy_2016)
<xr id="fig:MESSAGE-GLOBIOM_water1"/> presents the impact of water usage across different 2 degree scenarios from the Global Energy Assessment (GEA), and how adopting technologies can help reduce the water demand.
<figure id="fig:MESSAGE-GLOBIOM_water1">
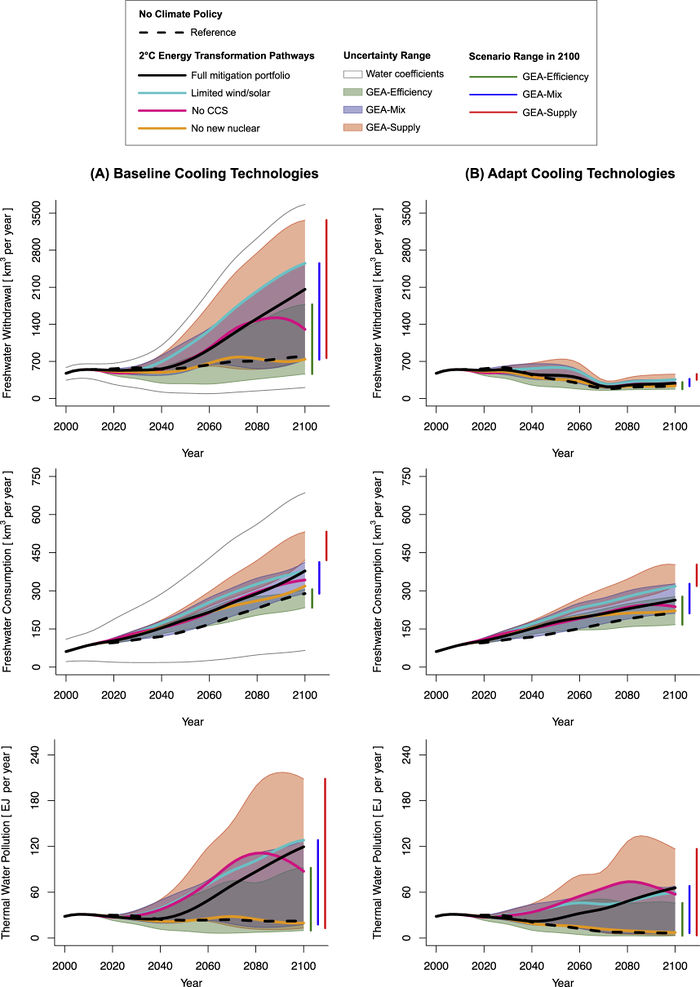
</figure>
Water consumption is responsive to the energy demand level, as depicted in <xr id="fig:MESSAGE-GLOBIOM_water1"/>. <xr id="fig:MESSAGE-GLOBIOM_water2"/> explores the relationship between energy demand and water use by computing the water consumption intensity of the energy pathways (global water consumption divided by final energy demand). The intensity of water consumption increases over the simulation period regardless of the demand level. The GEA-Efficiency scenarios display the largest range of water since low demand levels permit a greater flexibility in supply side technologies for climate change mitigation. (Fricko et al, 2016MSG-GLB_fricko_energy_2016)
<figure id="fig:MESSAGE-GLOBIOM_water2">
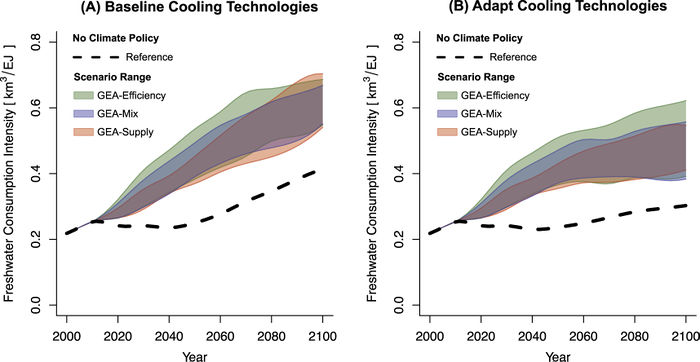
</figure>
8.4) Other sustainability dimensions - MESSAGE-GLOBIOM
Energy access
Three billion people globally depend on solid fuels for cooking and heating. According to Cameron et al (2016)MSG-GLB_cameron_policy_2016, "household air pollution from the incomplete combustion of these fuels globally leads to 4.3 million premature deaths each year, with 1.7 million of those in South Asia". The Indian government is subsidizing petroleum-based fuels (such as kerosene and liquefied petroleum gas - LPG) to increase their uptake, however, still more than 72% of Indians mostly rely on solid fuels. Further, it is expected that more stringent climate policies will increase the cost of fuels making a switch to cleaner cooking more challenging.
For studying energy access issues, a household fuel-choice model, Access, is used in combination with MESSAGE. At the moment the MESSAGE Access runs have been developed for South Asia, which has the largest amount of solid-fuel users in the world. These two models are run iteratively until convergence: the Access model takes fuel prices from MESSAGE, selects optimal fuel choices for all household groups, and returns aggregate residential demand for the five cooking fuels (LPG, piped gas, electricity, kerosene, and biomass). MESSAGE, in turn, determines the least-cost energy supply pathway to meet these demands and returns new prices. Climate policy is implemented from 2020 through 2100, with the implied carbon equivalent value rising at a discount rate of 5% per year over the time period.
The Access model reads in prices for five fuels from MESSAGE over the period from 2005 to 2100 and determines demand for each fuel in multiple heterogeneous population sub-groups. In this study, Access is implemented only for the MESSAGE South Asia region and represents only demand for cooking fuels. The Access model requires data inputs in three categories: 1) household characteristics and fuel preferences for each population sub-group calculated from nationally representative household surveys, 2) regional projections of population, GDP, urbanization, and electrification source and 3) cooking technology attribute data. When used in conjunction with MESSAGE, the two models iterate to account for the impact of changing household energy demands on fuel prices. MESSAGE-Access iterates until the output of the Access model from a given run is within 2% of its output from the previous run. This process is visualized in <xr id="fig:access"/>. (Cameron et al, 2016 MSG-GLB_cameron_policy_2016)
<figure id="fig:access">
</figure>
When current trends are projected to the future, the GHG emissions of India are expected to rise sharply by 2050. At the same time, urbanization and high expected GDP growth would also enable 1 billion people (63% of the population) to transition to clean cooking fuels over the period from 2010 to 2050 (see <xr id="fig:access1"/>). However, climate mitigation scenarios, even though reducing total emissions, could have a negative effect on the transition to clean cooking fuels, due to increased prices of LPG. <xr id="fig:access1"/> shows the effect of mitigation through the implementation carbon price if different stringency (US$10 (C10), US$20 (C20), US$30 (C30), and US$40 (C40) per ton CO2 equivalent in the year 2020) on GHG emissions versus solid-fuel reliance. (Cameron et al, 2016 MSG-GLB_cameron_policy_2016)
<figure id="fig:access1">
</figure>
The aforementioned negative impacts on energy access of mitigation policies can be counteracted by government policies. As is stated in Cameron et al (2016)MSG-GLB_cameron_policy_2016, "Policies that reduce stove costs shift more households to clean fuels per dollar invested than policies to reduce fuel costs. This is because, although stoves represent only a small share of the actual (levelized) cost of cooking with clean fuels, the high upfront costs of clean stoves represent a larger barrier to clean cooking uptake than fuel prices for many poor households." Therefore, as <xr id="fig:access2"/> shows, the most cost-effective measures are stove subsidies, and with different levels of support, different results in clean fuel uptake can be observed. For universal access, fuel price support would have to increase to 55 and 65%, respectively, with and without climate policy (at C30). (Cameron et al, 2016 MSG-GLB_cameron_policy_2016)
<figure id="fig:access2">
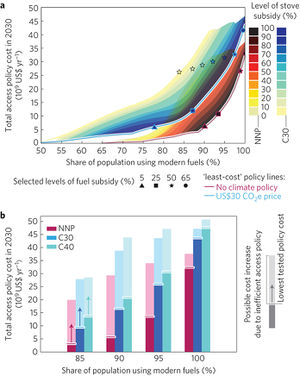
</figure>
The impacts of climate and energy access policies will depend on the household income group. The urban poor and higher expenditure rural households (U1 and R2) are likely to be the most affected by climate policy, but they are also likely to benefit the most of access policies. See <xr id="fig:access3"/> for more information. (Cameron et al, 2016 MSG-GLB_cameron_policy_2016)
<figure id="fig:access3">
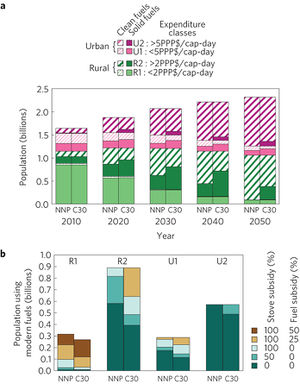
</figure>
9) Appendices - MESSAGE-GLOBIOM
For information on the mathematical formulation of MESSAGE and MACRO, please visit IIASA's MESSAGE-GLOBIOM documentation.
10) References - MESSAGE-GLOBIOM
{{#referencelist:
|references=MSG-GLB_lamarque_historical_2010;MSG-GLB_van_vuuren_representative_2011;MSG-GLB_rao_future_2016;MSG-GLB_fricko_energy_2016; MSG-GLB_cameron_policy_2016;MSG-GLB_keppo_short_2010;MSG-GLB_krey_implications_2009;MSG-GLB_oneill_mitigation_2010;MSG-GLB_schafer_structural_2005;MSG-GLB_messner_endogenized_1997;MSG-GLB_ekholm_determinants_2010;MSG-GLB_pachauri_pathways_2013;MSG-GLB_rogner_chapter_2012;MSG-GLB_hoogwijk_global_2004;MSG-GLB_hoogwijk_global_2008;MSG-GLB_christiansson_diffusion_1995;MSG-GLB_tubiello_reducing_2007;MSG-GLB_van_vuuren_future_2009;MSG-GLB_larson_chapter_2012;MSG-GLB_riahi_greenhouse_2000;MSG-GLB_riahi_prospects_2004;MSG-GLB_rao_role_2006;MSG-GLB_riahi_rcp_2011;MSG-GLB_riahi_scenarios_2007;MSG-GLB_wigley_magicc/scengen_2008;MSG-GLB_keppo_probabilistic_2007;MSG-GLB_meinshausen_greenhouse-gas_2009;MSG-GLB_meinshausen_what_2006;MSG-GLB_forest_quantifying_2002;MSG-GLB_amann_cost-effective_2011;MSG-GLB_amann_current_2004;MSG-GLB_berndes_contribution_2003;MSG-GLB_bringezu_assessing_2009;MSG-GLB_dornburg_biomass_2008;MSG-GLB_eickhout_local_2008;MSG-GLB_MSG-GLB_fischer_can_2009;MSG-GLB_granier_evolution_2011;MSG-GLB_manne_buying_1992;MSG-GLB_messner_messagemacro:_2000;MSG-GLB_nonhebel_energy_2007;MSG-GLB_plantinga_econometric_1999;MSG-GLB_rao_environmental_2012;MSG-GLB_rao_better_2013;MSG-GLB_riahi_chapter_2012;MSG-GLB_rokityanskiy_geographically_2007;MSG-GLB_sathaye_summary_2003;MSG-GLB_sathaye_ghg_2006;MSG-GLB_searchinger_use_2008;MSG-GLB_smeets_bottom-up_2007;MSG-GLB_smith_competition_2010;MSG-GLB_stavins_costs_1999;MSG-GLB_stehfest_climate_2009;MSG-GLB_van_vuuren_outlook_2009;MSG-GLB_van_vuuren_bio-energy_2010;MSG-GLB_van_vliet_copenhagen_2012;MSG-GLB_messner_users_1995;MSG-GLB_ipcc_climate_2007;MSG-GLB_ipcc_revised_1996;MSG-GLB_schneider_long-term_2008;MSG-GLB_loulou_markal-macro_2004;MSG-GLB_oneill_roads_2015;MSG-GLB_havlik_global_2011;MSG-GLB_havlik_climate_2014;MSG-GLB_kindermann_predicting_2006;MSG-GLB_gusti_algorithm_2010;MSG-GLB_meinshausen_emulating_2011;MSG-GLB_meinshausen_rcp_2011;MSG-GLB_rogelj_2020_2013;MSG-GLB_rogelj_probabilistic_2013;MSG-GLB_sullivan_electric_2013;MSG-GLB_rogelj_mitigation_2015;MSG-GLB_cofala_scenarios_2007;MSG-GLB_amann_regional_2013;MSG-GLB_environmental_protection_agency_epa_global_2013;MSG-GLB_joint_research_centre_global_emissions_emission_2011;MSG-GLB_rogner_assessment_1997;MSG-GLB_riahi_shared_2016;MSG-GLB_oneill_new_2014;MSG-GLB_kc_human_2014;MSG-GLB_dellink_long-term_2015;MSG-GLB_johnson_vre_2016;MSG-GLB_pietzcker_solar_2014;MSG-GLB_eurek_wind_2016;MSG-GLB_fricko_marker_2016;MSG-GLB_world_bank_group_world_2012;MSG-GLB_un_population_division_world_2010;MSG-GLB_international_energy_agency_energy_2012;MSG-GLB_international_energy_agency_world_2014;MSG-GLB_oecd_uranium_2003;MSG-GLB_leibowicz_growth_2015;MSG-GLB_alexandratos_world_2012;MSG-GLB_bouwman_exploring_2005;MSG-GLB_conant_grassland_2004;MSG-GLB_environmental_protection_agency_epa_US_2012;MSG-GLB_food_and_agricultural_organization_fao_global_2010;MSG-GLB_fritz_highlighting_2011;MSG-GLB_herrero_global_2013;MSG-GLB_herrero_systems_2008;MSG-GLB_keyzer_diet_2005;MSG-GLB_kindermann_global_2008;MSG-GLB_mccarl_surplus_1980;MSG-GLB_mitchell_improved_2005;MSG-GLB_muhammad_international_2011;MSG-GLB_oneill_meeting_2012;MSG-GLB_parton_analysis_1987;MSG-GLB_parton_observations_1993;MSG-GLB_ramankutty_farming_2008;MSG-GLB_reynolds_estimating_2000;MSG-GLB_ruesch_new_ipcc_2008;MSG-GLB_russ_global_2007;MSG-GLB_schloss_comparing_1999;MSG-GLB_schneider_agricultural_2007;MSG-GLB_seale_international_2003;MSG-GLB_sere_world_1996;MSG-GLB_skalsky_geo-bene_2008;MSG-GLB_takayama_spatial_1971;MSG-GLB_tubiello_faostat_2013;MSG-GLB_williams_computer_1995;MSG-GLB_wint_gridded_2007;MSG-GLB_you_entropy_2006;MSG-GLB_izaurralde_simulating_2006;MSG-GLB_FAO_global_2006;MSG-GLB_kindermann_global_forest_2008;MSG-GLB_biomass_handbook_2005;MSG-GLB_rametsteiner_study_2007;MSG-GLB_hamelinck_future_2001;MSG-GLB_leduc_optimal_2008;MSG-GLB_sørensen_economies_2005;MSG-GLB_carpentieri_future_1993;MSG-GLB_herzogbaum_forstpflanzen_2008;MSG-GLB_jurvélius_labor_1997;MSG-GLB_ILO_occupational_2007;MSG-GLB_FPP_holzernte_1999;MSG-GLB_jiroušek_productivity_2007;MSG-GLB_stokes_field_1986;MSG-GLB_wang_productivity_2004;MSG-GLB_hartsough_harvesting_2001;MSG-GLB_heston_penn_2006|+sep=; |browselinks=yes |columns=2 |header=List |listtype=ul
}}
{{#scite:
|bibtex=
@article{MSG-GLB_lamarque_historical_2010,
title = {Historical (1850–2000) gridded anthropogenic and biomass burning emissions of reactive gases and aerosols: methodology and application},
volume = {10},
issn = {1680-7324},
url = {http://www.atmos-chem-phys.net/10/7017/2010/},
doi = {10.5194/acp-10-7017-2010},
number = {15},
journal = {Atmos. Chem. Phys.},
author = {Lamarque, J.-F. and Bond, T. C. and Eyring, V. and Granier, C. and Heil, A. and Klimont, Z. and Lee, D. and Liousse, C. and Mieville, A. and Owen, B. and Schultz, M. G. and Shindell, D. and Smith, S. J. and Stehfest, E. and Van Aardenne, J. and Cooper, O. R. and Kainuma, M. and Mahowald, N. and McConnell, J. R. and Naik, V. and Riahi, K. and van Vuuren, D. P.},
month = aug,
year = {2010},
pages = {7017--7039}
}
}}
{{#scite:
|bibtex=
@article{MSG-GLB_van_vuuren_representative_2011,
title = {The representative concentration pathways: an overview},
volume = {109},
issn = {1573-1480},
url = {http://dx.doi.org/10.1007/s10584-011-0148-z},
doi = {10.1007/s10584-011-0148-z},
abstract = {This paper summarizes the development process and main characteristics of the Representative Concentration Pathways (RCPs), a set of four new pathways developed for the climate modeling community as a basis for long-term and near-term modeling experiments. The four RCPs together span the range of year 2100 radiative forcing values found in the open literature, i.e. from 2.6 to 8.5 W/m2. The RCPs are the product of an innovative collaboration between integrated assessment modelers, climate modelers, terrestrial ecosystem modelers and emission inventory experts. The resulting product forms a comprehensive data set with high spatial and sectoral resolutions for the period extending to 2100. Land use and emissions of air pollutants and greenhouse gases are reported mostly at a 0.5 × 0.5 degree spatial resolution, with air pollutants also provided per sector (for well-mixed gases, a coarser resolution is used). The underlying integrated assessment model outputs for land use, atmospheric emissions and concentration data were harmonized across models and scenarios to ensure consistency with historical observations while preserving individual scenario trends. For most variables, the RCPs cover a wide range of the existing literature. The RCPs are supplemented with extensions (Extended Concentration Pathways, ECPs), which allow climate modeling experiments through the year 2300. The RCPs are an important development in climate research and provide a potential foundation for further research and assessment, including emissions mitigation and impact analysis.},
number = {1},
journal = {Climatic Change},
author = {van Vuuren, Detlef P. and Edmonds, Jae and Kainuma, Mikiko and Riahi, Keywan and Thomson, Allison and Hibbard, Kathy and Hurtt, George C. and Kram, Tom and Krey, Volker and Lamarque, Jean-Francois and Masui, Toshihiko and Meinshausen, Malte and Nakicenovic, Nebojsa and Smith, Steven J. and Rose, Steven K.},
year = {2011},
pages = {5}
}
}}
{{#scite:
|bibtex=
@article{MSG-GLB_rao_future_2016,
title = {Future air pollution in the Shared Socio-economic Pathways},
issn = {0959-3780},
journal = {Global Environmental Change},
author = {Rao, Shilpa and Klimont, Zbigniew and Smith, Steven J and Van Dingenen, Rita and Dentener, Frank and Bouwman, Lex and Riahi, Keywan and Amann, Markus and Bodirsky, Benjamin Leon and van Vuuren, Detlef P},
year = {2016}
}
}}
{{#scite:
|bibtex=
@article{MSG-GLB_fricko_energy_2016,
title = {Energy sector water use implications of a 2° {C} climate policy},
volume = {11},
issn = {1748-9326},
number = {3},
journal = {Environmental Research Letters},
author = {Fricko, Oliver and Parkinson, Simon C and Johnson, Nils and Strubegger, Manfred and van Vliet, Michelle TH and Riahi, Keywan},
year = {2016},
pages = {034011}
}
}}
{{#scite:
|bibtex=
@article{MSG-GLB_cameron_policy_2016,
title = {Policy trade-offs between climate mitigation and clean cook-stove access in South Asia},
volume = {1},
issn = {2058-7546},
journal = {Nature Energy},
author = {Cameron, Colin and Pachauri, Shonali and Rao, Narasimha D and McCollum, David and Rogelj, Joeri and Riahi, Keywan},
year = {2016},
pages = {15010}
}
}}
{{#scite:
|bibtex=
@article{MSG-GLB_keppo_short_2010,
title = {Short term decisions for long term problems–The effect of foresight on model based energy systems analysis},
volume = {35},
issn = {0360-5442},
number = {5},
journal = {Energy},
author = {Keppo, Ilkka and Strubegger, Manfred},
year = {2010},
pages = {2033--2042}
}
}}
{{#scite:
|bibtex=
@article{MSG-GLB_krey_implications_2009,
title = {Implications of delayed participation and technology failure for the feasibility, costs, and likelihood of staying below temperature targets—Greenhouse gas mitigation scenarios for the 21st century},
volume = {31},
issn = {0140-9883},
journal = {Energy Economics},
author = {Krey, Volker and Riahi, Keywan},
year = {2009},
pages = {S94--S106}
}
}}
{{#scite:
|bibtex=
@article{MSG-GLB_oneill_mitigation_2010,
title = {Mitigation implications of midcentury targets that preserve long-term climate policy options},
volume = {107},
issn = {0027-8424},
number = {3},
journal = {Proceedings of the National Academy of Sciences},
author = {O’Neill, Brian C and Riahi, Keywan and Keppo, Ilkka},
year = {2010},
pages = {1011--1016}
}
}}
{{#scite:
|bibtex=
@article{MSG-GLB_schafer_structural_2005,
title = {Structural change in energy use},
volume = {33},
issn = {0301-4215},
number = {4},
journal = {Energy Policy},
author = {Schäfer, Andreas},
year = {2005},
pages = {429--437}
}
}}
{{#scite:
|bibtex=
@article{MSG-GLB_messner_endogenized_1997,
title = {Endogenized technological learning in an energy systems model},
volume = {7},
issn = {0936-9937},
number = {3},
journal = {Journal of Evolutionary Economics},
author = {Messner, Sabine},
year = {1997},
pages = {291--313}
}
}}
{{#scite:
|bibtex=
@article{MSG-GLB_ekholm_determinants_2010,
title = {Determinants of household energy consumption in India},
volume = {38},
issn = {0301-4215},
number = {10},
journal = {Energy Policy},
author = {Ekholm, Tommi and Krey, Volker and Pachauri, Shonali and Riahi, Keywan},
year = {2010},
pages = {5696--5707}
}
}}
{{#scite:
|bibtex=
@article{MSG-GLB_pachauri_pathways_2013,
title = {Pathways to achieve universal household access to modern energy by 2030},
volume = {8},
issn = {1748-9326},
number = {2},
journal = {Environmental Research Letters},
author = {Pachauri, Shonali and van Ruijven, Bas J and Nagai, Yu and Riahi, Keywan and van Vuuren, Detlef P and Brew-Hammond, Abeeku and Nakicenovic, Nebojsa},
year = {2013},
pages = {024015}
}
}}
{{#scite:
|bibtex=
@incollection{MSG-GLB_rogner_chapter_2012,
title = {Chapter 7 - Energy resources and potentials},
booktitle = {Global Energy Assessment - Toward a Sustainable Future},
publisher = {Cambridge University Press, Cambridge, UK and New York, NY, USA and the International Institute for Applied Systems Analysis, Laxenburg, Austria},
author = {Rogner, H and Aguilera, Roberto F and Archer, Christina and Bertani, Ruggero and Bhattacharya, S and Dusseault, M and Gagnon, Luc and Harbel, H and Hoogwijk, Monique and Johnson, Arthur},
year = {2012},
pages = {423--512}
}
}}
{{#scite:
|bibtex=
@report{MSG-GLB_hoogwijk_global_2004,
type = {report},
title = {On the global and regional potential of renewable energy sources (PhD Thesis)},
institution = {Department of Science, Technology and Society. Utrecht University},
author = {Hoogwijk, Monique Maria},
year = {2004}
}
}}
{{#scite:
|bibtex=
@article{MSG-GLB_hoogwijk_global_2008,
title = {Global potential of renewable energy sources: a literature assessment},
journal = {Background report prepared by order of REN21. Ecofys, PECSNL072975},
author = {Hoogwijk, Monique and Graus, Wina},
year = {2008}
}
}}
{{#scite:
|bibtex=
@article{MSG-GLB_christiansson_diffusion_1995,
title = {Diffusion and learning curves of renewable-energy technologies},
issn = {0304-7121},
journal = {IIASA Report},
author = {Christiansson, Lena},
year = {1995}
}
}}
{{#scite:
|bibtex=
@article{MSG-GLB_tubiello_reducing_2007,
title = {Reducing climate change impacts on agriculture: Global and regional effects of mitigation, 2000–2080},
volume = {74},
issn = {0040-1625},
number = {7},
journal = {Technological Forecasting and Social Change},
author = {Tubiello, Francesco N and Fischer, Günther},
year = {2007},
pages = {1030--1056}
}
}}
{{#scite:
|bibtex=
@article{MSG-GLB_van_vuuren_future_2009,
title = {Future bio-energy potential under various natural constraints},
volume = {37},
issn = {0301-4215},
number = {11},
journal = {Energy Policy},
author = {Van Vuuren, Detlef P and van Vliet, Jasper and Stehfest, Elke},
year = {2009},
pages = {4220--4230}
}
}}
{{#scite:
|bibtex=
@incollection{MSG-GLB_larson_chapter_2012,
address = {Cambridge University Press, Cambridge, UK and New York, NY, USA and the International Institute for Applied Systems Analysis, Laxenburg, Austria},
title = {Chapter 12 - Fossil Energy},
isbn = {9781 10700 5198 hardback 9780 52118 2935 paperback},
shorttitle = {Chapter 12 - Fossil Energy},
url = {www.globalenergyassessment.org},
booktitle = {Global Energy Assessment - Toward a Sustainable Future},
author = {Larson, Eric D. and Li, Zheng and Williams, Robert H.},
year = {2012},
pages = {901--992}
}
}}
{{#scite:
|bibtex=
@article{MSG-GLB_riahi_greenhouse_2000,
title = {Greenhouse gas emissions in a dynamics-as-usual scenario of economic and energy development},
volume = {63},
issn = {0040-1625},
number = {2},
journal = {Technological Forecasting and Social Change},
author = {Riahi, Keywan and Roehrl, R Alexander},
year = {2000},
pages = {175--205}
}
}}
{{#scite:
|bibtex=
@article{MSG-GLB_riahi_prospects_2004,
title = {Prospects for carbon capture and sequestration technologies assuming their technological learning},
volume = {29},
issn = {0360-5442},
number = {9},
journal = {Energy},
author = {Riahi, Keywan and Rubin, Edward S and Schrattenholzer, Leo},
year = {2004},
pages = {1309--1318}
}
}}
{{#scite:
|bibtex=
@article{MSG-GLB_rao_role_2006,
title = {The Role of Non-CO₃ Greenhouse Gases in Climate Change Mitigation: Long-term Scenarios for the 21st Century},
issn = {0195-6574},
journal = {The Energy Journal},
author = {Rao, Shilpa and Riahi, Keywan},
year = {2006},
pages = {177--200}
}
}}
{{#scite:
|bibtex=
@article{MSG-GLB_riahi_rcp_2011,
title = {{RCP} 8.5—{A} scenario of comparatively high greenhouse gas emissions},
volume = {109},
issn = {0165-0009},
number = {1-2},
journal = {Climatic Change},
author = {Riahi, Keywan and Rao, Shilpa and Krey, Volker and Cho, Cheolhung and Chirkov, Vadim and Fischer, Guenther and Kindermann, Georg and Nakicenovic, Nebojsa and Rafaj, Peter},
year = {2011},
pages = {33--57}
}
}}
{{#scite:
|bibtex=
@article{MSG-GLB_riahi_scenarios_2007,
title = {Scenarios of long-term socio-economic and environmental development under climate stabilization},
volume = {74},
issn = {0040-1625},
number = {7},
journal = {Technological Forecasting and Social Change},
author = {Riahi, Keywan and Grübler, Arnulf and Nakicenovic, Nebojsa},
year = {2007},
pages = {887--935}
}
}}
{{#scite:
|bibtex=
@article{MSG-GLB_wigley_magicc/scengen_2008,
title = {{MAGICC}/{SCENGEN} 5.3: {User} manual (version 2)},
volume = {80},
journal = {NCAR, Boulder, CO},
author = {Wigley, Tom ML},
year = {2008}
}
}}
{{#scite:
|bibtex=
@article{MSG-GLB_keppo_probabilistic_2007,
title = {Probabilistic temperature change projections and energy system implications of greenhouse gas emission scenarios},
volume = {74},
issn = {0040-1625},
number = {7},
journal = {Technological Forecasting and Social Change},
author = {Keppo, Ilkka and O'Neill, Brian C and Riahi, Keywan},
year = {2007},
pages = {936--961}
}
}}
{{#scite:
|bibtex=
@article{MSG-GLB_meinshausen_greenhouse-gas_2009,
title = {Greenhouse-gas emission targets for limiting global warming to 2 {C}},
volume = {458},
issn = {0028-0836},
number = {7242},
journal = {Nature},
author = {Meinshausen, Malte and Meinshausen, Nicolai and Hare, William and Raper, Sarah CB and Frieler, Katja and Knutti, Reto and Frame, David J and Allen, Myles R},
year = {2009},
pages = {1158--1162}
}
}}
{{#scite:
|bibtex=
@article{MSG-GLB_meinshausen_what_2006,
title = {What does a 2 {C} target mean for greenhouse gas concentrations? {A} brief analysis based on multi-gas emission pathways and several climate sensitivity uncertainty estimates},
volume = {270},
journal = {Avoiding dangerous climate change},
author = {Meinshausen, Malte},
year = {2006}
}
}}
{{#scite:
|bibtex=
@article{MSG-GLB_forest_quantifying_2002,
title = {Quantifying uncertainties in climate system properties with the use of recent climate observations},
volume = {295},
issn = {0036-8075},
number = {5552},
journal = {Science},
author = {Forest, Chris E and Stone, Peter H and Sokolov, Andrei P and Allen, Myles R and Webster, Mort D},
year = {2002},
pages = {113--117}
}
}}
{{#scite:
|bibtex=
@article{MSG-GLB_amann_cost-effective_2011,
title = {Cost-effective control of air quality and greenhouse gases in {Europe}: {Modeling} and policy applications},
volume = {26},
issn = {1364-8152},
shorttitle = {Cost-effective control of air quality and greenhouse gases in {Europe}},
url = {http://www.sciencedirect.com/science/article/pii/S1364815211001733},
doi = {10.1016/j.envsoft.2011.07.012},
number = {12},
urldate = {2016-03-24},
journal = {Environmental Modelling \& Software},
author = {Amann, Markus and Bertok, Imrich and Borken-Kleefeld, Jens and Cofala, Janusz and Heyes, Chris and Höglund-Isaksson, Lena and Klimont, Zbigniew and Nguyen, Binh and Posch, Maximilian and Rafaj, Peter and Sandler, Robert and Schöpp, Wolfgang and Wagner, Fabian and Winiwarter, Wilfried},
month = dec,
year = {2011},
keywords = {Air pollution, Convention on Long-range transboundary air pollution, Cost-effectiveness, Decision support, GAINS model, Integrated assessment, Science–policy interface},
pages = {1489--1501}
}
}}
{{#scite:
|bibtex=
@article{MSG-GLB_amann_current_2004,
title = {Current {Legislation}” and the “{Maximum} {Technically} {Feasible} {Reduction}” cases for the {CAFE} baseline emission projections},
url = {https://www.researchgate.net/profile/Zbigniew_Klimont/publication/230709494_The_Current_Legislation_and_the_Maximum_Technically_Feasible_Reduction_cases_for_the_CAFE_baseline_emission_projections._CAFE_Report__2/links/0deec53cd2d778aafb000000.pdf},
urldate = {2016-03-24},
journal = {IIASA, Vienna},
author = {Amann, Markus and Cabala, Rafal and Cofala, Janusz and Heyes, Chris and Klimont, Zbigniew and Schöpp, Wolfgang and Tarrason, Leonor and Simpson, David and Wind, Peter and Jonson, Jan-Eiof},
year = {2004}
}
}}
{{#scite:
|bibtex=
@article{MSG-GLB_berndes_contribution_2003,
title = {The contribution of biomass in the future global energy supply: a review of 17 studies},
volume = {25},
issn = {0961-9534},
shorttitle = {The contribution of biomass in the future global energy supply},
url = {http://www.sciencedirect.com/science/article/pii/S096195340200185X},
doi = {10.1016/S0961-9534(02)00185-X},
abstract = {This paper discusses the contribution of biomass in the future global energy supply. The discussion is based on a review of 17 earlier studies on the subject. These studies have arrived at widely different conclusions about the possible contribution of biomass in the future global energy supply (e.g., from below 100 EJ yr−1 to above 400 EJ yr−1 in 2050). The major reason for the differences is that the two most crucial parameters—land availability and yield levels in energy crop production—are very uncertain, and subject to widely different opinions (e.g., the assessed 2050 plantation supply ranges from below 50 EJ yr−1 to almost 240 EJ yr−1). However, also the expectations about future availability of forest wood and of residues from agriculture and forestry vary substantially among the studies.
The question how an expanding bioenergy sector would interact with other land uses, such as food production, biodiversity, soil and nature conservation, and carbon sequestration has been insufficiently analyzed in the studies. It is therefore difficult to establish to what extent bioenergy is an attractive option for climate change mitigation in the energy sector. A refined modeling of interactions between different uses and bioenergy, food and materials production—i.e., of competition for resources, and of synergies between different uses—would facilitate an improved understanding of the prospects for large-scale bioenergy and of future land-use and biomass management in general}, number = {1}, urldate = {2016-03-24}, journal = {Biomass and Bioenergy}, author = {Berndes, Göran and Hoogwijk, Monique and van den Broek, Richard}, month = jul, year = {2003}, keywords = {Assessment, Bioenergy, Biomass energy, Global, Potential, Regional, Review, Scenario}, pages = {1--28} } }}
{{#scite:
|bibtex=
@book{MSG-GLB_bringezu_assessing_2009,
title = {Assessing biofuels: towards sustainable production and use of resources},
isbn = {92-807-3052-5},
publisher = {United Nations Environment Programme},
author = {Bringezu, Stefan and Schütz, Helmut and O’Brien, Meghan and Kauppi, Lea and Howarth, Robert W and McNeely, Jeff},
year = {2009}
}
}}
{{#scite:
|bibtex=
@article{MSG-GLB_dornburg_biomass_2008,
title = {Biomass assessment: assessment of global biomass potentials and their links to food, water, biodiversity, energy demand and economy: inventory and analysis of existing studies: supporting document},
number = {500102 014},
journal = {Report/WAB},
author = {Dornburg, Veronika and Faaij, APC and Verweij, PA and Banse, Martin and Diepen, Kees van and Keulen, Herman van and Langeveld, Hans and Meeusen, Marieke and Ven, Gerrie van de and Wester, Flip},
year = {2008}
}
}}
{{#scite:
|bibtex=
@article{MSG-GLB_eickhout_local_2008,
title = {Local and global consequences of the {EU} renewable directive for biofuels: {Testing} the sustainability criteria},
journal = {Local and global consequences of the EU renewable directive for biofuels: testing the sustainability criteria},
author = {Eickhout, Bas and van den Born, Gert Jan and Notenboom, Jos and Oorschot, M van and Ros, JPM and Van Vuuren, DP and Westhoek, HJ},
year = {2008}
}
}}
{{#scite:
|bibtex=
@report{MSG-GLB_MSG-GLB_fischer_can_2009,
title = {Can technology deliver on the yield challenge to 2050?},
url = {http://www.fao.org/3/a-ak542e/ak542e12a.pdf},
institution = {Expert Meeting on How to feed the World in 2050. Food and Agriculture Organization of the United Nations},
author = {Fischer, RA and Byerlee, Derek and Edmeades, Gregory O},
year = {2009}
}
}}
{{#scite:
|bibtex=
@article{MSG-GLB_granier_evolution_2011,
title = {Evolution of anthropogenic and biomass burning emissions of air pollutants at global and regional scales during the 1980–2010 period},
volume = {109},
issn = {0165-0009},
number = {1-2},
journal = {Climatic Change},
author = {Granier, Claire and Bessagnet, Bertrand and Bond, Tami and D’Angiola, Ariela and van Der Gon, Hugo Denier and Frost, Gregory J and Heil, Angelika and Kaiser, Johannes W and Kinne, Stefan and Klimont, Zbigniew},
year = {2011},
pages = {163--190}
}
}}
{{#scite:
|bibtex=
@book{MSG-GLB_manne_buying_1992,
title = {Buying greenhouse insurance: the economic costs of carbon dioxide emission limits},
isbn = {0-262-13280-X},
publisher = {MIT press},
author = {Manne, Alan Sussmann and Richels, Richard G},
year = {1992}
}
}}
{{#scite:
|bibtex=
@article{MSG-GLB_messner_messagemacro:_2000,
title = {MESSAGE–MACRO: linking an energy supply model with a macroeconomic module and solving it iteratively},
volume = {25},
issn = {0360-5442},
number = {3},
journal = {Energy},
author = {Messner, Sabine and Schrattenholzer, Leo},
year = {2000},
pages = {267--282}
}
}}
{{#scite:
|bibtex=
@article{MSG-GLB_nonhebel_energy_2007,
title = {Energy from agricultural residues and consequences for land requirements for food production},
volume = {94},
issn = {0308-521X},
number = {2},
journal = {Agricultural Systems},
author = {Nonhebel, Sanderine},
year = {2007},
pages = {586--592}
}
}}
{{#scite:
|bibtex=
@article{MSG-GLB_plantinga_econometric_1999,
title = {An econometric analysis of the costs of sequestering carbon in forests},
volume = {81},
issn = {0002-9092},
number = {4},
journal = {American Journal of Agricultural Economics},
author = {Plantinga, Andrew J and Mauldin, Thomas and Miller, Douglas J},
year = {1999},
pages = {812--824}
}
}}
{{#scite:
|bibtex=
@article{MSG-GLB_rao_environmental_2012,
title = {Environmental modeling and methods for estimation of the global health impacts of air pollution},
volume = {17},
issn = {1420-2026},
number = {6},
journal = {Environmental Modeling \& Assessment},
author = {Rao, Shilpa and Chirkov, Vadim and Dentener, Frank and Van Dingenen, Rita and Pachauri, Shonali and Purohit, Pallav and Amann, Markus and Heyes, Chris and Kinney, Patrick and Kolp, Peter},
year = {2012},
pages = {613--622}
}
}}
{{#scite:
|bibtex=
@article{MSG-GLB_rao_better_2013,
title = {Better air for better health: {Forging} synergies in policies for energy access, climate change and air pollution},
volume = {23},
issn = {0959-3780},
number = {5},
journal = {Global environmental change},
author = {Rao, Shilpa and Pachauri, Shonali and Dentener, Frank and Kinney, Patrick and Klimont, Zbigniew and Riahi, Keywan and Schoepp, Wolfgang},
year = {2013},
pages = {1122--1130}
}
}}
{{#scite:
|bibtex=
@incollection{MSG-GLB_riahi_chapter_2012,
address = {Cambridge University Press, Cambridge, UK and New York, NY, USA and the International Institute for Applied Systems Analysis, Laxenburg, Austria},
title = {Chapter 17 - Energy Pathways for Sustainable Development},
isbn = {9781 10700 5198 hardback 9780 52118 2935 paperback},
shorttitle = {Chapter 17 - Energy Pathways for Sustainable Development},
url = {www.globalenergyassessment.org},
booktitle = {Global Energy Assessment - Toward a Sustainable Future},
author = {Riahi, Keywan and Dentener, Frank and Gielen, Dolf and Grubler, Arnulf and Jewell, Jessica and Klimont, Zbigniew and Krey, Volker and McCollum, David and Pachauri, Shonali and Rao, Shilpa and van Ruijven, Bas and van Vuuren, Detlef P. and Wilson, Charlie},
year = {2012},
pages = {1203--1306}
}
}}
{{#scite:
|bibtex=
@article{MSG-GLB_rokityanskiy_geographically_2007,
title = {Geographically explicit global modeling of land-use change, carbon sequestration, and biomass supply},
volume = {74},
issn = {0040-1625},
number = {7},
journal = {Technological Forecasting and Social Change},
author = {Rokityanskiy, Dmitry and Benítez, Pablo C and Kraxner, Florian and McCallum, Ian and Obersteiner, Michael and Rametsteiner, Ewald and Yamagata, Yoshiki},
year = {2007},
pages = {1057--1082}
}
}}
{{#scite:
|bibtex=
@article{MSG-GLB_sathaye_summary_2003,
title = {A summary note estimating global forestry {GHG} mitigation potential and costs: {A} dynamic partial equilibrium approach},
volume = {10},
journal = {working draft, August},
author = {Sathaye, Jayant and Chan, Peter and Dale, Larry and Makundi, Willy and Andrasko, Ken},
year = {2003},
pages = {448--457}
}
}}
{{#scite:
|bibtex=
@article{MSG-GLB_sathaye_ghg_2006,
title = {{GHG} mitigation potential, costs and benefits in global forests: a dynamic partial equilibrium approach},
issn = {0195-6574},
journal = {The Energy Journal},
author = {Sathaye, Jayant and Makundi, Willy and Dale, Larry and Chan, Peter and Andrasko, Kenneth},
year = {2006},
pages = {127--162}
}
}}
{{#scite:
|bibtex=
@article{MSG-GLB_searchinger_use_2008,
title = {Use of {US} croplands for biofuels increases greenhouse gases through emissions from land-use change},
volume = {319},
issn = {0036-8075},
number = {5867},
journal = {Science},
author = {Searchinger, Timothy and Heimlich, Ralph and Houghton, Richard A and Dong, Fengxia and Elobeid, Amani and Fabiosa, Jacinto and Tokgoz, Simla and Hayes, Dermot and Yu, Tun-Hsiang},
year = {2008},
pages = {1238--1240}
}
}}
{{#scite:
|bibtex=
@article{MSG-GLB_smeets_bottom-up_2007,
title = {A bottom-up assessment and review of global bio-energy potentials to 2050},
volume = {33},
issn = {0360-1285},
number = {1},
journal = {Progress in Energy and combustion science},
author = {Smeets, Edward MW and Faaij, André PC and Lewandowski, Iris M and Turkenburg, Wim C},
year = {2007},
pages = {56--106}
}
}}
{{#scite:
|bibtex=
@article{MSG-GLB_smith_competition_2010,
title = {Competition for land},
volume = {365},
issn = {0962-8436},
number = {1554},
journal = {Philosophical Transactions of the Royal Society of London B: Biological Sciences},
author = {Smith, Pete and Gregory, Peter J and Van Vuuren, Detlef and Obersteiner, Michael and Havlík, Petr and Rounsevell, Mark and Woods, Jeremy and Stehfest, Elke and Bellarby, Jessica},
year = {2010},
pages = {2941--2957}
}
}}
{{#scite:
|bibtex=
@article{MSG-GLB_stavins_costs_1999,
title = {The costs of carbon sequestration: a revealed-preference approach},
volume = {89},
issn = {0002-8282},
number = {4},
journal = {The American Economic Review},
author = {Stavins, Robert N},
year = {1999},
pages = {994--1009}
}
}}
{{#scite:
|bibtex=
@article{MSG-GLB_stehfest_climate_2009,
title = {Climate benefits of changing diet},
volume = {95},
issn = {0165-0009},
number = {1-2},
journal = {Climatic change},
author = {Stehfest, Elke and Bouwman, Lex and Van Vuuren, Detlef P and Den Elzen, Michel GJ and Eickhout, Bas and Kabat, Pavel},
year = {2009},
pages = {83--102}
}
}}
{{#scite:
|bibtex=
@incollection{MSG-GLB_van_vuuren_outlook_2009,
title = {Outlook on agricultural changes and its drivers},
isbn = {1-59726-538-1},
booktitle = {Agriculture at a {Crossroads}-the {Global} {Report} of the {International} {Assessment} of {Agricultural} {Knowledge}, {Science}, and {Technology}},
publisher = {Island Press},
author = {van Vuuren, Detlef and Ochola, Washington and Riha, Susan and Giampietro, Mario and Ginzo, Hector and Henrichs, Thomas and Hussain, Sajidin Hussain and Kok, Kaspar and Makhura, Moraka Makhura and Mirza, Monirul},
year = {2009}
}
}}
{{#scite:
|bibtex=
@article{MSG-GLB_van_vuuren_bio-energy_2010,
title = {Bio-energy use and low stabilization scenarios},
issn = {0195-6574},
journal = {The Energy Journal},
author = {Van Vuuren, Detlef P and Bellevrat, Elie and Kitous, Alban and Isaac, Morna},
year = {2010},
pages = {193--221}
}
}}
{{#scite:
|bibtex=
@article{MSG-GLB_van_vliet_copenhagen_2012,
title = {Copenhagen accord pledges imply higher costs for staying below 2 {C} warming},
volume = {113},
issn = {0165-0009},
number = {2},
journal = {Climatic Change},
author = {van Vliet, Jasper and van den Berg, Maarten and Schaeffer, Michiel and van Vuuren, Detlef P and Den Elzen, Michel and Hof, Andries F and Beltran, Angelica Mendoza and Meinshausen, Malte},
year = {2012},
pages = {551--561}
}
}}
{{#scite:
|bibtex=
@report{MSG-GLB_messner_users_1995,
title = {User's Guide for MESSAGE III},
url = {http://webarchive.iiasa.ac.at/Admin/PUB/Documents/WP-95-069.pdf},
institution = {International Institute for Applied Systems Analysis (IIASA)},
author = {Messner, Sabine and Strubegger, Manfred},
year = {1995}
}
}}
{{#scite:
|bibtex=
@book{MSG-GLB_ipcc_climate_2007,
title = {Climate Change 2007: Synthesis Report. Contribution of Working Groups I, II and III to the Fourth Assessment Report of the Intergovernmental Panel on Climate Change},
url = {http://www.ipcc.ch/pdf/assessment-report/ar4/syr/ar4_syr_full_report.pdf},
publisher = {IPCC, Geneva, Switzerland},
author = {IPCC},
year = {2007}
}
}}
{{#scite:
|bibtex=
@book{MSG-GLB_ipcc_revised_1996,
title = {Revised 1996 {IPCC} Guidelines for National Greenhouse Gas Inventories: The Workbook (Volume 2)},
url = {http://www.ipcc-nggip.iges.or.jp/public/gl/invs5a.html},
publisher = {IPCC, Geneva, Switzerland},
author = {IPCC},
year = {1996}
}
}}
{{#scite:
|bibtex=
@article{MSG-GLB_schneider_long-term_2008,
title = {Long-term uranium supply estimates},
volume = {162},
number = {3},
journal = {Nuclear Technology},
author = {Schneider, Erich A and Sailor, William C},
year = {2008},
pages = {379--387}
}
}}
{{#scite:
|bibtex=
@book{MSG-GLB_loulou_markal-macro_2004,
author = "Richard Loulou and Gary Goldstein and Ken Noble",
title = "Documentation for the MARKAL Family of Models - Part II: MARKAL-MACRO",
publisher = "IEA Energy Technology Systems Analysis Programme (ETSAP)",
year = "2004",
type = "Manual",
month = "October",
url = "http://www.iea-etsap.org/web/MrklDoc-II_MARKALMACRO.pdf",
}
}}
{{#scite:
|bibtex=
@article{MSG-GLB_oneill_roads_2015,
title = {The roads ahead: narratives for shared socioeconomic pathways describing world futures in the 21st century},
issn = {0959-3780},
journal = {Global Environmental Change},
author = {O’Neill, Brian C and Kriegler, Elmar and Ebi, Kristie L and Kemp-Benedict, Eric and Riahi, Keywan and Rothman, Dale S and van Ruijven, Bas J and van Vuuren, Detlef P and Birkmann, Joern and Kok, Kasper},
year = {2015}
}
}}
{{#scite:
|bibtex=
@article{MSG-GLB_havlik_global_2011,
title = {Global land-use implications of first and second generation biofuel targets},
volume = {39},
issn = {0301-4215},
number = {10},
journal = {Energy Policy},
author = {Havlík, Petr and Schneider, Uwe A and Schmid, Erwin and Böttcher, Hannes and Fritz, Steffen and Skalský, Rastislav and Aoki, Kentaro and De Cara, Stephane and Kindermann, Georg and Kraxner, Florian},
year = {2011},
pages = {5690--5702}
}
}}
{{#scite:
|bibtex=
@article{MSG-GLB_havlik_climate_2014,
title = {Climate change mitigation through livestock system transitions},
volume = {111},
issn = {0027-8424},
number = {10},
journal = {Proceedings of the National Academy of Sciences},
author = {Havlík, Petr and Valin, Hugo and Herrero, Mario and Obersteiner, Michael and Schmid, Erwin and Rufino, Mariana C and Mosnier, Aline and Thornton, Philip K and Böttcher, Hannes and Conant, Richard T},
year = {2014},
pages = {3709--3714}
}
}}
{{#scite:
|bibtex=
@article{MSG-GLB_kindermann_predicting_2006,
title = {Predicting the deforestation-trend under different carbon-prices},
volume = {1},
number = {1},
journal = {Carbon Balance and management},
author = {Kindermann, Georg E and Obersteiner, Michael and Rametsteiner, Ewald and McCallum, Ian},
year = {2006},
pages = {15}
}
}}
{{#scite:
|bibtex=
@article{MSG-GLB_gusti_algorithm_2010,
title = {An algorithm for simulation of forest management decisions in the global forest model},
issn = {1561-5359},
journal = {Штучний інтелект},
author = {Gusti, MI},
year = {2010}
}
}}
{{#scite:
|bibtex=
@article{MSG-GLB_meinshausen_emulating_2011,
title = {Emulating coupled atmosphere-ocean and carbon cycle models with a simpler model, {MAGICC}6–{Part} 1: {Model} description and calibration},
volume = {11},
issn = {1680-7316},
number = {4},
journal = {Atmospheric Chemistry and Physics},
author = {Meinshausen, Malte and Raper, SCB and Wigley, TML},
year = {2011},
pages = {1417--1456}
}
}}
{{#scite:
|bibtex=
@article{MSG-GLB_meinshausen_rcp_2011,
title = {The {RCP} greenhouse gas concentrations and their extensions from 1765 to 2300},
volume = {109},
issn = {0165-0009},
number = {1-2},
journal = {Climatic change},
author = {Meinshausen, Malte and Smith, Steven J and Calvin, K and Daniel, John S and Kainuma, MLT and Lamarque, JF and Matsumoto, K and Montzka, SA and Raper, SCB and Riahi, K},
year = {2011},
pages = {213--241}
}
}}
{{#scite:
|bibtex=
@article{MSG-GLB_rogelj_2020_2013,
title = {2020 emissions levels required to limit warming to below 2°C},
volume = {3},
issn = {1758-678X},
number = {4},
journal = {Nature Climate Change},
author = {Rogelj, Joeri and McCollum, David L and O’Neill, Brian C and Riahi, Keywan},
year = {2013},
pages = {405--412}
}
}}
{{#scite:
|bibtex=
@article{MSG-GLB_rogelj_probabilistic_2013,
title = {Probabilistic cost estimates for climate change mitigation},
volume = {493},
issn = {0028-0836},
number = {7430},
journal = {Nature},
author = {Rogelj, Joeri and McCollum, David L and Reisinger, Andy and Meinshausen, Malte and Riahi, Keywan},
year = {2013},
pages = {79--83}
}
}}
{{#scite:
|bibtex=
@article{MSG-GLB_sullivan_electric_2013,
author = {Sullivan, Patrick and Krey, Volker and Riahi, Keywan}, title = {Impacts of considering electric sector variability and reliability in the MESSAGE model}, journal = {Energy Strategy Reviews}, volume = {1}, number = {3}, pages = {157-163}, year = {2013}
} }}
{{#scite: |bibtex= @article{MSG-GLB_rogelj_mitigation_2015, title = {Mitigation choices impact carbon budget size compatible with low temperature goals}, volume = {10}, issn = {1748-9326}, number = {7}, journal = {Environmental Research Letters}, author = {Rogelj, Joeri and Reisinger, Andy and McCollum, David L and Knutti, Reto and Riahi, Keywan and Meinshausen, Malte}, year = {2015}, pages = {075003} } }}
{{#scite:
|bibtex=
@article{MSG-GLB_cofala_scenarios_2007,
title = {Scenarios of global anthropogenic emissions of air pollutants and methane until 2030},
volume = {41},
issn = {1352-2310},
number = {38},
journal = {Atmospheric Environment},
author = {Cofala, Janusz and Amann, Markus and Klimont, Zbigniew and Kupiainen, Kaarle and Höglund-Isaksson, Lena},
year = {2007},
pages = {8486--8499}
}
}}
{{#scite:
|bibtex=
@article{MSG-GLB_amann_regional_2013,
title = {Regional and global emissions of air pollutants: {Recent} trends and future scenarios},
volume = {38},
issn = {1543-5938},
journal = {Annual Review of Environment and Resources},
author = {Amann, Markus and Klimont, Zbigniew and Wagner, Fabian},
year = {2013},
pages = {31--55}
}
}}
{{#scite:
|bibtex=
@webpage{MSG-GLB_environmental_protection_agency_epa_global_2013,
Type = webpage
title = {Global {Mitigation} of {Non}-{CO}2 {Greenhouse} {Gases}: 2010-2030},
url = {https://www3.epa.gov/climatechange/Downloads/EPAactivities/MAC_Report_2013.pdf},
author = {Environmental Protection Agency (EPA)},
year = {2013}
}
}}
{{#scite:
|bibtex=
@webpage{MSG-GLB_joint_research_centre_global_emissions_emission_2011,
title = {Emission {Database} for {Global} {Atmospheric} {Research} {EDGAR} v4.2},
url = {http://edgar.jrc.ec.europa.eu/overview.php?v=42},
author = {Joint Research Centre, Global Emissions},
month = nov,
year = {2011}
}
}}
{{#scite:
|bibtex=
@article{MSG-GLB_rogner_assessment_1997,
title = {An assessment of world hydrocarbon resources},
volume = {22},
issn = {1056-3466},
number = {1},
journal = {Annual review of energy and the environment},
author = {Rogner, Hans-Holger},
year = {1997},
pages = {217--262}
}
}}
{{#scite:
|bibtex=
@article{MSG-GLB_riahi_shared_2016,
title = {The Shared Socioeconomic Pathways and their Energy, Land Use, and Greenhouse Gas Emissions Implications},
volume = {in press},
doi = {10.1016/j.gloenvcha.2016.05.009},
journal = {Global Environmental Change},
author = {Riahi, Keywan and Vuuren, Detlef P. van and Kriegler, Elmar and Edmonds, Jae and O’Neill, Brian and Fujimori, Shinichiro and Bauer, Nico and Calvin, Katherine and Dellink, Rob and Fricko, Oliver and Lutz, Wolfgang and Popp, Alexander and Cuaresma, Jesus Crespo and KC, Samir and Leimbach, Marian and Jiang, Leiwen and Kram, Tom and Rao, Shilpa and Emmerling, Johannes and Ebi, Kristie and Hasegawa, Tomoko and Havlik, Petr and Humpenöder, Florian and Silva, Lara Aleluia Da and Smith, Steve and Stehfest, Elke and Bosetti, Valentina and Eom, Jiyong and Gernaat, David and Masui, Toshihiko and Rogelj, Joeri and Strefler, Jessica and Drouet, Laurent and Krey, Volker and Luderer, Gunnar and Harmsen, Mathijs and Takahashi, Kiyoshi and Baumstark, Lavinia and Doelman, Jonathan and Kainuma, Mikiko and Klimont, Zbigniew and Marangoni, Giacomo and Lotze-Campen, Hermann and Obersteiner, Michael and Tabeau, Andrzej and Tavoni, Massimo},
url = {http://pure.iiasa.ac.at/13280/},
year = {2016}
}
}}
{{#scite:
|bibtex=
@article{MSG-GLB_oneill_new_2014,
title = {A new scenario framework for climate change research: the concept of shared socioeconomic pathways},
volume = {122},
issn = {0165-0009},
number = {3},
journal = {Climatic Change},
author = {O’Neill, Brian C and Kriegler, Elmar and Riahi, Keywan and Ebi, Kristie L and Hallegatte, Stephane and Carter, Timothy R and Mathur, Ritu and van Vuuren, Detlef P},
year = {2014},
pages = {387--400}
}
}}
{{#scite:
|bibtex=
@article{MSG-GLB_kc_human_2014,
title = {The human core of the shared socioeconomic pathways: {Population} scenarios by age, sex and level of education for all countries to 2100},
issn = {0959-3780},
journal = {Global Environmental Change},
author = {KC, Samir and Lutz, Wolfgang},
year = {2014}
}
}}
{{#scite:
|bibtex=
@article{MSG-GLB_dellink_long-term_2015,
title = {Long-term economic growth projections in the Shared Socioeconomic Pathways},
issn = {0959-3780},
journal = {Global Environmental Change},
author = {Dellink, Rob and Chateau, Jean and Lanzi, Elisa and Magné, Bertrand},
year = {2015},
url = {http://pure.iiasa.ac.at/13280/}
}
}}
{{#scite:
|bibtex=
@article{MSG-GLB_johnson_vre_2016,
title = {A reduced-form approach for representing the impacts of wind and solar PV deployment on the structure and operation of the electricity system},
author = {Johnson, Nils and Strubegger, Manfred and McPherson, Madleine and Parkinson, Simon and Krey, Volker and Sullivan, Patrick},
journal = {Energy Economics},
volume = {In Review},
year = {2016}
}
}}
{{#scite: |bibtex= @article{MSG-GLB_pietzcker_solar_2014, title = {Using the sun to decarbonize the power sector: The economic potential of photovoltaics and concentrating solar power}, author = {Pietzcker, R. C. and Stetter, D. and Manger, S. and Luderer, G.}, journal = {Applied Energy}, volume = {135}, year = {2014}, pages = {704-720} } }}
{{#scite:
|bibtex=
@article{MSG-GLB_eurek_wind_2016,
title = {An improved global wind resource estimate for integrated assessment models},
author = {Eurek, K. and Sullivan, P. and Gleason, M. and Hettinger, D. and Heimiller, D.M. and Lopez, A.},
journal = {Energy Economics},
volume = {In Review},
year = {2016}
}
}}
{{#scite:
|bibtex=
@article{MSG-GLB_fricko_marker_2016,
title = {The marker quantification of the shared socioeconomic pathway 2: a middle-of-the-road scenario for the 21st century},
volume = {In press},
journal = {Global Environmental Change},
author = {Fricko, Oliver and Havlik, Petr and Rogelj, Joeri and Klimont, Zbigniew and Gusti, Mykola and Johnson, Nils and Kolp, Peter and Strubegger, Manfred and Valin, Hugo and Amann, Markus and Ermolieva, Tatiana and Forsell, Nicklas and Herrero, Mario and Heyes, Chris and Kindermann, Georg and Krey, Volker and McCollum, David L. and Obersteiner, Michael and Pachauri, Shonali and Rao, Shilpa and Schmid, Erwin and Schoepp, Wolfgang and Riahi, Keywan},
year = {2016}
}
}}
{{#scite:
|bibtex=
@book{MSG-GLB_world_bank_group_world_2012,
title = {World {Development} {Indicators} 2012},
isbn = {0-8213-8985-8},
publisher = {World Bank Publications},
author = Template:World Bank Group,
year = {2012}
}
}}
{{#scite:
|bibtex=
@techreport{MSG-GLB_un_population_division_world_2010,
title = {World Population Projection},
author = {UN Population Division},
institution = {UN},
year = {2010}
}
}}
{{#scite:
|bibtex=
@techreport{MSG-GLB_international_energy_agency_energy_2012,
title = {Energy Balances},
author = {International Energy Agency},
institution = {International Energy Agency},
year = {2012}
}
}}
{{#scite:
|bibtex=
@techreport{MSG-GLB_international_energy_agency_world_2014,
title = {World {Energy} {Outlook} 2014},
url = {http://www.worldenergyoutlook.org/weo2014/},
author = {International Energy Agency},
institution = {International Energy Agency},
year = {2014}
}
}}
{{#scite:
|bibtex=
@techreport{MSG-GLB_oecd_uranium_2003,
author = {{OECD} and {NEA}}, title = {Uranium 2003: Resources, Production and Demand}, institution = Template:OECD/NEA, number = {NEA-05291}, month = {June}, year = {2003}, type = {Report}
} }}
{{#scite:
|bibtex=
@article{MSG-GLB_leibowicz_growth_2015,
author = {Benjamin D. Leibowicz}, title = {Growth and competition in renewable energy industries: Insights from an integrated assessment model with strategic firms}, journal = {Energy Economics}, volume = {52, Part A}, pages = {13 - 25}, year = {2015}, issn = {0140-9883}, doi = {http://dx.doi.org/10.1016/j.eneco.2015.09.010}
} }}
{{#scite:
|bibtex=
@techreport{MSG-GLB_alexandratos_world_2012,
author = {Alexandratos, Nikos and Bruinsma, Jelle}, title = {World agriculture towards 2030/2050: the 2012 revision}, institution = {FAO}, number = {12-03}, type = {ESA Working Paper}, month = {June}, year = {2012}, type = {Report}
} }}
{{#scite: |bibtex= @article{MSG-GLB_bouwman_exploring_2005,
author = {Bouwman, A.F. and der Hoek, K.W. Van and Eickhout, B. and Soenario, I.}, title = {Exploring changes in world ruminant production systems}, journal = {Agricultural Systems}, volume = {84}, number = {2}, pages = {121 - 153}, keywords = {Livestock production}, issn = {0308-521X}, url = {http://www.sciencedirect.com/science/article/B6T3W-4D1R2W8-1/2/03e2156d5e708f1dd8a94eded7badddc}, year = {2005}, type = {Journal Article}
} }}
{{#scite: |bibtex= @article{MSG-GLB_conant_grassland_2004,
author = {Conant, Richard T. and Paustian, Keith}, title = {Grassland Management Activity Data: Current Sources and Future Needs}, journal = {Environmental Management}, volume = {33}, number = {4}, pages = {467-473}, keywords = {Soil carbon sequestration Grasslands Management activity data}, issn = {0364-152X}, url = {http://dx.doi.org/10.1007/s00267-003-9104-7}, year = {2004}, type = {Journal Article}
} }}
{{#scite: |bibtex= @techreport{MSG-GLB_environmental_protection_agency_epa_US_2012,
author = {EPA}, title = {US Environmental Protection Agency Global Emissions Database}, institution = {US Environmental Protection Agency}, url = {http://www.epa.gov/climatechange/ghgemissions/global.html}, year = {2012}, type = {Report}
} }}
{{#scite: |bibtex= @techreport{MSG-GLB_food_and_agricultural_organization_fao_global_2010,
author = {FAO}, title = {Global Forest Resources Assessment}, institution = {Food and Agriculture Organization of the United Nations}, url = {http://www.fao.org/forestry/fra/fra2010/en/}, year = {2010}, type = {Report}
} }}
{{#scite: |bibtex= @article{MSG-GLB_fritz_highlighting_2011,
author = {Fritz, Steffen and See, Linda and McCallum, Ian and Schill, Christian and Obersteiner, Michael and van der Velde, Marijn and Boettcher, Hannes and Havlík, Petr and Achard, Frédéric}, title = {Highlighting continued uncertainty in global land cover maps for the user community}, journal = {Environmental Research Letters}, volume = {6}, number = {4}, pages = {044005}, url = {http://stacks.iop.org/1748-9326/6/i=4/a=044005}, year = {2011}, type = {Journal Article}
} }}
{{#scite:
|bibtex=
@article{MSG-GLB_herrero_global_2013,
author = {Herrero, M. and Havlik, P. and Valin, H. and Rufino, M.C. and Notenbaert, A.M.O. and Thornton, P.K. and Blummel, M. and Weiss, F. and Obertsteiner, M.}, title = {Global livestock systems: biomass use, production, feed efficiencies and greenhouse gas emissions},
journal = {Proceedings of the National Academy of Sciences},
type = {Journal Article},
volume = {110}, issn = {0027-8424}, number = {52}, year = {2013}, pages = {20888--20893} } }}
{{#scite: |bibtex= @article{MSG-GLB_herrero_systems_2008,
author = {Herrero, M. and Thornton, P.K. and Kruska, R. and Reid, R.S.}, title = {Systems dynamics and the spatial distribution of methane emissions from African domestic ruminants to 2030}, journal = {Agriculture, Ecosystems \& Environment}, volume = {126}, number = {1-2}, pages = {122 - 137}, keywords = {Methane}, issn = {0167-8809}, url = {http://www.sciencedirect.com/science/article/pii/S0167880908000121}, year = {2008}, type = {Journal Article}
} }}
{{#scite: |bibtex= @article{MSG-GLB_keyzer_diet_2005,
author = {Keyzer, M.A. and Merbis, M.D. and Pavel, I.F.P.W. and van Wesenbeeck, C.F.A.}, title = {Diet shifts towards meat and the effects on cereal use: can we feed the animals in 2030?}, journal = {Ecological Economics}, volume = {55}, number = {2}, pages = {187-202}, keywords = {Cereal feed demand, Dietary change, Food consumption pattern, Land use, Meat demand}, issn = {0921-8009}, url = {http://www.sciencedirect.com/science/article/pii/S0921800904004100}, year = {2005}, type = {Journal Article}
} }}
{{#scite:
|bibtex=
@article{MSG-GLB_kindermann_global_2008,
author = {Kindermann, G. and Obersteiner, M. and Sohngen, B. and Sathaye, J. and Andrasko, K. and Rametsteiner, E. and Schlamadinger, B. and Wunder, S. and Beach, R.}, title = {Global cost estimates of reducing carbon emissions through avoided deforestation}, journal = {Proceedings of the National Academy of Sciences}, volume = {105}, number = {30}, pages = {10302}, year = {2008}, type = {Journal Article}
} }}
{{#scite: |bibtex= @article{MSG-GLB_mccarl_surplus_1980,
author = {McCarl, Bruce A. and Spreen, Thomas H.}, title = {Price Endogenous Mathematical Programming as a Tool for Sector Analysis}, journal = {American Journal of Agricultural Economics}, volume = {62}, number = {1}, pages = {87-102}, issn = {00029092}, url = {http://www.jstor.org/stable/1239475}, year = {1980}, type = {Journal Article}
} }}
{{#scite: |bibtex= @article{MSG-GLB_mitchell_improved_2005,
author = {Mitchell, Timothy D. and Jones, Philip D.}, title = {An improved method of constructing a database of monthly climate observations and associated high-resolution grids}, journal = {International Journal of Climatology}, volume = {25}, number = {6}, pages = {693-712}, keywords = {climate, observations, grids, homogeneity, temperature, precipitation, vapour, cloud}, issn = {1097-0088}, url = {http://dx.doi.org/10.1002/joc.1181}, year = {2005}, type = {Journal Article}
} }}
{{#scite: |bibtex= @techreport{MSG-GLB_muhammad_international_2011,
author = {Muhammad, A. and Seale, J. and Meade, B. and Regmi, A.}, title = {International Evidence on Food Consumption Patterns: An Update Using 2005 International Comparison Program Data}, institution = {USDA-ERS}, number = {1929}, type = {Technical Bulletin}, year = {2011}, type = {Report}
} }}
{{#scite: |bibtex= @techreport{MSG-GLB_oneill_meeting_2012,
author = {O’Neill, B.C. and Carter, T.R. and Ebi, K.L. and Edmonds, J. and Hallegatte, S. and Kemp-Benedict, E. and Kriegler, E. and Mearns, L. and Moss, R. and Riahi, K. and van Ruijven, B. and van Vuuren, D.}, title = {Meeting Report of the Workshop on The Nature and Use of New Socioeconomic Pathways for Climate Change Research}, institution = {NCAR}, month = {November 2-4, 2011}, url = {http://www.isp.ucar.edu/socio-economic-pathways}, year = {2012}, type = {Report}
} }}
{{#scite: |bibtex= @article{MSG-GLB_parton_analysis_1987,
author = {Parton, WJ and Schimel, DS and Ojima, DS and Cole, CV}, title = {Analysis of factors controlling soil organic matter levels in Great Plains grasslands}, journal = {Soil Science Society of America Journal}, volume = {51}, number = {5}, pages = {1173-1179}, year = {1987}, type = {Journal Article}
} }}
{{#scite: |bibtex= @article{MSG-GLB_parton_observations_1993,
author = {Parton, W. J. and Scurlock, J. M. O. and Ojima, D. S. and Gilmanov, T. G. and Scholes, R. J. and Schimel, D. S. and Kirchner, T. and Menaut, J. C. and Seastedt, T. and Moya, E. G. and Kamnalrut, A. and Kinyamario, J. I.}, title = {Observations and modeling of biomass and soil organic-matter dynamics for the grassland biome worldwide}, journal = {Global Biogeochemical Cycles}, volume = {7}, pages = {785-809}, year = {1993}, type = {Journal Article}
} }}
{{#scite: |bibtex= @article{MSG-GLB_ramankutty_farming_2008,
author = {Ramankutty, N. and Evan, A.T. and Monfreda, C. and Foley, J.A.}, title = {Farming the planet: 1. Geographic distribution of global agricultural lands in the year 2000}, journal = {Global Biogeochemical Cycles}, volume = {22}, number = {1}, pages = {1-19}, issn = {0886-6236}, year = {2008}, type = {Journal Article}
} }}
{{#scite: |bibtex= @article{MSG-GLB_reynolds_estimating_2000,
author = {Reynolds, CA and Jackson, TJ and Rawls, WJ}, title = {Estimating soil water-holding capacities by linking the Food and Agriculture Organization soil map of the world with global pedon databases and continuous pedotransfer functions}, journal = {Water Resources Research}, volume = {36}, number = {12}, pages = {3653-3662}, year = {2000}, type = {Journal Article}
} }}
{{#scite: |bibtex= @techreport{MSG-GLB_ruesch_new_ipcc_2008,
author = {Ruesch, Aaron and Gibbs, Holly K.}, title = {New IPCC Tier-1 Global Biomass Carbon Map For the Year 2000}, institution = {Oak Ridge National Laboratory}, type = {Available online from the Carbon Dioxide Information Analysis Center}, url = {http://cdiac.ornl.gov/epubs/ndp/global_carbon/carbon_documentation.html}, year = {2008}, type = {Report}
} }}
{{#scite: |bibtex= @article{MSG-GLB_russ_global_2007,
author = {Russ, P. and Wiesenthal, T. and van Regemorter, D. and Ciscar, J.C.}, title = {Global Climate Policy Scenarios for 2030 and beyond: Analysis of Greenhouse Gas Emission Reduction Pathway Scenarios with the POLES and GEME3 Models}, journal = {Institute for Prospective technological Studies, October}, year = {2007}, type = {Journal Article}
} }}
{{#scite: |bibtex= @article{MSG-GLB_schloss_comparing_1999,
author = {Schloss, A. L. and Kicklighter, D. W. and Kaduk, J. and Wittenberg, U. and (The Participants of the Potsdam NPP Model Comparison)}, title = {Comparing global models of terrestrial net primary productivity (NPP): comparison of NPP to climate and the Normalized Difference Vegetation Index (NDVI)}, journal = {Global Change Biology}, volume = {5}, number = {S1}, pages = {25-34}, keywords = {NPP, global, model, climate, NDVI, seasonal}, issn = {1365-2486}, url = {http://dx.doi.org/10.1046/j.1365-2486.1999.00004.x}, year = {1999}, type = {Journal Article}
} }}
{{#scite: |bibtex= @article{MSG-GLB_schneider_agricultural_2007,
author = {Schneider, Uwe A. and McCarl, Bruce A. and Schmid, Erwin}, title = {Agricultural sector analysis on greenhouse gas mitigation in US agriculture and forestry}, journal = {Agricultural Systems}, volume = {94}, number = {2}, pages = {128 - 140}, keywords = {Abatement function}, issn = {0308-521X}, url = {http://www.sciencedirect.com/science/article/pii/S0308521X06001028}, year = {2007}, type = {Journal Article}
} }}
{{#scite: |bibtex= @techreport{MSG-GLB_seale_international_2003,
author = {Seale, James and Regmi, Anita and Bernstein, Jason}, title = {International Evidence on Food Consumption Patterns}, institution = {USDA-ERS}, number = {1904}, type = {Technical Bulletin}, month = {October}, url = {http://www.ers.usda.gov/Data/InternationalFoodDemand/}, year = {2003}, type = {Report}
} }}
{{#scite: |bibtex= @techreport{MSG-GLB_sere_world_1996,
author = {Seré, C. and Steinfeld, H.}, title = {World livestock production systems: current status, issues and trends}, institution = {Food and Agriculture Organisation}, number = {127}, type = {Animal and Health Paper}, url = {http://www.fao.org/WAIRDOCS/LEAD/X6101E/X6101E00.HTM}, year = {1996}, type = {Report}
} }}
{{#scite: |bibtex= @techreport{MSG-GLB_skalsky_geo-bene_2008,
author = {Skalsky, R. and Tarasovicova, Z. and Balkovic, J. and Schmid, E. and Fuchs, M. and Moltchanova, E. and Kindermann, G. and Scholtz, P.}, title = {Geo-bene global database for bio-physical modeling v.1.0. Concepts, methodologies and data.Technical Report}, institution = {IIASA}, month = {accessed 13.03.09}, url = {http://www.geo-bene.eu/?q=node/1734S}, year = {2008}, type = {Report}
} }}
{{#scite: |bibtex= @book{MSG-GLB_takayama_spatial_1971,
author = {Takayama, T. and Judge, G.G.}, title = {Spatial and temporal price and allocation models}, publisher = {North-Holland Amsterdam}, year = {1971}, type = {Book}
} }}
{{#scite: |bibtex= @article{MSG-GLB_tubiello_faostat_2013,
author = {Tubiello, Francesco N and Salvatore, Mirella and Rossi, Simone and Ferrara, Alessandro and Fitton, Nuala and Smith, Pete}, title = {The FAOSTAT database of greenhouse gas emissions from agriculture}, journal = {Environmental Research Letters}, volume = {8}, number = {1}, pages = {015009}, url = {http://stacks.iop.org/1748-9326/8/i=1/a=015009}, year = {2013}, type = {Journal Article}
} }}
{{#scite: |bibtex= @article{MSG-GLB_williams_computer_1995,
author = {Williams, J.R. and Singh, VP}, title = {The EPIC model}, journal = {Computer models of watershed hydrology}, pages = {909-1000}, year = {1995}, type = {Journal Article}
} }}
{{#scite: |bibtex= @book{MSG-GLB_wint_gridded_2007,
author = {Wint, W. and Robinson, T.}, title = {Gridded livestock of the world 2007}, publisher = {FAO}, year = {2007}, type = {Book}
} }}
{{#scite: |bibtex= @article{MSG-GLB_you_entropy_2006,
author = {You, Liangzhi and Wood, Stanley}, title = {An entropy approach to spatial disaggregation of agricultural production}, journal = {Agricultural Systems}, volume = {90}, number = {1-3}, pages = {329 - 347}, issn = {0308-521X}, url = {http://www.sciencedirect.com/science/article/B6T3W-4JKYWM1-1/2/381253576eb09660fc9860c6c8bb8e1f}, year = {2006}, type = {Journal Article}
} }}
{{#scite: |bibtex= @article{MSG-GLB_izaurralde_simulating_2006,
author = {Izaurralde, R. C. and Williams, J. R. and McGill, W. B. and Rosenberg, N. J. and Jakas, M. C. Q.}, title = {Simulating soil C dynamics with EPIC: Model description and testing against long-term data}, journal = {Ecological Modelling}, volume = {192}, number = {3-4}, pages = {362-384}, keywords = {Climate change
Soil C model Soil carbon sequestration Tillage Water erosion Wind erosion},
url = {http://www.scopus.com/scopus/inward/record.url?eid=2-s2.0-31944437556&partnerID=40&rel=R8.2.0 }, year = {2006}, type = {Journal Article}
} }}
{{#scite: |bibtex= @report{sauer_agriculture_2008,
author = {Sauer, T. and Havlík, P. and Kindermann, G. and Schneider, U.A. . }, title = {Agriculture, Population, Land and Water Scarcity in a changing World - the Role of Irrigation}, institution = {Congress of the European Association of Agricultural Economists}, year = {2008}, type = {Report}
} }}
{{#scite: |bibtex= @techreport{MSG-GLB_FAO_global_2006,
author = {FAO}, title = {Global Forest Resources Assessment 2005. Progress towards sustainable forest management.}, institution = {Food and Agriculture Organization of the United Nations}, year = {2006}, type = {Report}
} }}
{{#scite: |bibtex= @article{MSG-GLB_kindermann_global_forest_2008,
author = {Kindermann, G. E. and McCallum, I. and Fritz, S. and Obersteiner, M.}, title = {A global forest growing stock, biomass and carbon map based on FAO statistics}, journal = {Silva Fennica}, volume = {42}, number = {3}, pages = {387-396}, keywords = {Biomass map
Downscaling Regression analysis},
url = {http://www.scopus.com/scopus/inward/record.url?eid=2-s2.0-46249088682&partnerID=40&rel=R8.2.0 }, year = {2008}, type = {Journal Article}
} }}
{{#scite: |bibtex= @book{MSG-GLB_biomass_handbook_2005,
author = {Biomass Technology Group}, title = {Handbook Biomass Gasification}, publisher = {H.A.M. Knoef. ISBN: 90-810068-1-9}, year = {2005}, type = {Book}
} }}
{{#scite: |bibtex= @techreport{MSG-GLB_rametsteiner_study_2007,
author = {Rametsteiner, E and Nilsson, S and Böttcher, H and Havlik, P and Kraxner, F and Leduc, S and Obersteiner, M and Rydzak, F and Schneider, U and Schwab, D and Willmore, L}, title = {Study of the Effects of Globalization on the Economic Viability of EU Forestry. Final Report of the AGRI Tender Project: AGRI-G4-2006-06 [2007]. EC Contract Number 30-CE-0097579/00-89}, institution = {EC/IIASA}, url = {http://ec.europa.eu/agriculture/analysis/external/viability_forestry/index_en.htm}, year = {2007}, type = {Report}
} }}
{{#scite: |bibtex= @techreport{MSG-GLB_hamelinck_future_2001,
author = {Hamelinck, C.N. and Faaij, A.P.C.}, title = {Future Prospects for Production of Methanol and Hydrogen from Biomass}, institution = {Utrecht University, Copernicus Institute, Science Technology and Society}, year = {2001}, type = {Report}
} }}
{{#scite: |bibtex= @article{MSG-GLB_leduc_optimal_2008,
author = {Leduc, S. and Schwab, D. and Dotzauer, E. and Schmid, E. and Obersteiner, M.}, title = {Optimal location of wood gasification plants for methanol production with heat recovery}, journal = {International Journal of Energy Research}, volume = {32}, pages = {1080--1091 [2008]}, year = {2008}, type = {Journal Article}
} }}
{{#scite: |bibtex= @techreport{MSG-GLB_sørensen_economies_2005,
author = {Sørensen, A. L.}, title = {Economies of Scale in Biomass Gasification Systems}, institution = {IIASA }, number = {Interim Report IR-05-030}, year = {2005}, type = {Report}
} }}
{{#scite: |bibtex= @article{MSG-GLB_carpentieri_future_1993,
author = {Carpentieri, A. E. and Larson, E. D. and Woods, J.}, title = {Future biomass-based electricity supply in Northeast Brazil}, journal = {Biomass and Bioenergy}, volume = {4}, number = {3}, pages = {149-173}, keywords = {bagasse
Biomass electricity Brazil gas turbine gasifier plantations},
url = {http://www.scopus.com/scopus/inward/record.url?eid=2-s2.0-0027382662&partnerID=40&rel=R8.2.0 }, year = {1993}, type = {Journal Article}
} }}
{{#scite: |bibtex= @report{MSG-GLB_herzogbaum_forstpflanzen_2008,
author = {Herzogbaum, GmbH}, title = {Forstpflanzen-Preisliste 2008. HERZOG.BAUM Samen & Pflanzen GmbH. Koaserbauerstr. 10, A - 4810 Gmunden. Austria (also available at www.energiehoelzer.at)}, institution = {Herzogbaum GmbH}, year = {2008}, type = {report}
} }}
{{#scite: |bibtex= @techreport{MSG-GLB_jurvélius_labor_1997,
author = {Jurvélius, Mike}, title = {Labor-intensive harvesting of tree plantations in the southern Philippines. Forest harvesting case -study 9. RAP Publication: 1997/41}, institution = {Food and Agriculture Organization of the United Nations}, year = {1997}, type = {Report}
} }}
{{#scite: |bibtex= @techreport{MSG-GLB_ILO_occupational_2007,
author = {ILO}, title = {Occupational Wages and Hours of Work and Retail Food Prices, Statistics from the ILO October Inquiry}, institution = {International Labor Organisation}, year = {2007}, type = {Report}
} }}
{{#scite: |bibtex= @techreport{MSG-GLB_FPP_holzernte_1999,
author = {FPP}, title = {Holzernte in der Durchforstung; Leistungszahlen Kosten - OeBF Seiltabelle Sortimentverfahren (SKM-TAB)}, institution = {Kooperationsabkommen Forst-Platte-Papier}, year = {1999}, type = {Report}
} }}
{{#scite: |bibtex= @article{MSG-GLB_jiroušek_productivity_2007,
author = {Jiroušek, R. and Klvač, R. and Skoupý, A.}, title = {Productivity and costs of the mechanised cut-to-length wood harvesting system in clear-felling operations}, journal = {Journal of Forest Science}, volume = {53}, number = {10}, pages = {476-482}, keywords = {Average tree volume
Harvester Hauling distance Payload},
url = {http://www.scopus.com/scopus/inward/record.url?eid=2-s2.0-35448931938&partnerID=40&rel=R8.2.0 }, year = {2007}, type = {Journal Article}
} }}
{{#scite: |bibtex= @article{MSG-GLB_stokes_field_1986,
author = {Stokes, B. J. and Frederick, D. J. and Curtin, D. T.}, title = {Field trials of a short-rotation biomass feller buncher and selected harvesting systems}, journal = {Biomass}, volume = {11}, number = {3}, pages = {185-204}, keywords = {Biomass
harvesting production yield},
url = {http://www.scopus.com/scopus/inward/record.url?eid=2-s2.0-0022984004&partnerID=40&rel=R8.2.0 }, year = {1986}, type = {Journal Article}
} }}
{{#scite: |bibtex= @article{MSG-GLB_wang_productivity_2004,
author = {Wang, J. and Long, C. and McNeel, J. and Baumgras, J.}, title = {Productivity and cost of manual felling and cable skidding in central Appalachian hardwood forests}, journal = {Forest Products Journal}, volume = {54}, number = {12}, pages = {45-51}, url = {http://www.scopus.com/scopus/inward/record.url?eid=2-s2.0-11844274724&partnerID=40&rel=R8.2.0 }, year = {2004}, type = {Journal Article}
} }}
{{#scite: |bibtex= @article{MSG-GLB_hartsough_harvesting_2001,
author = {Hartsough, B. R. and Zhang, X. and Fight, R. D.}, title = {Harvesting cost model for small trees in natural stands in the Interior Northwest}, journal = {Forest Products Journal}, volume = {51}, number = {4}, pages = {54-61}, url = {http://www.scopus.com/scopus/inward/record.url?eid=2-s2.0-0035306334&partnerID=40&rel=R8.2.0 }, year = {2001}, type = {Journal Article}
} }}
{{#scite: |bibtex= @techreport{MSG-GLB_heston_penn_2006,
author = {Heston, A. and Summers, R. and Aten, B.}, title = {Penn World Table Version 6.2}, institution = {Center for International Comparisons of Production, Income and Prices at the University of Pennsylvania. September 2006. http://pwt.econ.upenn.edu/php_site/pwt62/pwt62_form.php}, year = {2006}, type = {Report}
} }}
- ↑ Daniel Huppmann, Matthew Gidden, Oliver Fricko, Peter Kolp, Clara Orthofer, Michael Pimmer, Nikolay Kushin, Adriano Vinca, Alessio Mastrucci, Keywan Riahi, and Volker Krey. The messageix integrated assessment model and the ix modeling platform (ixmp): an open framework for integrated and cross-cutting analysis of energy, climate, the environment, and sustainable development. Environmental Modelling & Software, 112:143–156, 2019. doi:10.1016/j.envsoft.2018.11.012.
- ↑ S. Rao, Z. Klimont, S.J. Smith, R. Van Dingenen, F. Dentener, L. Bouwman, K. Riahi, M. Amann, B.L. Bodirsky, D.P. van Vuuren, L. Aleluia Reis, K. Calvin, L. Drouet, O. Fricko, S. Fujimori, D. Gernaat, P. Havlik, M. Harmsen, T. Hasegawa, C. Heyes, J. Hilaire, G. Luderer, T. Masui, E. Stehfest, J. Strefler, S. van der Sluis, and M. Tavoni. Future air pollution in the shared socio-economic pathways. Global Environmental Change, 42:346–358, 2017. doi:10.1016/j.gloenvcha.2016.05.012.
- ↑ D.L. McCollum, W. Zhou, C. Bertram, H.-S. De Boer, V. Bosetti, S. Busch, J. Després, L. Drouet, J. Emmerling, M. Fay, O. Fricko, S. Fujimori, M. Gidden, M. Harmsen, D. Huppmann, G. Iyer, V. Krey, E. Kriegler, C. Nicolas, S. Pachauri, S. Parkinson, M. Poblete-Cazenave, P. Rafaj, N. Rao, J. Rozenberg, A. Schmitz, W. Schoepp, D. Van Vuuren, and K. Riahi. Energy investment needs for fulfilling the paris agreement and achieving the sustainable development goals. Nature Energy, 3(7):589–599, 2018. doi:10.1038/s41560-018-0179-z.
- ↑ A. Grubler, C. Wilson, N. Bento, B. Boza-Kiss, V. Krey, D.L. McCollum, N.D. Rao, K. Riahi, J. Rogelj, S. De Stercke, J. Cullen, S. Frank, O. Fricko, F. Guo, M. Gidden, P. Havlík, D. Huppmann, G. Kiesewetter, P. Rafaj, W. Schoepp, and H. Valin. A low energy demand scenario for meeting the 1.5 °c target and sustainable development goals without negative emission technologies. Nature Energy, 3(6):515–527, 2018. doi:10.1038/s41560-018-0172-6.
- ↑ Keywan Riahi, Detlef P. van Vuuren, Elmar Kriegler, Jae Edmonds, Brian O’Neill, Shinichiro Fujimori, Nico Bauer, Katherine Calvin, Rob Dellink, Oliver Fricko, Wolfgang Lutz, Alexander Popp, Jesus Crespo Cuaresma, Samir KC, Marian Leimbach, Leiwen Jiang, Tom Kram, Shilpa Rao, Johannes Emmerling, Kristie Ebi, Tomoko Hasegawa, Petr Havlik, Florian Humpenoder, Lara Aleluia Da Silva, Steve Smith, Elke Stehfest, Valentina Bosetti, Jiyong Eom, David Gernaat, Toshihiko Masui, Joeri Rogelj, Jessica Strefler, Laurent Drouet, Volker Krey, Gunnar Luderer, Mathijs Harmsen, Kiyoshi Takahashi, Lavinia Baumstark, Jonathan Doelman, Mikiko Kainuma, Zbigniew Klimont, Giacomo Marangoni, Hermann Lotze-Campen, Michael Obersteiner, Andrzej Tabeau, and Massimo Tavoni. The Shared Socioeconomic Pathways and their Energy, Land Use, and Greenhouse Gas Emissions Implications. Global Environmental Change, 42:153–168, 2017. URL: http://pure.iiasa.ac.at/13280/, doi:10.1016/j.gloenvcha.2016.05.009.
- ↑ Daniel Huppmann, Matthew Gidden, Oliver Fricko, Peter Kolp, Clara Orthofer, Michael Pimmer, Nikolay Kushin, Adriano Vinca, Alessio Mastrucci, Keywan Riahi, and Volker Krey. The messageix integrated assessment model and the ix modeling platform (ixmp): an open framework for integrated and cross-cutting analysis of energy, climate, the environment, and sustainable development. Environmental Modelling & Software, 112:143–156, 2019. doi:10.1016/j.envsoft.2018.11.012.
- ↑ 7.0 7.1 7.2 7.3 Brian C O’Neill, Elmar Kriegler, Kristie L Ebi, Eric Kemp-Benedict, Keywan Riahi, Dale S Rothman, Bas J van Ruijven, Detlef P van Vuuren, Joern Birkmann, and Kasper Kok. The roads ahead: narratives for shared socioeconomic pathways describing world futures in the 21st century. Global Environmental Change, 2015.
- ↑ Keywan Riahi, Detlef P. van Vuuren, Elmar Kriegler, Jae Edmonds, Brian O’Neill, Shinichiro Fujimori, Nico Bauer, Katherine Calvin, Rob Dellink, Oliver Fricko, Wolfgang Lutz, Alexander Popp, Jesus Crespo Cuaresma, Samir KC, Marian Leimbach, Leiwen Jiang, Tom Kram, Shilpa Rao, Johannes Emmerling, Kristie Ebi, Tomoko Hasegawa, Petr Havlik, Florian Humpenoder, Lara Aleluia Da Silva, Steve Smith, Elke Stehfest, Valentina Bosetti, Jiyong Eom, David Gernaat, Toshihiko Masui, Joeri Rogelj, Jessica Strefler, Laurent Drouet, Volker Krey, Gunnar Luderer, Mathijs Harmsen, Kiyoshi Takahashi, Lavinia Baumstark, Jonathan Doelman, Mikiko Kainuma, Zbigniew Klimont, Giacomo Marangoni, Hermann Lotze-Campen, Michael Obersteiner, Andrzej Tabeau, and Massimo Tavoni. The Shared Socioeconomic Pathways and their Energy, Land Use, and Greenhouse Gas Emissions Implications. Global Environmental Change, 42:153–168, 2017. URL: http://pure.iiasa.ac.at/13280/, doi:10.1016/j.gloenvcha.2016.05.009.
- ↑ Oliver Fricko, Petr Havlik, Joeri Rogelj, Zbigniew Klimont, Mykola Gusti, Nils Johnson, Peter Kolp, Manfred Strubegger, Hugo Valin, Markus Amann, Tatiana Ermolieva, Nicklas Forsell, Mario Herrero, Chris Heyes, Georg Kindermann, Volker Krey, David L. McCollum, Michael Obersteiner, Shonali Pachauri, Shilpa Rao, Erwin Schmid, Wolfgang Schoepp, and Keywan Riahi. The marker quantification of the shared socioeconomic pathway 2: a middle-of-the-road scenario for the 21st century. Global Environmental Change, 42:251–267, 2017.
- ↑ Daniel Huppmann, Matthew Gidden, Oliver Fricko, Peter Kolp, Clara Orthofer, Michael Pimmer, Nikolay Kushin, Adriano Vinca, Alessio Mastrucci, Keywan Riahi, and Volker Krey. The messageix integrated assessment model and the ix modeling platform (ixmp): an open framework for integrated and cross-cutting analysis of energy, climate, the environment, and sustainable development. Environmental Modelling & Software, 112:143–156, 2019. doi:10.1016/j.envsoft.2018.11.012.
- ↑ Sabine Messner and Manfred Strubegger. User’s Guide for MESSAGE III. 1995. URL: http://pure.iiasa.ac.at/id/eprint/4527/1/WP-95-069.pdf.
Note: From 1990 to 2010 MESSAGE employs 5-year time steps with 10-year steps employed thereafter